Coupled Mixed Model for joint genetic analysis of complex disorders from independently collected data sets: application to Alzheimer's disease and substance use disorder
bioRxiv(2019)
摘要
In the last decade, Genome-wide Association studies (GWASs) have contributed to decoding the human genome by uncovering genetic variations associated with diseases. ``Joint analysis99, which involves analyzing multiple independently generated GWAS data sets, has been explored extensively as follow-ups. However, most of the analyses remain in the preliminary stage. In this paper, we propose a method, called Coupled Mixed Model (CMM), that allows performing the joint analysis of GWAS on two independently collected data sets with different phenotypes, using a set of multivariate sparse mixed models. The CMM method does not require the data sets to have the same phenotypes as it aims to infer the uncollected phenotypes using statistical learning modeling. Moreover, CMM takes account of the confounders due to population stratification, family structures, and cryptic relatedness, as well as the confounders arising during data collection that frequently appear in joint genetic studies. In this work, we verify the performance of our method using simulation experiments. We also conduct a real data joint analysis of two independent data sets which were generated for investigation of genetic association for Alzheimer9s disease and substance use disorder, respectively. Our results reveal new insights into these diseases. A Python implementation of the software is available at: https://github.com/HaohanWang/CMM
更多查看译文
AI 理解论文
溯源树
样例
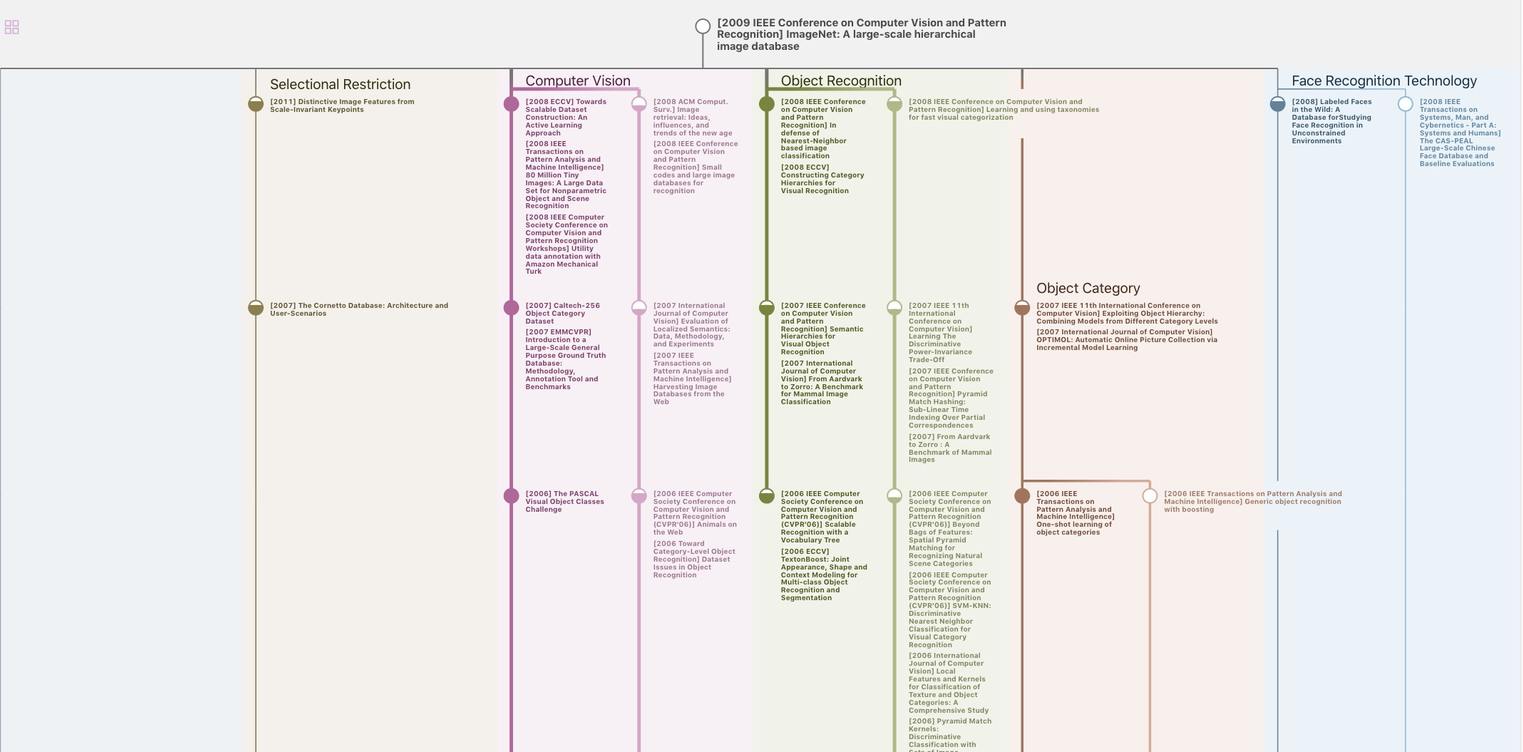
生成溯源树,研究论文发展脉络
Chat Paper
正在生成论文摘要