Identifying the Number of Clusters in Short Text Using Bayesian Nonparametric Model
2017 IEEE 29th International Conference on Tools with Artificial Intelligence (ICTAI)(2017)
摘要
Before inferring the real number of clusters in short text clustering, Dirichlet Multinomial Mixture (DMM) model makes assumption that there are at most Kmax clusters. In some cases, it is difficult to choose a proper Kmax beforehand. In the paper, we propose a novel model based on Pitman-Yor Process to capture the power-law phenomenon of the cluster distribution. Specifically, each text chooses one of the active clusters or a new cluster with probabilities derived from the Pitman-Yor Process Mixture model (PYPM). Different from DMM model, our model does not require Kmax as input. Discriminative words and nondiscriminative words are identified automatically to help enhance text clustering. Parameters are estimated efficiently by collapsed Gibbs sampling. The experiments on real-world datasets validate the effectiveness of the proposed model in comparison with other state-of-theart models.
更多查看译文
关键词
Pitman Yor Process,Short Text Clustering,Collapsed Gibbs Sampling
AI 理解论文
溯源树
样例
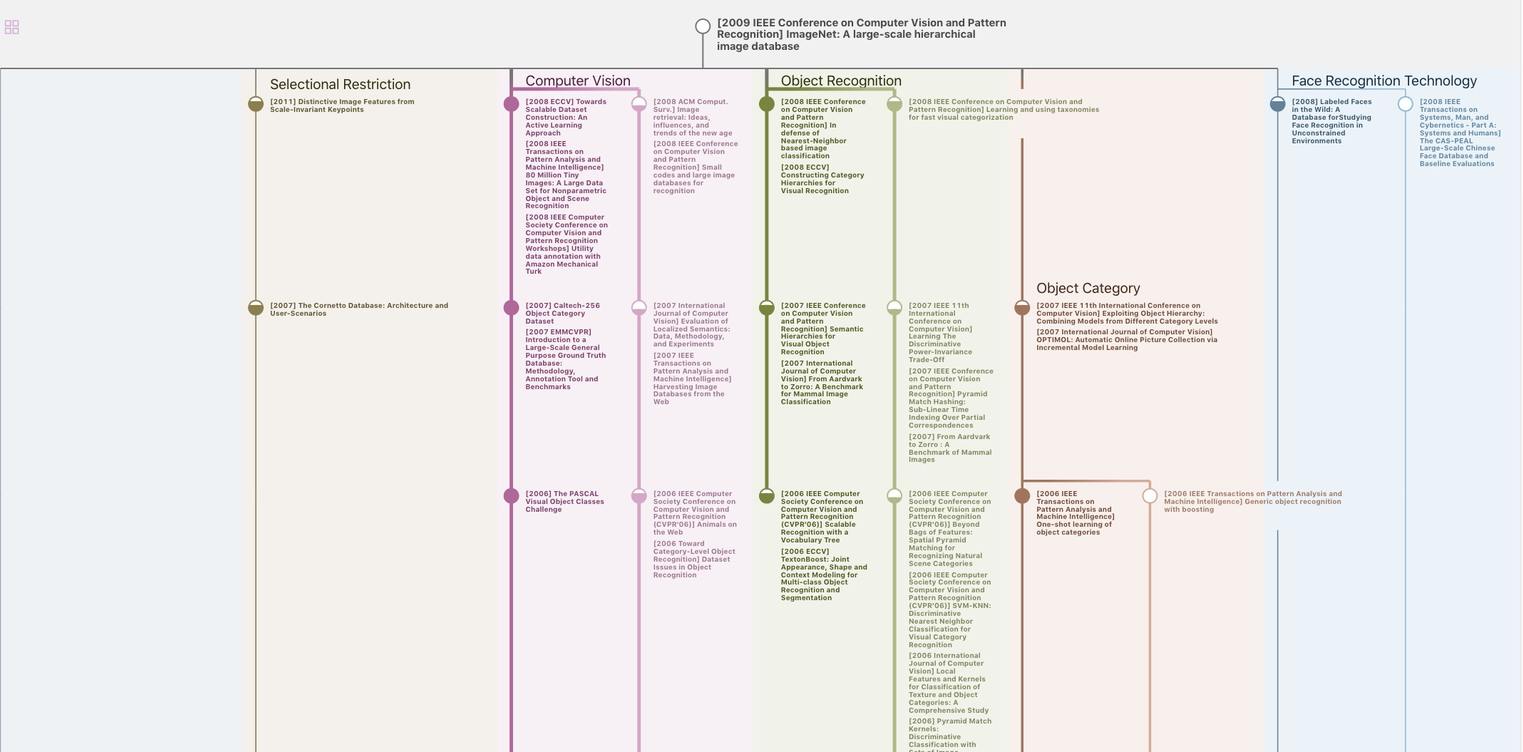
生成溯源树,研究论文发展脉络
Chat Paper
正在生成论文摘要