Learning Optimal Forecast Aggregation in Partial Evidence Environments
Mathematics of Operations Research(2021)
摘要
We consider the forecast aggregation problem in repeated settings where the forecasts are of a binary state of nature. In each period multiple experts provide forecasts about the state. The goal of the aggregator is to aggregate those forecasts into a subjective accurate forecast. We assume that the experts are Bayesian and the aggregator is non-Bayesian and ignorant of the information structure (i.e., the distribution over the signals) under which the experts make their forecasts. The aggregator observes the experts' forecasts only. At the end of each period, the realized state is observed by the aggregator. We focus on the question of whether the aggregator can learn to optimally aggregate the forecasts of the experts, where the optimal aggregation is the Bayesian aggregation that takes into account all the information in the system. We consider the class of partial evidence information structures, where each expert is exposed to a different subset of conditionally independent signals. Our main results are positive: we show that optimal aggregation can be learned in polynomial time in quite a wide range of instances in partial evidence environments. We provide an exact characterization of the instances where optimal learning is possible as well as those where it is impossible.
更多查看译文
关键词
information aggregation,Bayesian experts,online learning
AI 理解论文
溯源树
样例
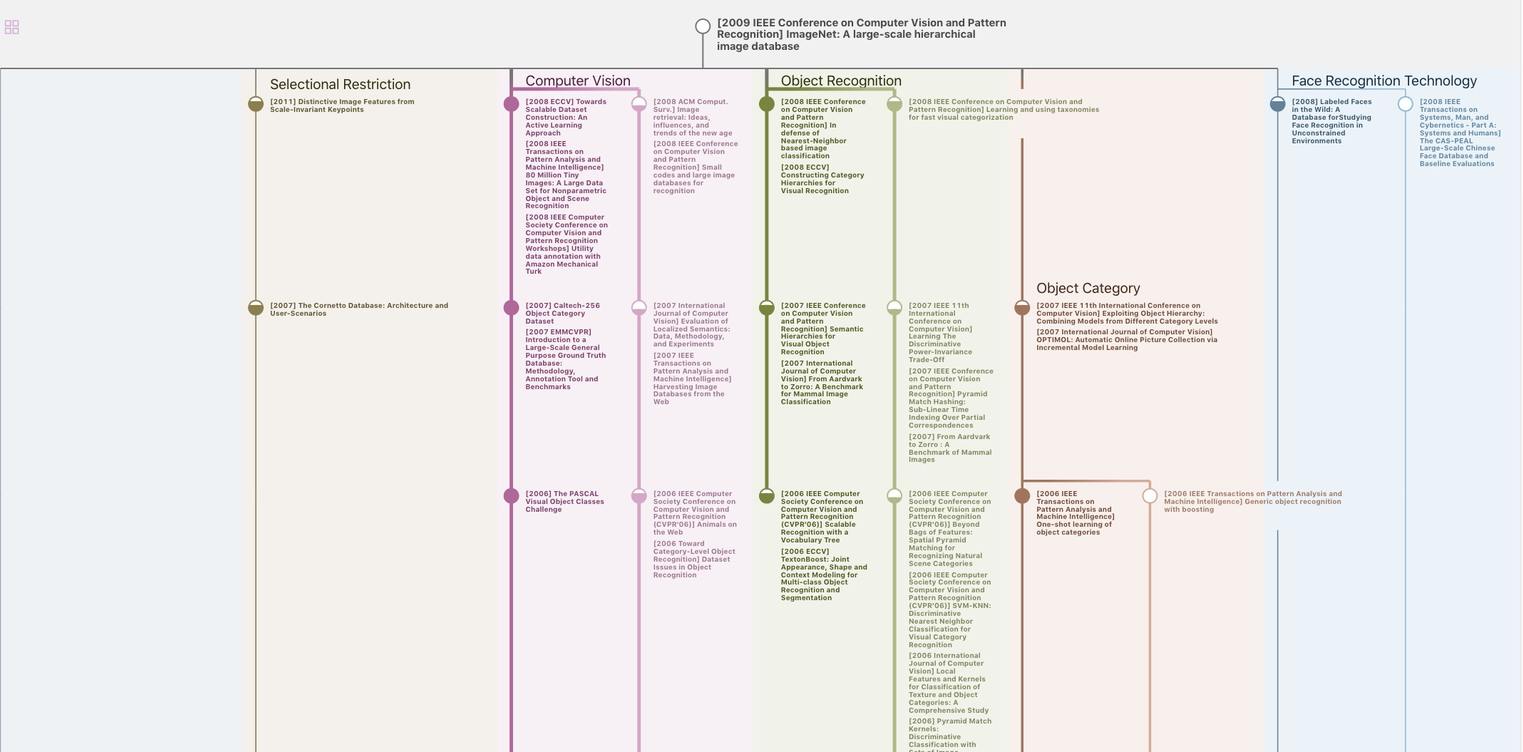
生成溯源树,研究论文发展脉络
Chat Paper
正在生成论文摘要