Context-Based Time Score: An Effective Similarity Function For Link Prediction In Social Networks
WEBMEDIA'18: PROCEEDINGS OF THE 24TH BRAZILIAN SYMPOSIUM ON MULTIMEDIA AND THE WEB(2018)
摘要
Online social networks (OSNs) have become an extremely relevant way for modeling social interactions among people in a group or community. A comprehensive set of studies has analyzed how to predict which new interactions will occur between the participants from OSNs. The problem of predicting new interactions is formally stated as link prediction. Most studies related to link prediction is based on similarity functions that use data from different types such as topological (data about the network structure), temporal (chronological interaction data) or contextual (participant and link attributes), usually available on OSNs. However, none of those studies uses the different types of data simultaneously, leading to poor incorporation of the different aspects of the OSNs in link prediction. To address this issue, the present paper introduces a new similarity function called Context-based Time Score (CTS), which combines topological, temporal and contextual data to improve accuracy in predicting the occurrence of new connections. Experiments with ten different datasets revealed that CTS can outperform similarity functions that do not take the three types of data simultaneously.
更多查看译文
关键词
network theory (graphs), online social networks, data mining, link mining, link prediction, unsupervised approach
AI 理解论文
溯源树
样例
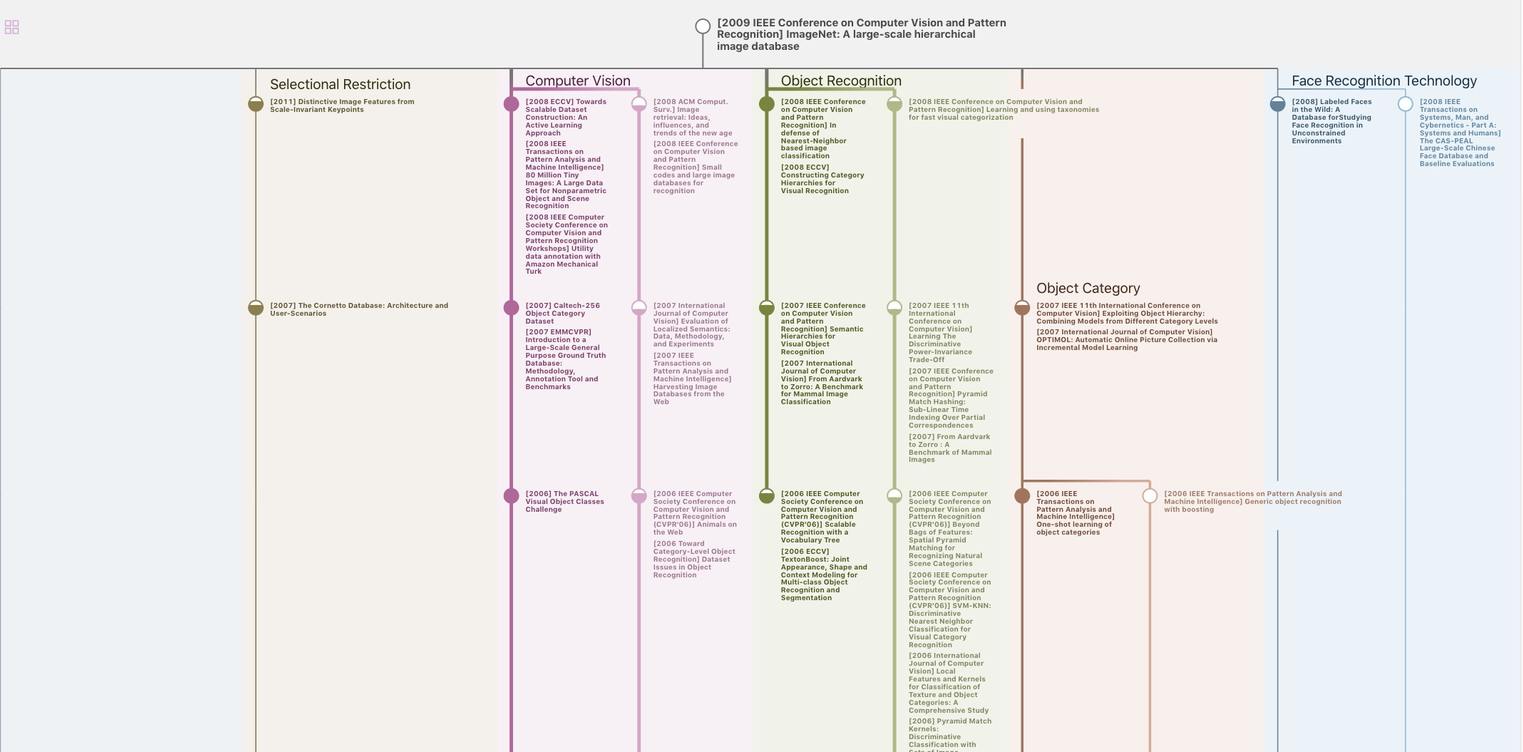
生成溯源树,研究论文发展脉络
Chat Paper
正在生成论文摘要