Learning both matching cost and smoothness constraint for stereo matching.
Neurocomputing(2018)
摘要
A typical stereo matching algorithm involves calculating of the matching cost and smoothness constraint. Recently some algorithms show the good performances by learning the matching cost, but the learning of smoothness constraint have been little researched. This paper focuses on both aspects by using convolutional neural networks. The proposed method first learns a Euclidean embedding for each image using a convolutional neural network with a triplet-based loss function, where the matching cost is directly computed by the squared L2 distances between two vectors in the embedding space. Then we use similar convolutional neural networks to learn the smoothness constraint, and a generalized Semiglobal Matching algorithm is proposed to estimate the disparity. The proposed method has a comparable performance with the state-of-the-art algorithms by using smaller and shallower networks, and the experiments show that learning both of matching cost and smoothness constraint has positive effects for stereo matching.
更多查看译文
关键词
Stereo matching,Convolutional neural network,Smoothness constraint,Semiglobal matching
AI 理解论文
溯源树
样例
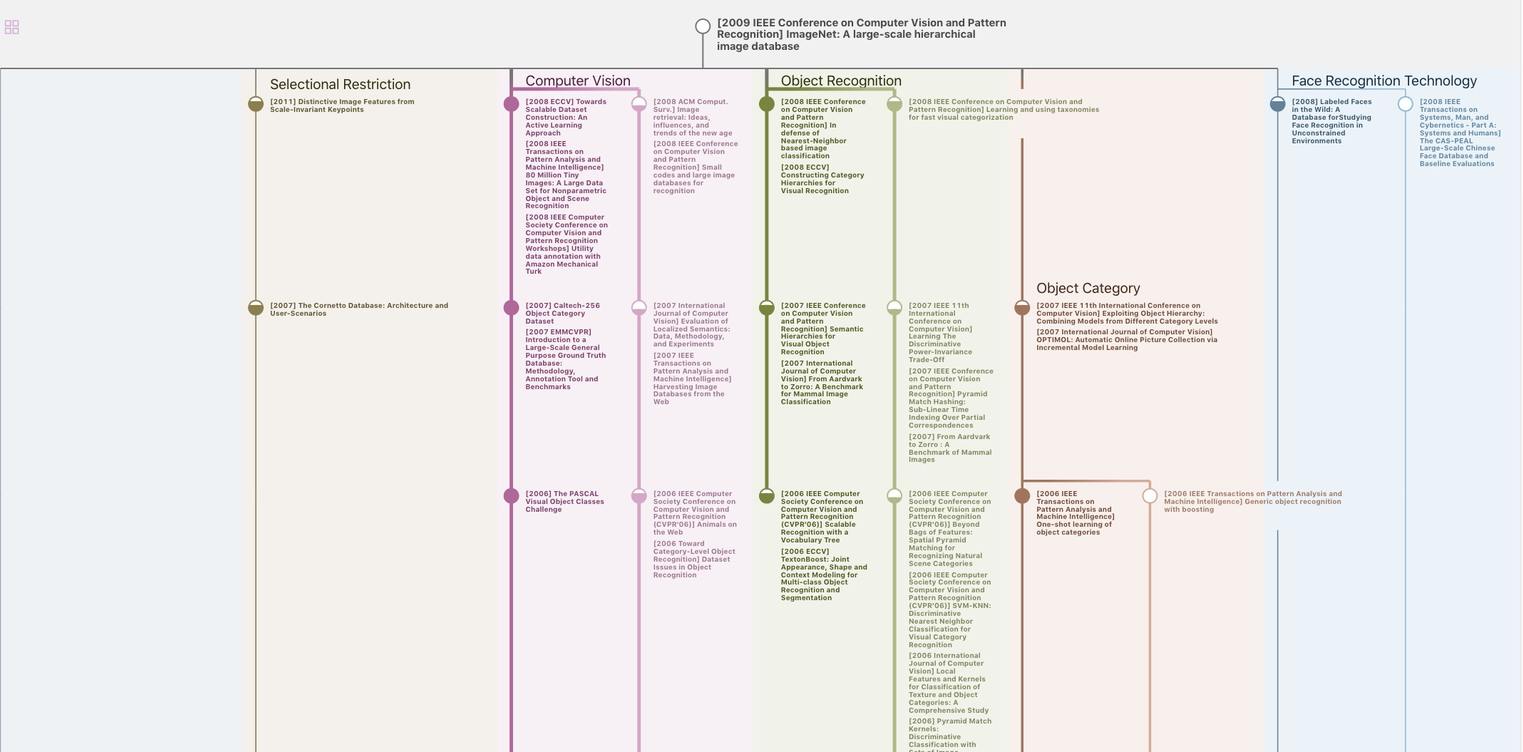
生成溯源树,研究论文发展脉络
Chat Paper
正在生成论文摘要