Learning Joint Gaussian Representations for Movies, Actors, and Literary Characters.
arXiv (Cornell University)(2018)
摘要
Understanding of narrative content has become an increasingly popular topic. However, narrative semantics pose difficult challenges as the effects of multiple narrative facets, such as the text, events, character types, and genres, are tightly intertwined. We present a joint representation learning framework for embedding actors, literary characters, movies, genres, and descriptive keywords as Gaussian distributions and translation vectors on the Gaussian means. The Gaussian variance naturally corresponds to actors' versatility, a central concept in acting. Our estimate of actors' versatility agree with domain experts' rankings 65.95% of the time. This is, to our knowledge, the first computational technique for estimating this semantic concept. Additionally, the model substantially outperforms a TransE baseline in prediction of actor casting choices.
更多查看译文
关键词
joint gaussian representations,literary characters,actors,movies
AI 理解论文
溯源树
样例
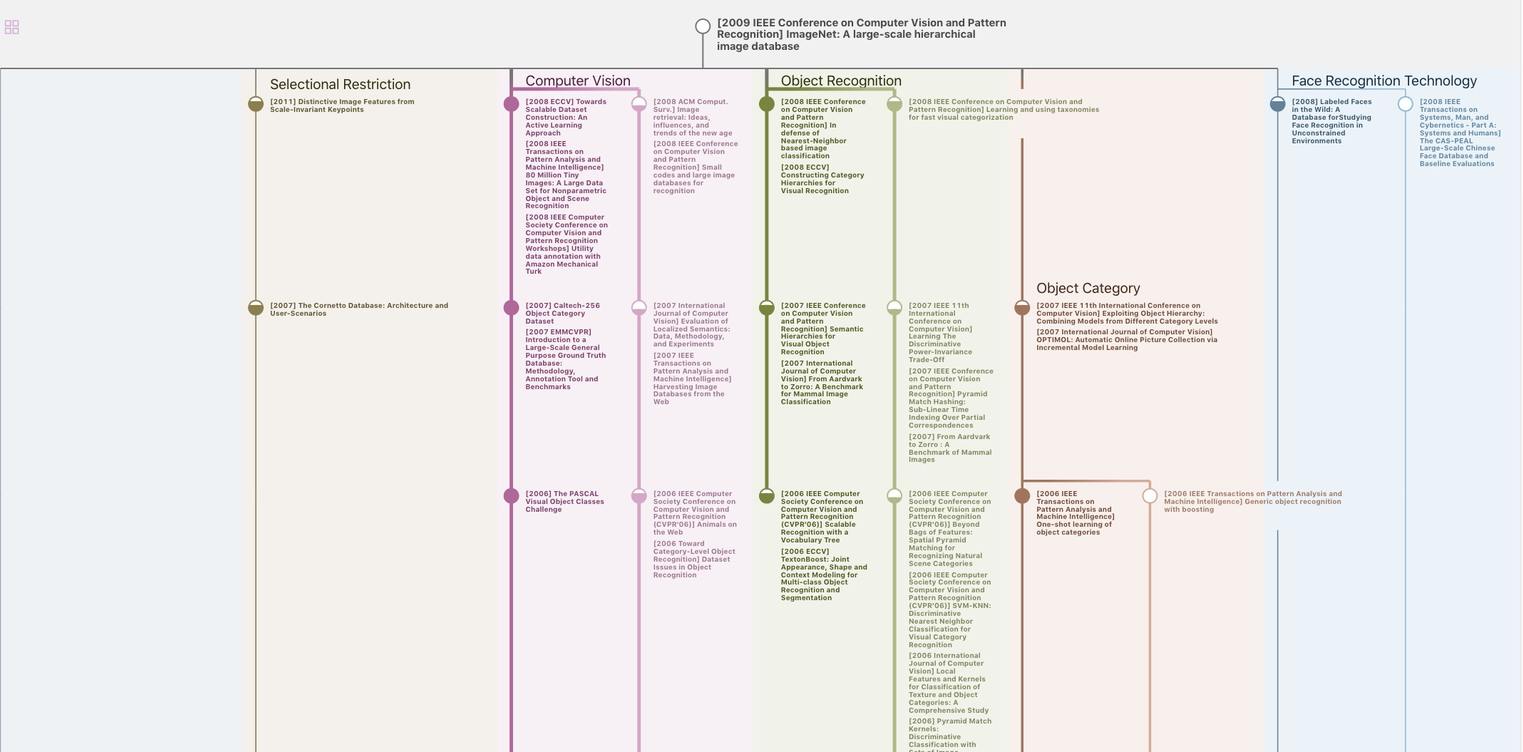
生成溯源树,研究论文发展脉络
Chat Paper
正在生成论文摘要