Clustered Optimization of a Small-Scale Robot Swarm with Minimal On-Board Sensing
2018 ANNUAL AMERICAN CONTROL CONFERENCE (ACC)(2018)
摘要
This paper explores the ability of a swarm of small-scale robots to optimize robot locomotion speed if only extremely limited on-board sensing capability is granted for each individual robot. This is done to assess benefits of swarm optimization versus individual control. The optimization of simulated robot locomotion speed is evaluated under multiple classical and machine learning algorithms. Furthermore, a new algorithm, Clustered Particle Swarm Optimization (CPSO), is developed based on existing optimization and clustering algorithms. The algorithms are based on an experimentally-validated nominal dynamic model for small-scale robots, with environmental parameters extended over a wider range of uncertainty. The nonlinear relationship between multiple actuation parameters and robot performance, specifically robot speed in this work, encourages a numerical method to search for a set of actuation parameters that leads to a higher robot speed. Three algorithms are presented and compared, including Simulated Annealing (SA), Particle Swarm Optimization (PSO), and CPSO. The comparison between SA and PSO demonstrates the utility of optimizing robots in a swarm. By increasing the number of robots, the optimizing iteration number can be reduced. The comparison of simulation of PSO and CPSO shows a 23% improvement with minimal sensing assumed from the robots. The single sensing feature, selected manually using phase plots, is the average foot height at a certain actuation phase in each cycle.
更多查看译文
关键词
simulated robot locomotion speed,multiple classical machine learning algorithms,CPSO,clustering algorithms,experimentally-validated nominal dynamic model,small-scale robots,multiple actuation parameters,robot performance,specifically robot speed,higher robot speed,single sensing feature,small-scale robot swarm,on-board sensing capability,individual robot,iteration number optimization,clustered particle swarm optimization
AI 理解论文
溯源树
样例
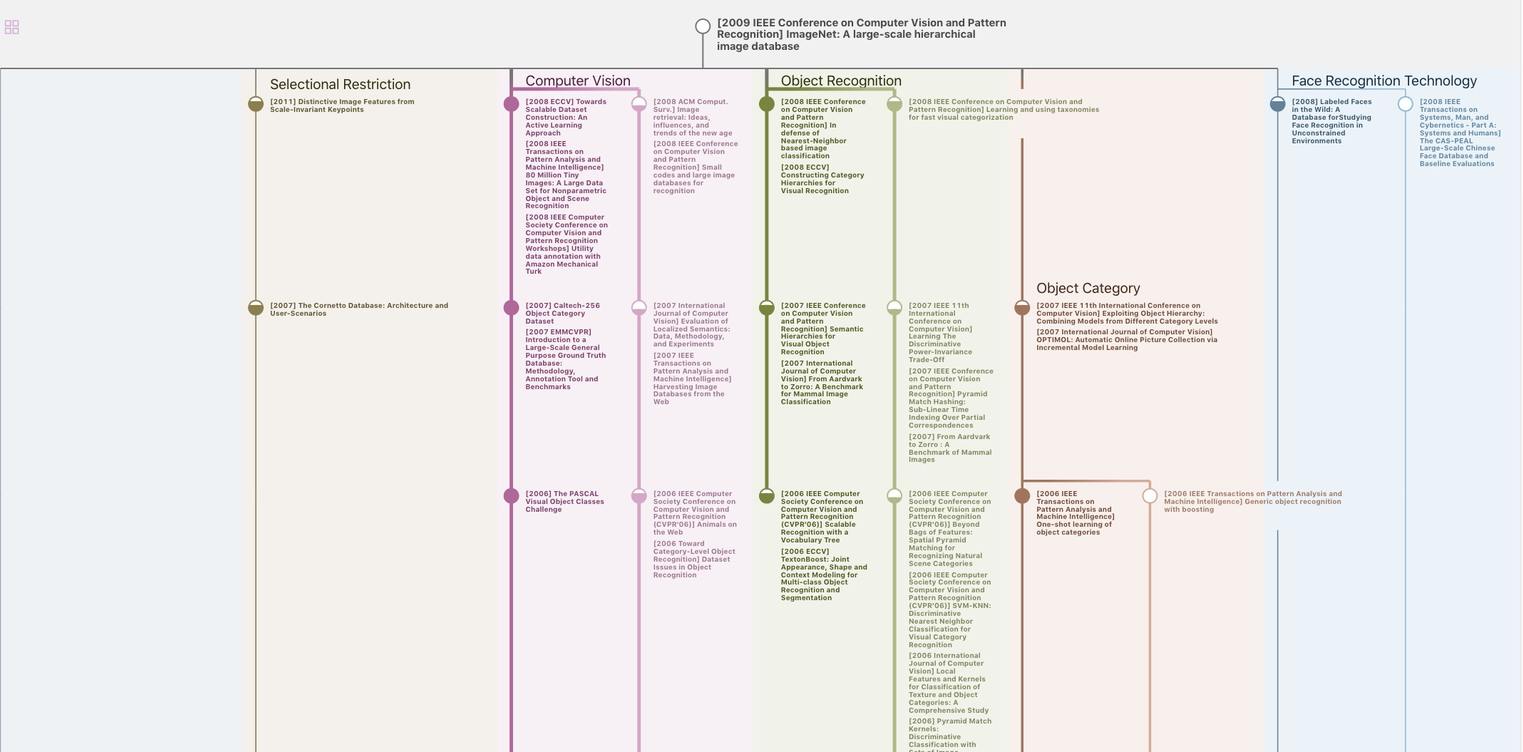
生成溯源树,研究论文发展脉络
Chat Paper
正在生成论文摘要