SybilFuse: Combining Local Attributes with Global Structure to Perform Robust Sybil Detection.
IEEE Conference on Communications and Network Security(2018)
摘要
Sybil attacks are becoming increasingly widespread and pose a significant threat to online social systems; a single adversary can inject multiple colluding identities in the system to compromise security and privacy. Recent works have leveraged social network-based trust relationships to defend against Sybil attacks. However, existing defenses are based on oversimplified assumptions about network structure, which do not necessarily hold in real-world social networks. Recognizing these limitations, we propose SYBILFUSE, a defense-in-depth framework for Sybil detection when the oversimplified assumptions are relaxed. SYBILFUSE adopts a collective classification approach by first training local classifiers to compute local trust scores for nodes and edges, and then propagating the local scores through the global network structure via weighted random walk and loopy belief propagation mechanisms. We evaluate our framework on both synthetic and real-world network topologies, including a large-scale, labeled Twitter network comprising 20M nodes and 265M edges, and demonstrate that SYBILFUSE outperforms state-of-the-art approaches significantly. In particular, SYBILFUSE achieves 98% of Sybil coverage among top-ranked nodes.
更多查看译文
关键词
global structure,robust sybil detection,Sybil attacks,social systems,security,privacy,real-world social networks,SYBILFUSE,defense-in-depth framework,collective classification approach,training local classifiers,local trust scores,local scores,global network structure,weighted random walk,loopy belief propagation mechanisms,real-world network topologies,Sybil coverage,local attributes,social network-based trust relationships
AI 理解论文
溯源树
样例
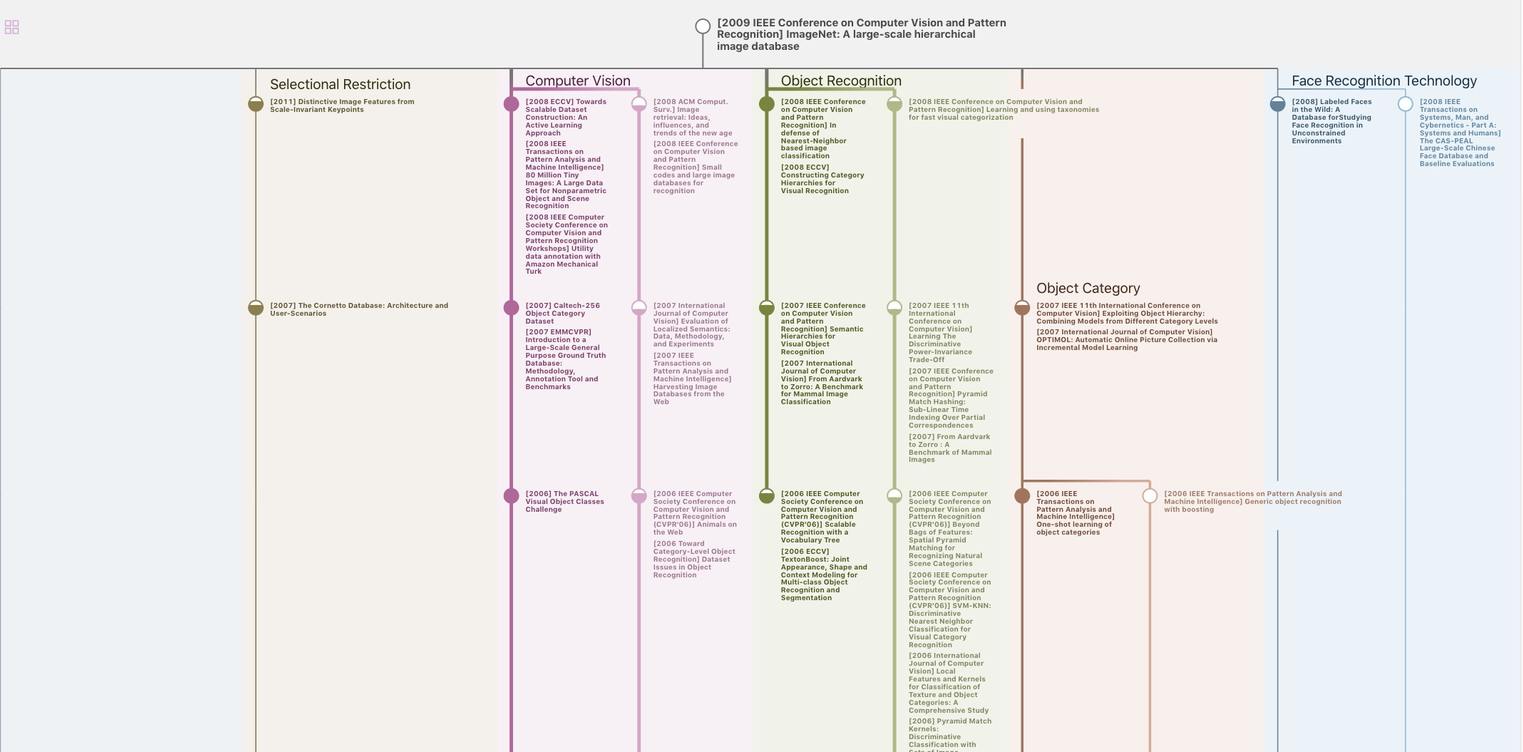
生成溯源树,研究论文发展脉络
Chat Paper
正在生成论文摘要