SnapTask: Towards Efficient Visual Crowdsourcing for Indoor Mapping
2018 IEEE 38th International Conference on Distributed Computing Systems (ICDCS)(2018)
摘要
Visual crowdsourcing (VCS) offers an inexpensive method to collect visual data for implementing tasks, such as 3D mapping and place detection, thanks to the prevalence of smartphone cameras. However, without proper guidance, participants may not always collect data from desired locations with a required Quality-of-Information (QoI). This often causes either a lack of data in certain areas, or extra overheads for processing unnecessary redundancy. In this work, we propose SnapTask, a participatory VCS system that aims at creating complete indoor maps by guiding participants to efficiently collect visual data of high QoI. It applies Structure-from-Motion (SfM) techniques to reconstruct 3D models of indoor environments, which are then converted into indoor maps. To increase coverage with minimal redundancy, SnapTask determines locations for the next data collection tasks by analyzing the coverage of the generated 3D model and the camera views of the collected images. In addition, it overcomes the limitations of SfM techniques by utilizing crowdsourced annotations to reconstruct featureless surfaces (e.g. glass walls) in the 3D model. According to a field test in a library, the indoor map generated by SnapTask successfully reconstructs 100% of the library walls and 98.12% of objects and traversal areas within the library. With the same amount of input data our design of guided data collection increases the map coverage by 20.72% and 34.45%, respectively, compared with unguided participatory and opportunistic VCS.
更多查看译文
关键词
crowdsourcing,indoor mapping,participatory crowdsourcing,featureless reconstruction
AI 理解论文
溯源树
样例
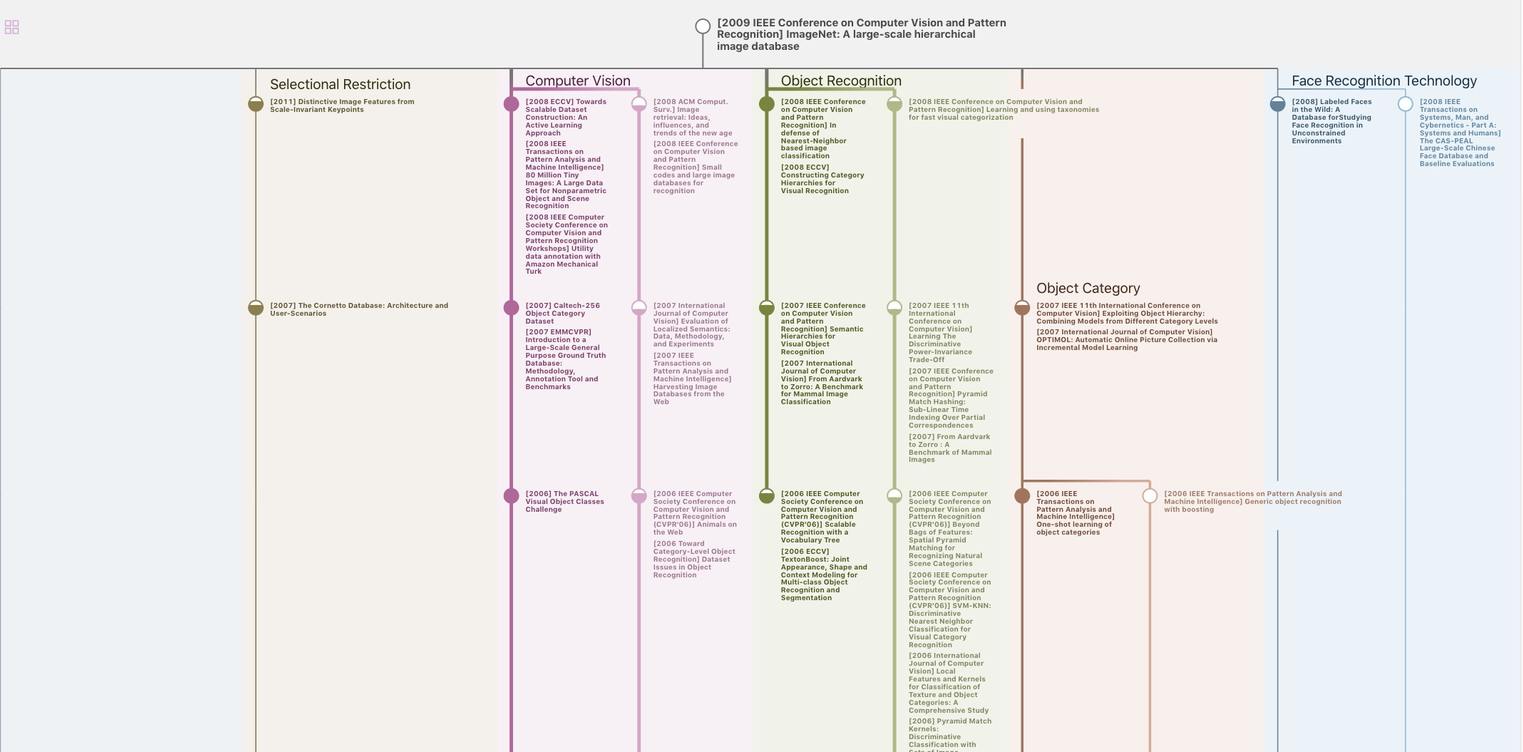
生成溯源树,研究论文发展脉络
Chat Paper
正在生成论文摘要