Sharp Convergence Rates for Langevin Dynamics in the Nonconvex Setting.
arXiv: Machine Learning(2018)
摘要
We study the problem of sampling from a distribution where the negative logarithm of the target density is $L$-smooth everywhere and $m$-strongly convex outside a ball of radius $R$, but potentially non-convex inside this ball. We study both overdamped and underdamped Langevin MCMC and prove upper bounds on the time required to obtain a sample from a distribution that is within $epsilon$ of the target distribution in $1$-Wasserstein distance. For the first-order method (overdamped Langevin MCMC), the time complexity is $tilde{mathcal{O}}left(e^{cLR^2}frac{d}{epsilon^2}right)$, where $d$ is the dimension of the underlying space. For the second-order method (underdamped Langevin MCMC), the time complexity is $tilde{mathcal{O}}left(e^{cLR^2}frac{sqrt{d}}{epsilon}right)$ for some explicit positive constant $c$. Surprisingly, the convergence rate is only polynomial in the dimension $d$ and the target accuracy $epsilon$. It is however exponential in the problem parameter $LR^2$, which is a measure of non-logconcavity of the target distribution.
更多查看译文
AI 理解论文
溯源树
样例
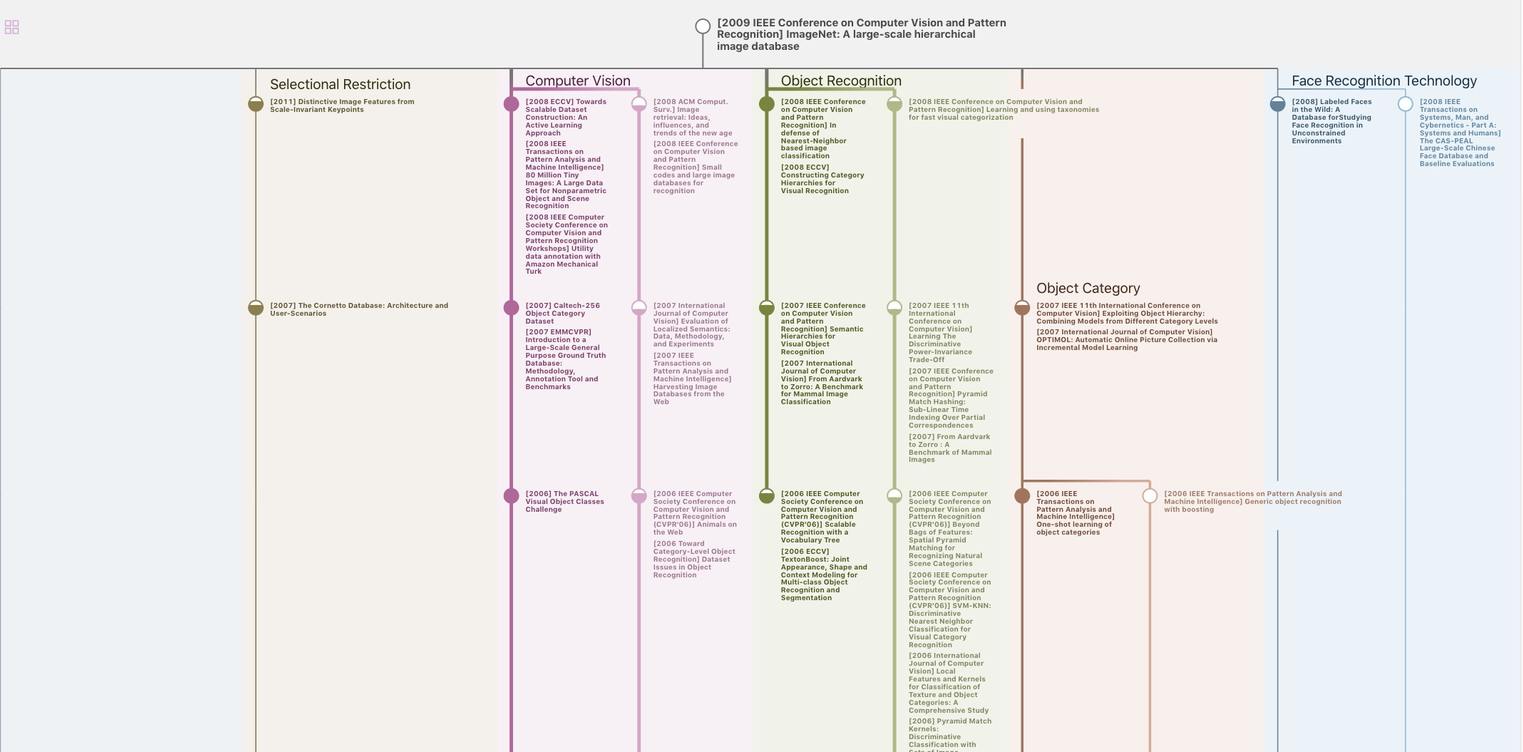
生成溯源树,研究论文发展脉络
Chat Paper
正在生成论文摘要