Tracking depression severity from audio and video based on speech articulatory coordination
Computer Speech & Language(2019)
摘要
The ability to track depression severity over time using passive sensing of speech would enable frequent and inexpensive monitoring, allowing rapid assessment of treatment efficacy as well as improved long term care of individuals at high risk for depression. In this paper an algorithm is proposed that estimates the articulatory coordination of speech from audio and video signals, and uses these coordination features to learn a prediction model to track depression severity with treatment. In addition, the algorithm is able to adapt its prediction model to an individual’s baseline data in order to improve tracking accuracy. The algorithm is evaluated on two data sets. The first is the Wyss Institute Biomarkers for Depression (WIBD) multi-modal data set, which includes audio and video speech recordings. The second data set was collected by Mundt et al (2007) and contains audio speech recordings only. The data sets are comprised of patients undergoing treatment for depression as well as control subjects. In its within-subject tracking of clinical Hamilton depression (HAM-D) ratings, the algorithm achieves root mean squared error (RMSE) of 5.49 with Spearman correlation of r = 0.63 on the WIBD data set, and achieves RMSE = 5.99 with r = 0.48 on the Mundt data set.
更多查看译文
关键词
Depression,Speech,Articulation,Coordination,Audio,Video
AI 理解论文
溯源树
样例
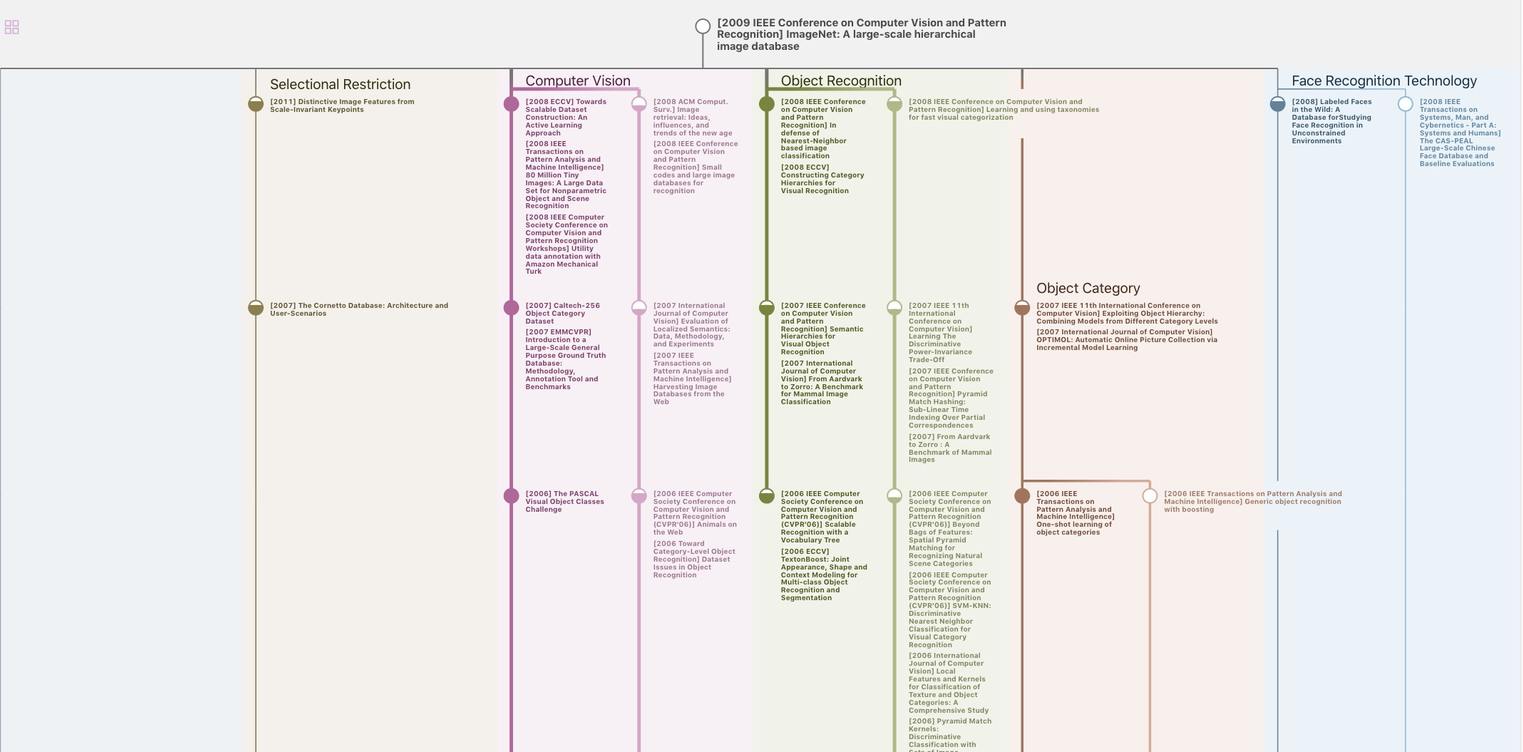
生成溯源树,研究论文发展脉络
Chat Paper
正在生成论文摘要