Inexact trust-region algorithms on Riemannian manifolds.
ADVANCES IN NEURAL INFORMATION PROCESSING SYSTEMS 31 (NIPS 2018)(2018)
摘要
We consider an inexact variant of the popular Riemannian trust-region algorithm for structured big-data minimization problems. The proposed algorithm approximates the gradient and the Hessian in addition to the solution of a trust-region sub-problem. Addressing large-scale finite-sum problems, we specifically propose sub-sampled algorithms with a fixed bound on sub-sampled Hessian and gradient sizes, where the gradient and Hessian are computed by a random sampling technique. Numerical evaluations demonstrate that the proposed algorithms outperform state-of-the-art Riemannian deterministic and stochastic gradient algorithms across different applications.
更多查看译文
关键词
matrix completion,principal components analysis,riemannian manifolds
AI 理解论文
溯源树
样例
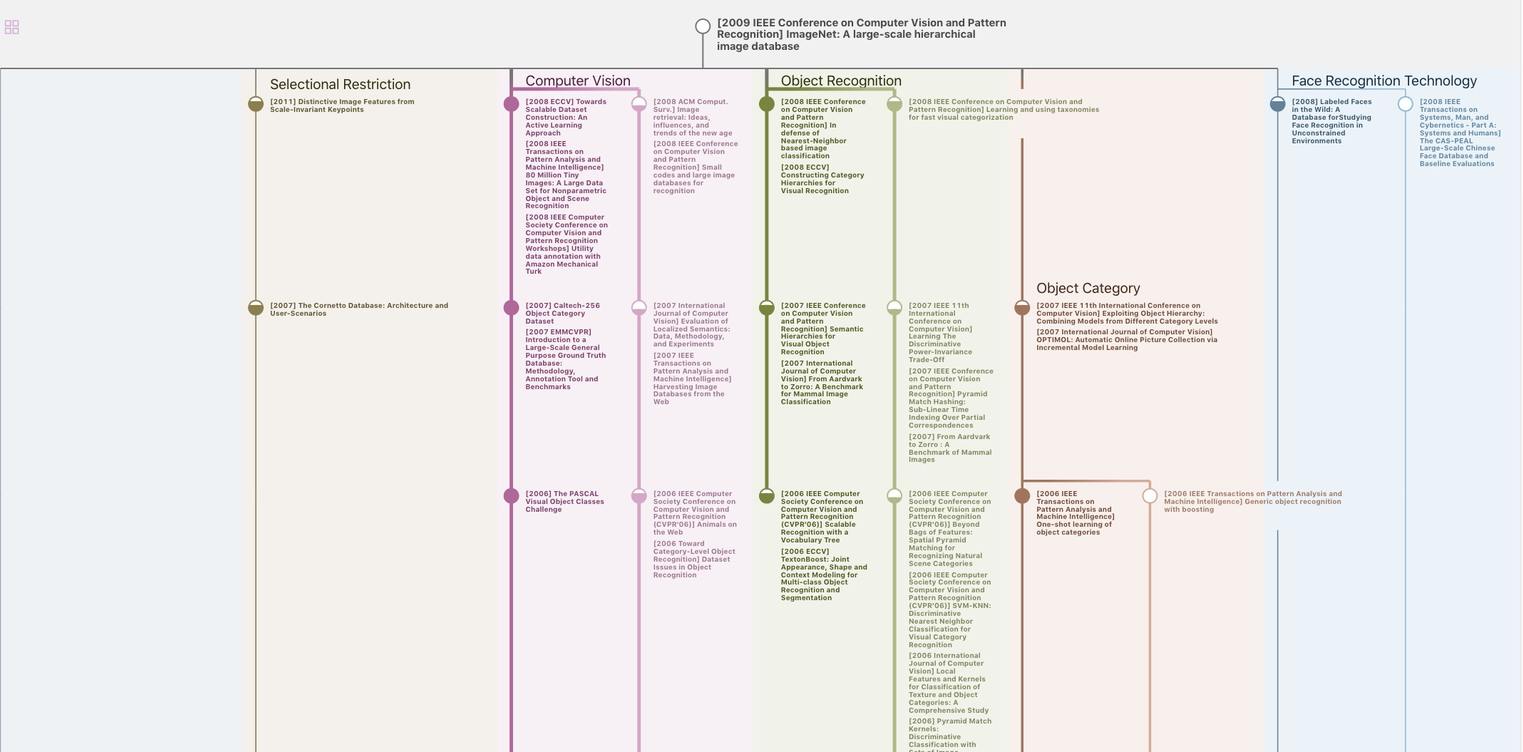
生成溯源树,研究论文发展脉络
Chat Paper
正在生成论文摘要