Correlation and redundancy on machine learning performance for chemical databases
JOURNAL OF CHEMOMETRICS(2018)
摘要
Variable reduction is an essential step for establishing a robust, accurate, and generalized machine learning model. Variable correlation and redundancy/total correlation are the primary considerations in many variable reduction methods given that they directly impact model performances. However, their effects vary from one class of databases to another. To clarify their effects on regression models on the basis of small chemical databases, a series of calculations are performed. Regression models are built on features with various correlation coefficients and redundancies by 4 machine learning methods: random forest, support vector machine, extreme learning machine, and multiple linear regression. The results suggest that the correlation is, as expected, closely related to the prediction accuracy; ie, generally, the features with large correlation coefficients regarding to response variables achieve better regression models than those with lower ones. However, for the redundancy, no trends on the performances of regression models are disclosed. This may indicate that for these chemical molecular databases, the redundancy might not be a primary concern. Feature correlation and redundancy, quasi-pairwise factors in machine learning modeling, are widely considered in variable reduction methods. Their effects on regression models are not uniform for databases in various areas. Therefore, they are investigated for 4 types of regression models, random forest, support vector machine, extreme learning machine, and multiple linear regression, based on small chemical databases with quantum chemical and structural molecular descriptors. The correlation is closely related to the prediction, and generally, higher correlation leads to better predictions; the redundancy effect is clueless, which means that the redundancy is not certain to deteriorate the regression model based on chemical databases. On the basis of regression and density functional theory calculations, an optimal setting for obtaining quantum chemical descriptors is suggested for similar database regression modeling.
更多查看译文
关键词
chemical databases,correlation,density functional theory (DFT),machine learning regression,redundancy,total correlation
AI 理解论文
溯源树
样例
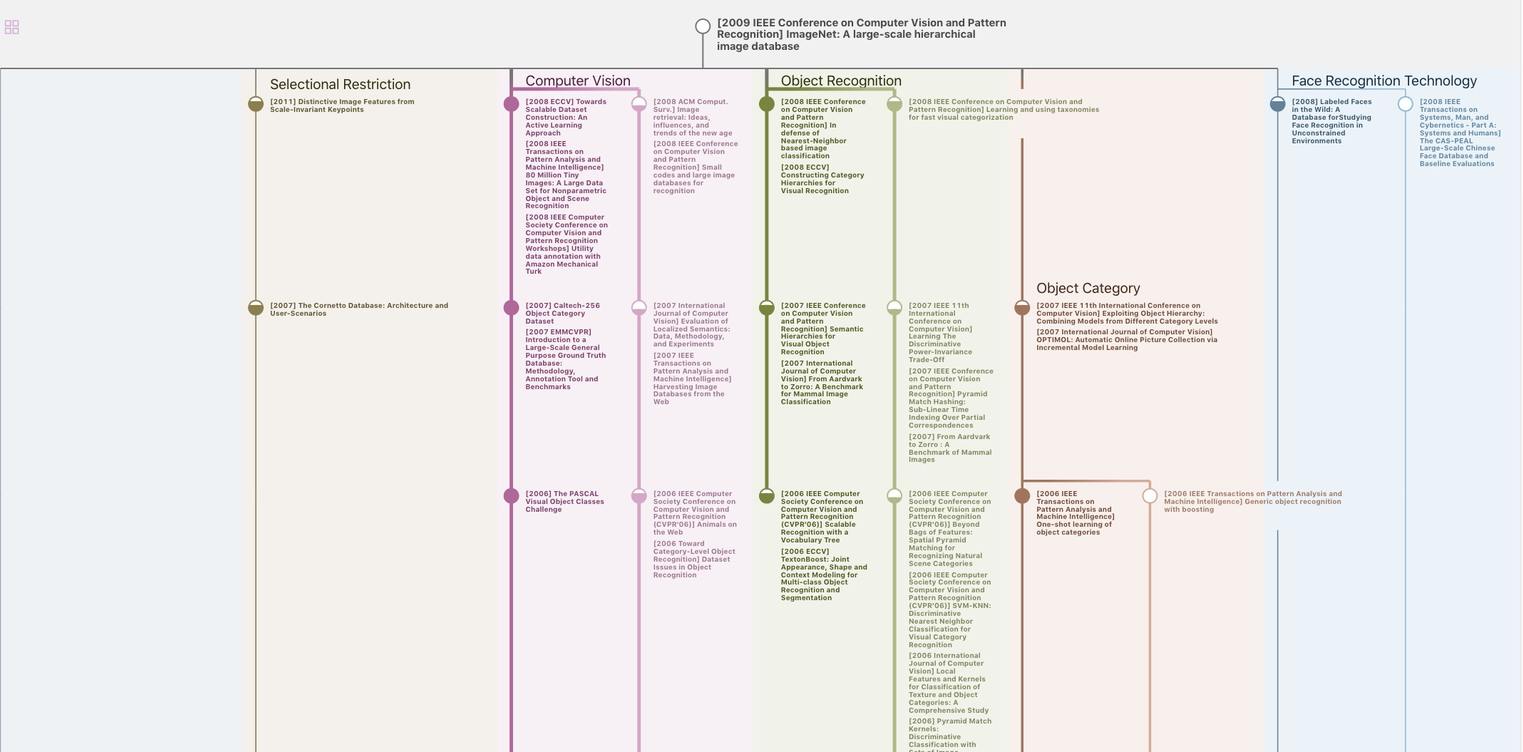
生成溯源树,研究论文发展脉络
Chat Paper
正在生成论文摘要