Genetic variant pathogenicity prediction trained using large-scale disease specific clinical sequencing datasets
bioRxiv(2019)
摘要
Background: Recent advances in high-throughput DNA sequencing technologies have expanded our understanding of the molecular underpinnings of various genetic disorders and have led to increased utilization of genomic tests by clinicians. However, each test can generate thousands of variants, and given the paucity of functional studies assessing each one of them, experimental validation of a variants clinical significance is not feasible for clinical laboratories. Therefore, many variants are reported as variants of unknown clinical significance due to this gap. However, the creation of large variant databases like the Genome Aggregation Database has significantly improved the interpretation of novel variants. Specifically, pathogenicity prediction for novel missense variants can now utilize features describing regional variant constraint. Constrained genomic regions are those that have an unusually low variant counts in the general population. Earlier pathogenicity classifiers tried to capture these regions using protein domains.Methods and Findings: Here we introduce one of the largest variant datasets derived from clinical sequencing panels to assess the utility of using old and new concepts of regional features as pathogenicity scores. This dataset is compiled from 17,071 patients surveyed with clinical genomic sequencing for cardiomyopathy, epilepsy, or rasopathies. We use this dataset to justify the necessity of disease specific classifiers, and train PathoPredictor, a disease specific ensemble classifier of pathogenicity based on regional constraint and variant level features.Conclusion: Disease specific features improve missense variant pathogenicity prediction. As such, PathoPredictor achieves an average precision greater than 90% for variants from all 112 tested disease genes while approaching 100% accuracy for some genes, making it superior to existing generic pathogenicity metrics it uses as features.
更多查看译文
关键词
machine learning,clinical sequencing,pathogenicity prediction
AI 理解论文
溯源树
样例
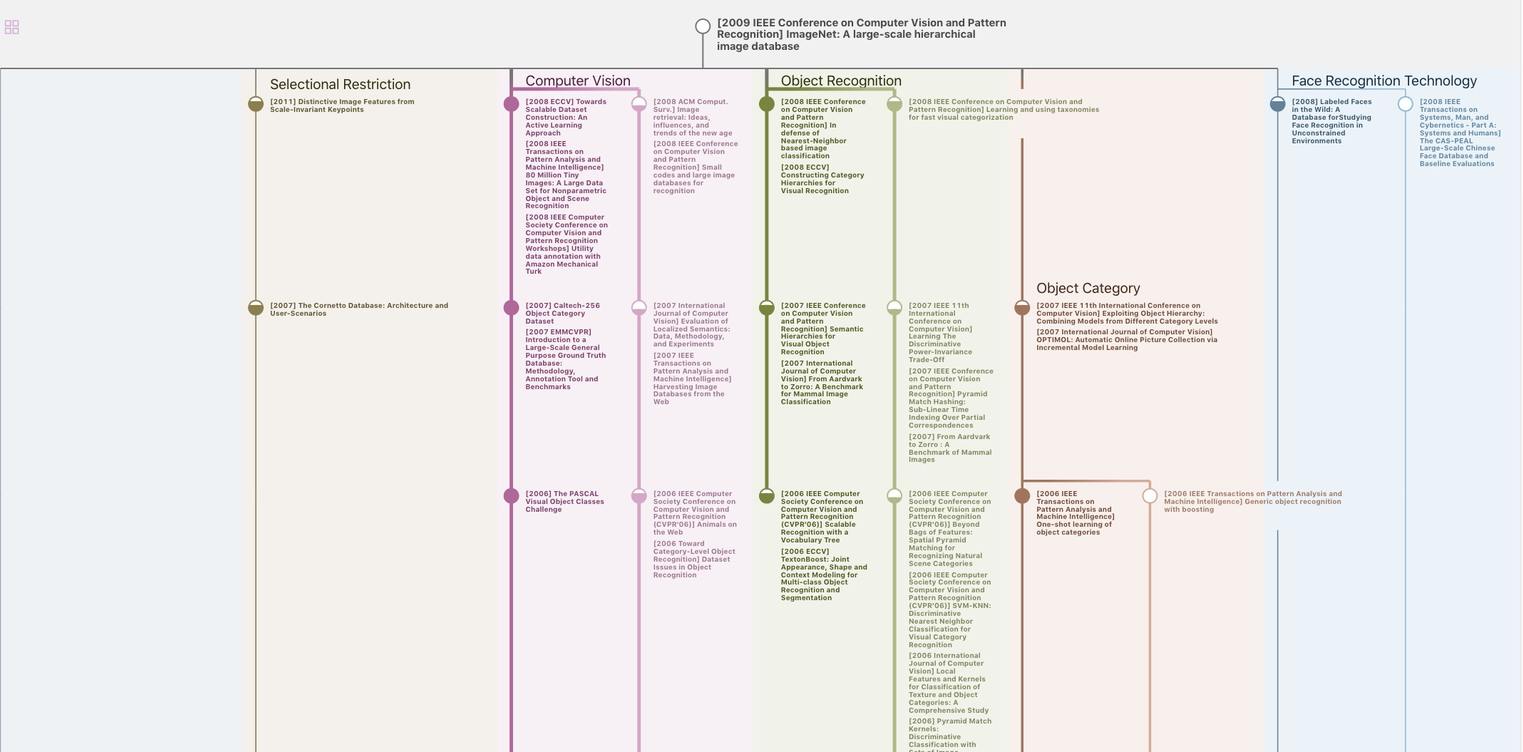
生成溯源树,研究论文发展脉络
Chat Paper
正在生成论文摘要