Adrenal incidentaloma: machine learning-based quantitative texture analysis of unenhanced CT can effectively differentiate sPHEO from lipid-poor adrenal adenoma.
JOURNAL OF CANCER(2018)
摘要
Objective: To evaluate the feasibility and accuracy of machine learning based texture analysis of unenhanced CT images in differentiating subclinical pheochromocytoma (sPHEO) from lipid-poor adenoma (LPA) in adrenal incidentaloma (AI). Methods: Seventy-nine patients with 80 LPA and 29 patients with 30 sPHEO were included in the study. Texture parameters were derived using imaging software (MaZda). Thirty texture features were selected and LPA was performed for the features selected. The number of positive features was used to predict results. Logistic multiple regression analysis was performed on the 30 texture features, and a predictive equation was created based on the coefficients obtained. Results: LPA yielded a misclassification rate of 19.39% in differentiating sPHEO from LPA. Our predictive model had an accuracy rate of 94.4% (102/108), with a sensitivity of 86.2% (25/29) and a specificity of 97.5% (77/79) for differentiation. When the number of positive features was greater than 8, the accuracy of prediction was 85.2% (92/108), with a sensitivity of 96.6% (28/29) and a specificity of 81% (64/79). Conclusions: Machine learning-based quantitative texture analysis of unenhanced CT may be a reliable quantitative method in differentiating sPHEO from LPA when AI is present.
更多查看译文
关键词
Texture analysis,adrenal incidentaloma,sPHEO,lipid-poor adrenal adenoma,differentiation
AI 理解论文
溯源树
样例
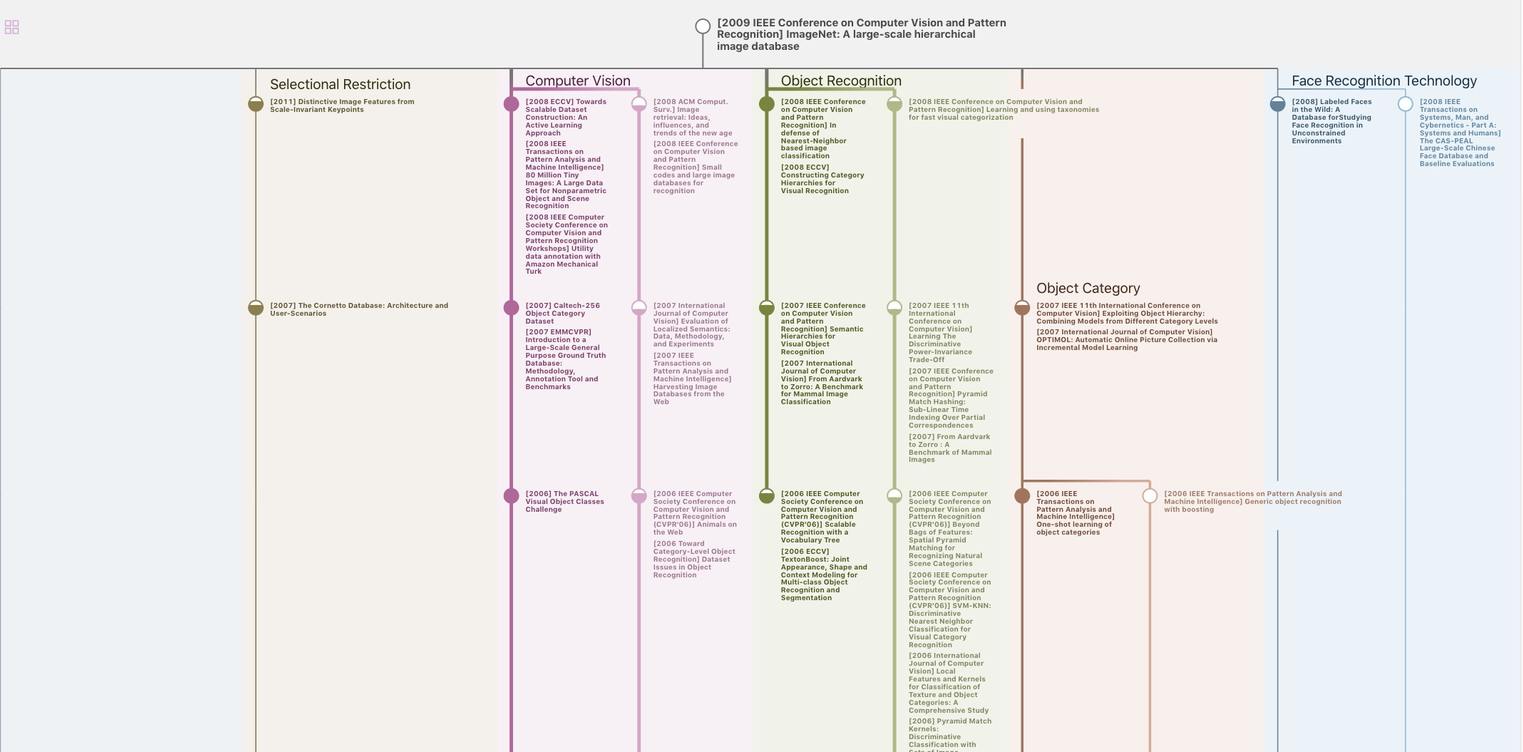
生成溯源树,研究论文发展脉络
Chat Paper
正在生成论文摘要