Optimizing Query Evaluations using Reinforcement Learning for Web Search.
SIGIR(2018)
摘要
In web search, typically a candidate generation step selects a small set of documents---from collections containing as many as billions of web pages---that are subsequently ranked and pruned before being presented to the user. In Bing, the candidate generation involves scanning the index using statically designed match plans that prescribe sequences of different match criteria and stopping conditions. In this work, we pose match planning as a reinforcement learning task and observe up to 20% reduction in index blocks accessed, with small or no degradation in the quality of the candidate sets.
更多查看译文
关键词
Web search,query evaluation,reinforcement learning
AI 理解论文
溯源树
样例
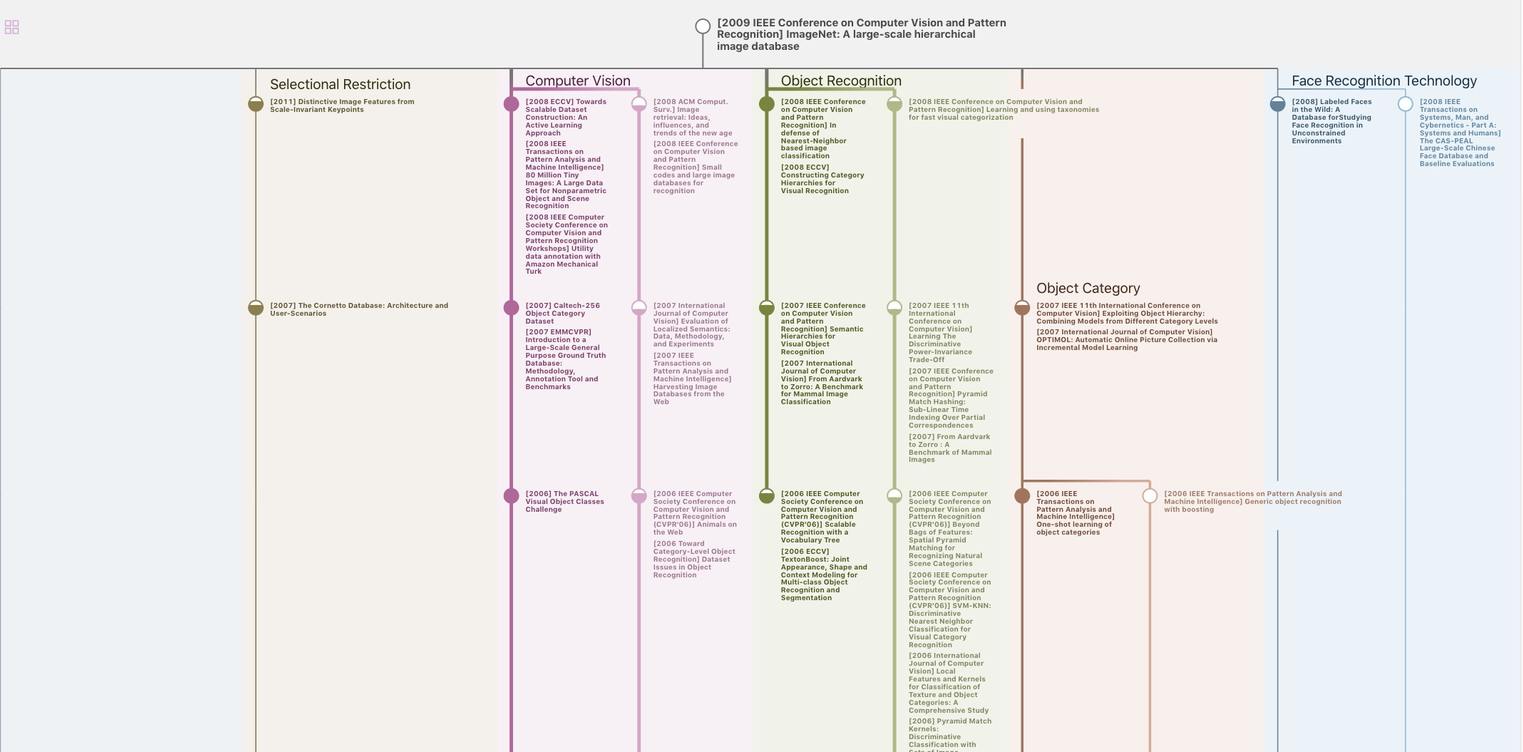
生成溯源树,研究论文发展脉络
Chat Paper
正在生成论文摘要