Prototype-Based Compound Discovery using Deep Generative Models.
MOLECULAR PHARMACEUTICS(2018)
摘要
Designing a new drug is a lengthy and expensive process. As the space of potential molecules is very large (Polishchuk, P.G.; Madzhidov, T. I.; Varnek, A. Estimation of the size of drug-like chemical space based on GDB-17 data. J. Comput.-Aided Mol. Des. 2013, 27, 675-679 10.1007/s10822-013-9672-4), a common technique during drug discovery is to start from a molecule which already has some of the desired properties. An interdisciplinary team of scientists generates hypothesis about the required changes to the prototype. In this work, we develop a deep-learning unsupervised-approach that automatically generates potential drug molecules given a prototype drug. We show that the molecules generated by the system are valid molecules and significantly different from the prototype drug. Out of the compounds generated by the system, we identified 35 known FDA-approved drugs. As an example, our system generated isoniazid, one of the main drugs for tuberculosis. We suggest several ranking functions for the generated molecules and present results that the top ten generated molecules per prototype drug contained in our retrospective experiments 23 known FDA-approved drugs.
更多查看译文
关键词
prototype-based drug discovery,compound design,generative models,deep learning for medicine
AI 理解论文
溯源树
样例
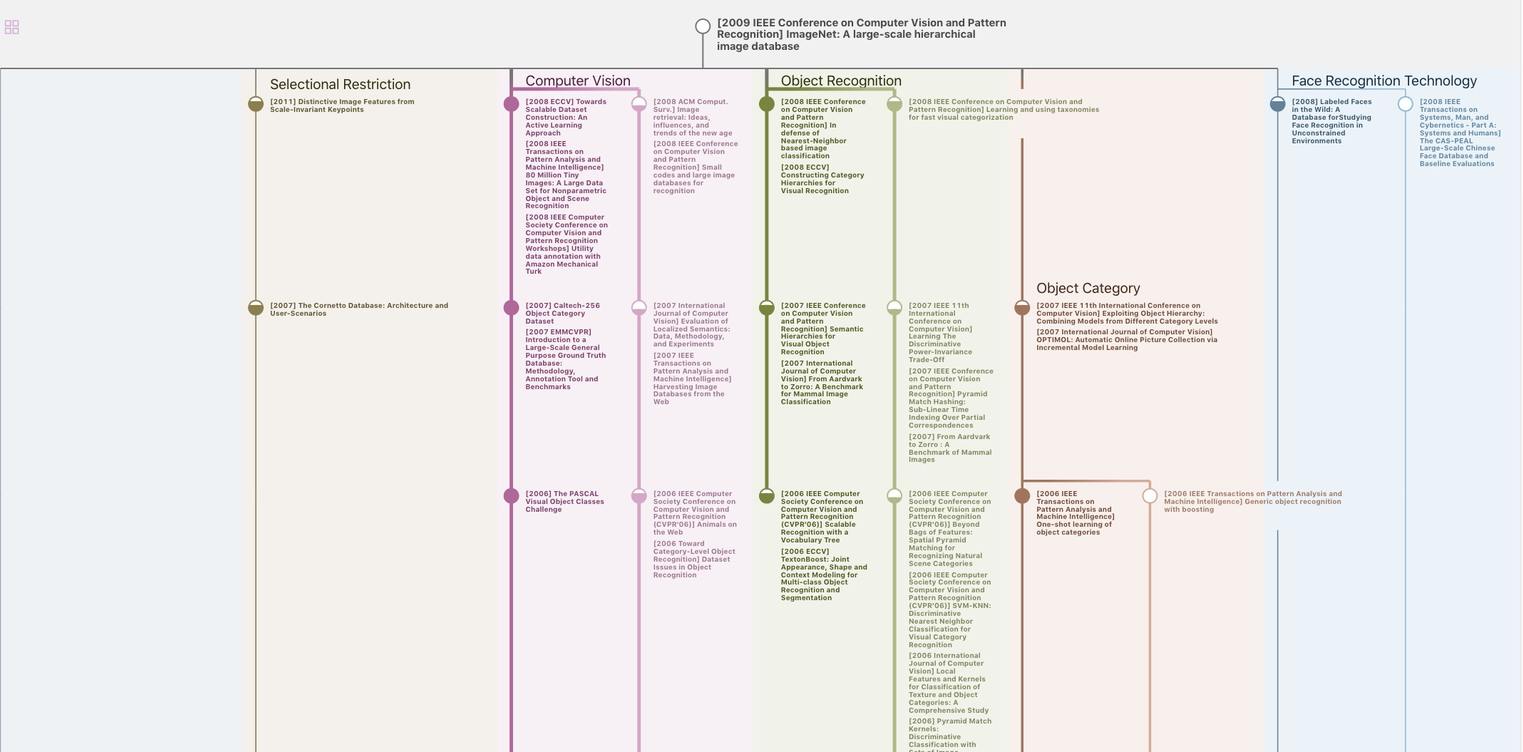
生成溯源树,研究论文发展脉络
Chat Paper
正在生成论文摘要