Simultaneous denoising and moving object detection using low rank approximation.
Future Generation Computer Systems(2019)
摘要
Moving Object Detection (MOD) and Background Subtraction (BS) are the fundamental tasks in video surveillance systems. But, one of the major challenges which badly affects the accuracy of detection is the presence of noise in the captured video sequence. In this work, we propose a new moving object detection method from noisy video data named as De-Noising and moving object detection by Low Rank approximation and l1-TV regularization (DNLRl1TV). In general, background of videos are assumed to lie in a low-rank subspace and moving objects are assumed to be piecewise smooth in the spatial and temporal direction. The proposed method consolidates the nuclear norm, l2-norm, l1-norm and Total Variation (TV) regularization in a unified framework to obtain simultaneous denoising and MOD performance. The nuclear norm exploits the low-rank property of the background, the sparsity is enhanced by the l1-norm, TV regularization is used to explore the foreground spatial smoothness and noise is detected and removed by the l2-norm regularization. Extensive experiments demonstrate that the proposed method outperforms the state-of-the-art approaches in terms of denoising capability and detection accuracy.
更多查看译文
关键词
Moving object detection,Low rank recovery,Background subtraction,Robust principle component analysis
AI 理解论文
溯源树
样例
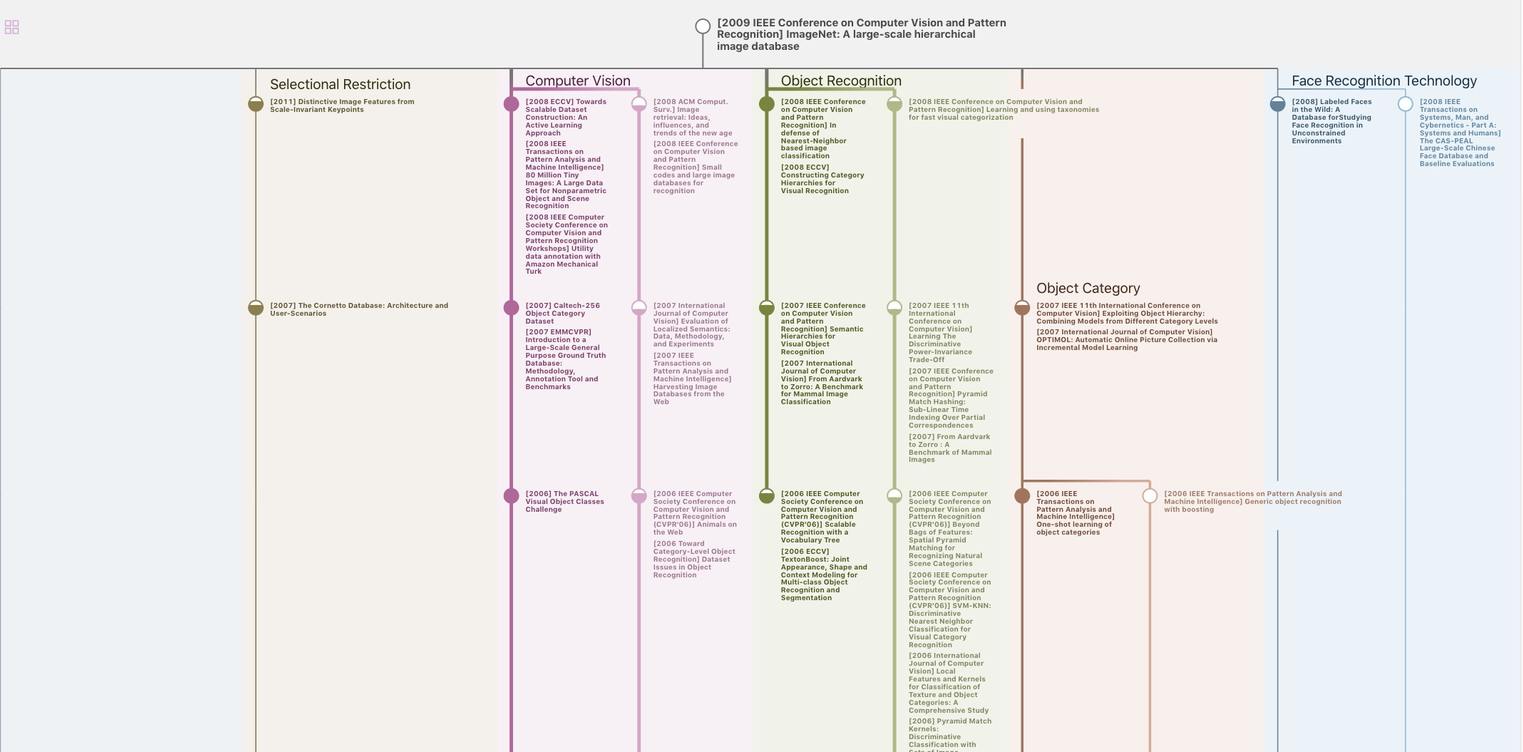
生成溯源树,研究论文发展脉络
Chat Paper
正在生成论文摘要