Bag of local features for person re-identification on large-scale datasets.
JOURNAL OF ELECTRONIC IMAGING(2018)
摘要
In recent years, large-scale person re-identification has attracted a lot of attention from video surveillance. Usual approaches addressing this task either learn the effective feature embeddings or design the learning architectures to obtain discriminative metrics. Most of them only focus on improving the accuracy of recognition but neglect retrieval efficiency. To improve the accuracy and efficiency of person re-identification simultaneously, an accurate and fast method is proposed based on the bag of visual words (BoVW) model, which has widely been applied in image retrieval. A bag of local features is developed to simplify feature representation for person re-identification. Cross-view dictionary learning is used to eliminate the redundancy of these local features. These local features consist of scale invariant feature transform and local maximal occurrence representation (LOMO) that are invariant in scale and color, respectively. Finally, integrated BoVW histograms are obtained, which encode the images by k-means clustering. Experiments conducted on the CUHK03, Market1501, and MARS datasets show that the proposed method performs favorably against existing approaches. (c) 2018 SPIE and IS&T
更多查看译文
关键词
person re-identification,bag of visual words,cross-view dictionary learning,reranking,large-scale datasets
AI 理解论文
溯源树
样例
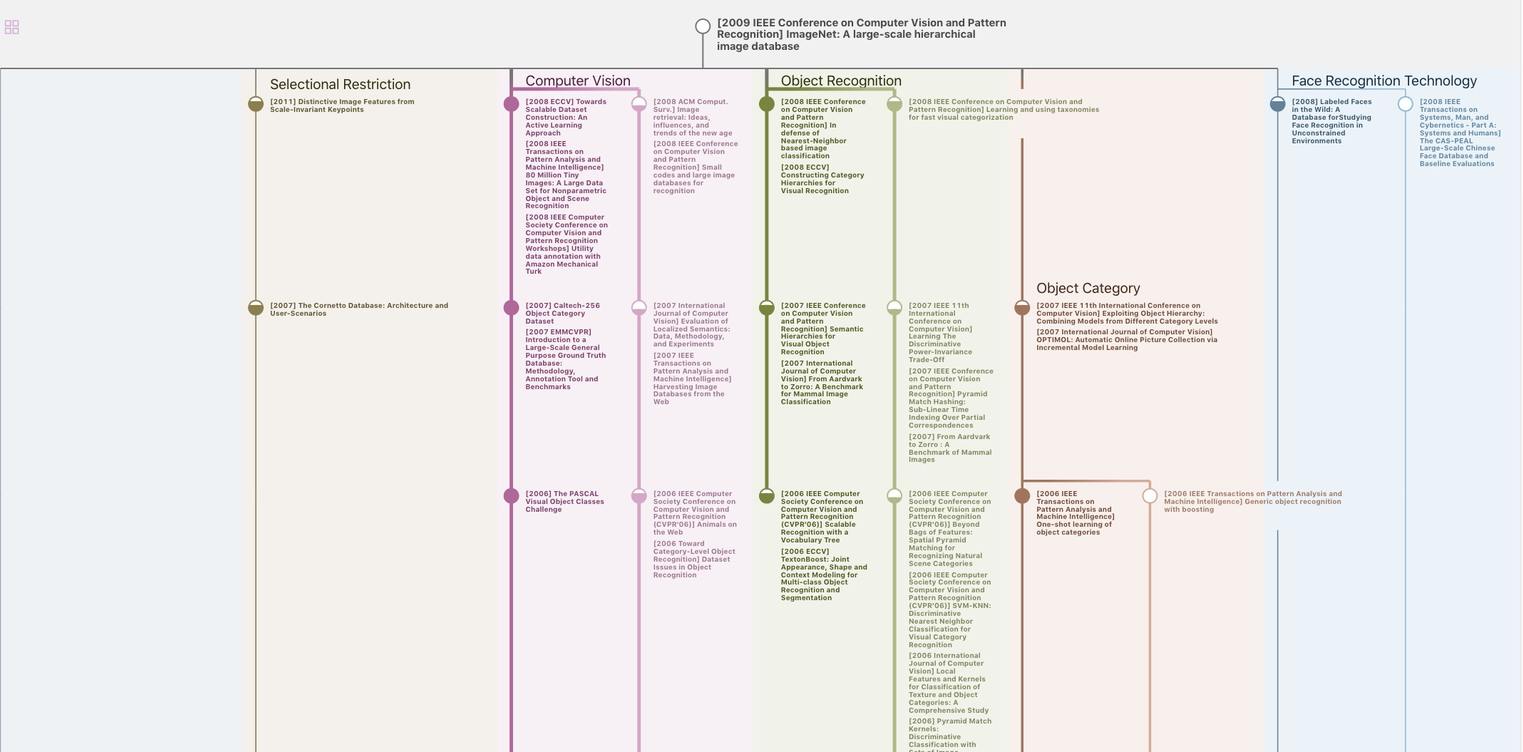
生成溯源树,研究论文发展脉络
Chat Paper
正在生成论文摘要