Fast and Precise Hippocampus Segmentation Through Deep Convolutional Neural Network Ensembles and Transfer Learning
Neuroinformatics(2019)
摘要
Automatic segmentation of the hippocampus from 3D magnetic resonance imaging mostly relied on multi-atlas registration methods. In this work, we exploit recent advances in deep learning to design and implement a fully automatic segmentation method, offering both superior accuracy and fast result. The proposed method is based on deep Convolutional Neural Networks (CNNs) and incorporates distinct segmentation and error correction steps. Segmentation masks are produced by an ensemble of three independent models, operating with orthogonal slices of the input volume, while erroneous labels are subsequently corrected by a combination of Replace and Refine networks. We explore different training approaches and demonstrate how, in CNN-based segmentation, multiple datasets can be effectively combined through transfer learning techniques, allowing for improved segmentation quality. The proposed method was evaluated using two different public datasets and compared favorably to existing methodologies. In the EADC-ADNI HarP dataset, the correspondence between the method’s output and the available ground truth manual tracings yielded a mean Dice value of 0.9015, while the required segmentation time for an entire MRI volume was 14.8 seconds. In the MICCAI dataset, the mean Dice value increased to 0.8835 through transfer learning from the larger EADC-ADNI HarP dataset.
更多查看译文
关键词
Convolutional neural networks,Deep learning,Error correction,Hippocampus segmentation,Magnetic resonance imaging,Transfer learning
AI 理解论文
溯源树
样例
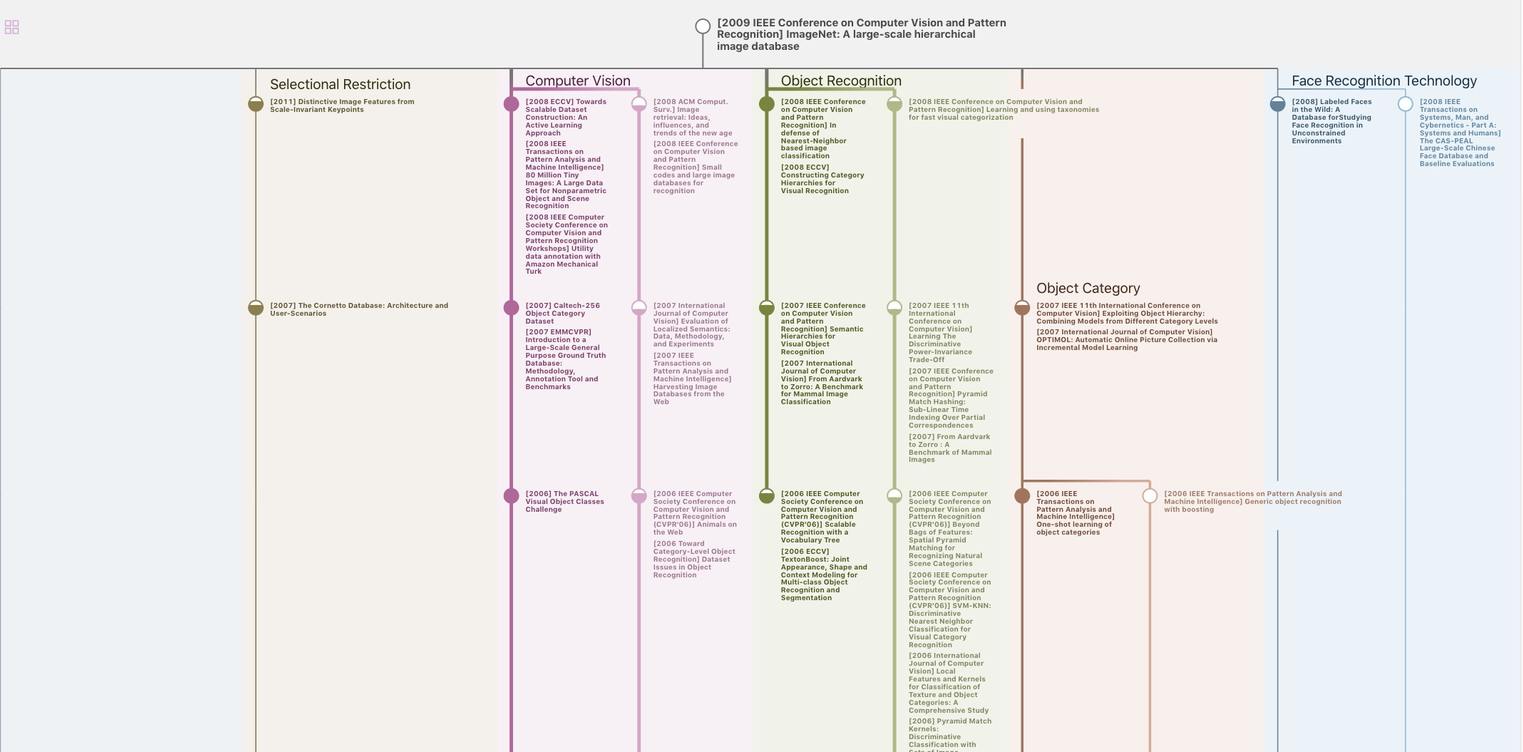
生成溯源树,研究论文发展脉络
Chat Paper
正在生成论文摘要