MATIC: Learning around errors for efficient low-voltage neural network accelerators
2018 Design, Automation & Test in Europe Conference & Exhibition (DATE)(2018)
摘要
As a result of the increasing demand for deep neural network (DNN)-based services, efforts to develop dedicated hardware accelerators for DNNs are growing rapidly. However, while accelerators with high performance and efficiency on convolutional deep neural networks (Conv-DNNs) have been developed, less progress has been made with regards to fully-connected DNNs (FC-DNNs). In this paper, we propose MATIC (Memory Adaptive Training with In-situ Canaries), a methodology that enables aggressive voltage scaling of accelerator weight memories to improve the energy-efficiency of DNN accelerators. To enable accurate operation with voltage overscaling, MATIC combines the characteristics of destructive SRAM reads with the error resilience of neural networks in a memory-adaptive training process. Furthermore, PVT-related voltage margins are eliminated using bit-cells from synaptic weights as in-situ canaries to track runtime environmental variation. Demonstrated on a low-power DNN accelerator that we fabricate in 65 nm CMOS, MATIC enables up to 60-80 mV of voltage overscaling (3.3× total energy reduction versus the nominal voltage), or 18.6× application error reduction.
更多查看译文
关键词
Deep neural networks,voltage scaling,SRAM,machine learning acceleration
AI 理解论文
溯源树
样例
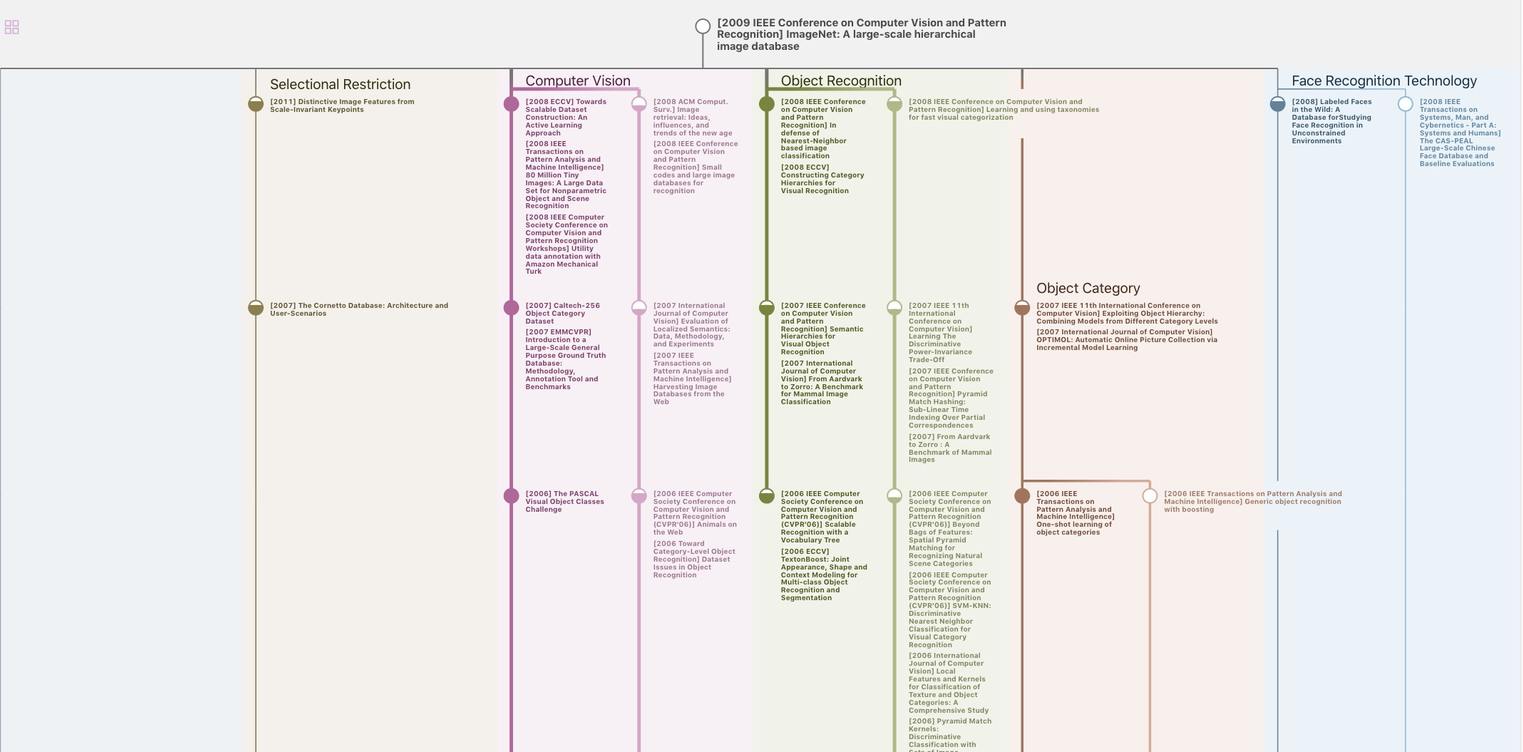
生成溯源树,研究论文发展脉络
Chat Paper
正在生成论文摘要