Convolutional Geometric Matrix Completion.
arXiv: Learning(2018)
摘要
Geometric matrix completion~(GMC) has been proposed for recommendation by integrating the relationship~(link) graphs among users/items into matrix completion~(MC) . Traditional mbox{GMC} methods typically adopt graph regularization to impose smoothness priors for mbox{MC}. Recently, geometric deep learning on graphs~(mbox{GDLG}) is proposed to solve the GMC problem, showing better performance than existing GMC methods including traditional graph regularization based methods. To the best of our knowledge, there exists only one GDLG method for GMC, which is called mbox{RMGCNN}. RMGCNN combines graph convolutional network~(GCN) and recurrent neural network~(RNN) together for GMC. In the original work of RMGCNN, RMGCNN demonstrates better performance than pure GCN-based method. In this paper, we propose a new mbox{GMC} method, called underline{c}onvolutional underline{g}eometric underline{m}atrix underline{c}ompletion~(CGMC), for recommendation with graphs among users/items. CGMC is a pure GCN-based method with a newly designed graph convolutional network. Experimental results on real datasets show that CGMC can outperform other state-of-the-art methods including RMGCNN.
更多查看译文
AI 理解论文
溯源树
样例
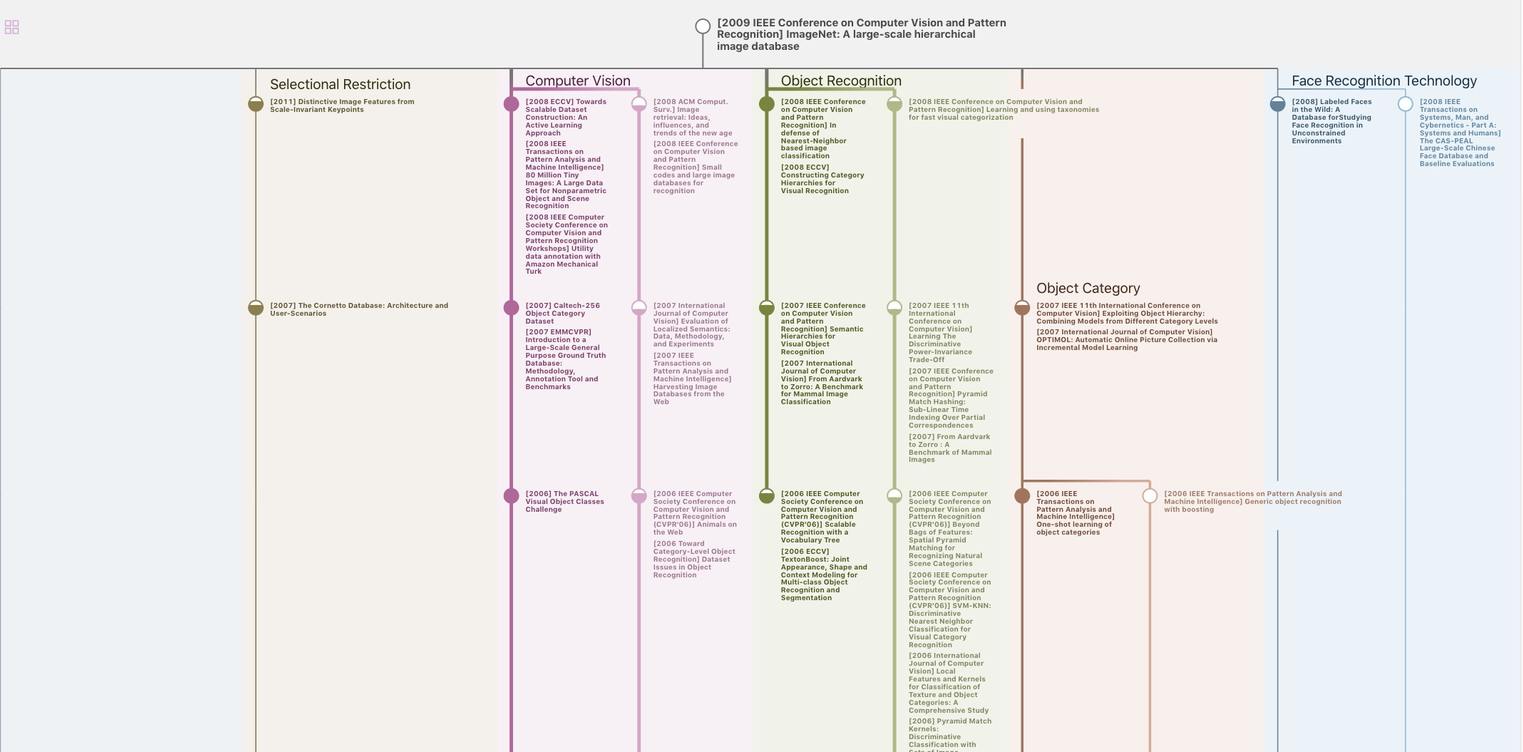
生成溯源树,研究论文发展脉络
Chat Paper
正在生成论文摘要