SuperDepth: Self-Supervised, Super-Resolved Monocular Depth Estimation
arXiv (Cornell University)(2018)
摘要
Recent techniques in self-supervised monocular depth estimation are approaching the performance of supervised methods, but operate in low resolution only. We show that high resolution is key towards high-fidelity self-supervised monocular depth prediction. Inspired by recent deep learning methods for Single-Image Super-Resolution, we propose a sub-pixel convolutional layer extension for depth super-resolution that accurately synthesizes high-resolution disparities from their corresponding low-resolution convolutional features. In addition, we introduce a differentiable flip-augmentation layer that accurately fuses predictions from the image and its horizontally flipped version, reducing the effect of left and right shadow regions generated in the disparity map due to occlusions. Both contributions provide significant performance gains over the state-of-the-art in self-supervised depth and pose estimation on the public KITTI benchmark. A video of our approach can be found at https://youtu.be/jKNgBeBMx0I.
更多查看译文
关键词
self-supervised monocular depth estimation,self-supervised monocular depth prediction,subpixel convolutional layer extension,depth super-resolution,high-resolution disparities,low-resolution convolutional features,flip-augmentation layer,single-image super-resolution,deep learning methods,super-resolved monocular depth estimation,public KITTI benchmark,pose estimation
AI 理解论文
溯源树
样例
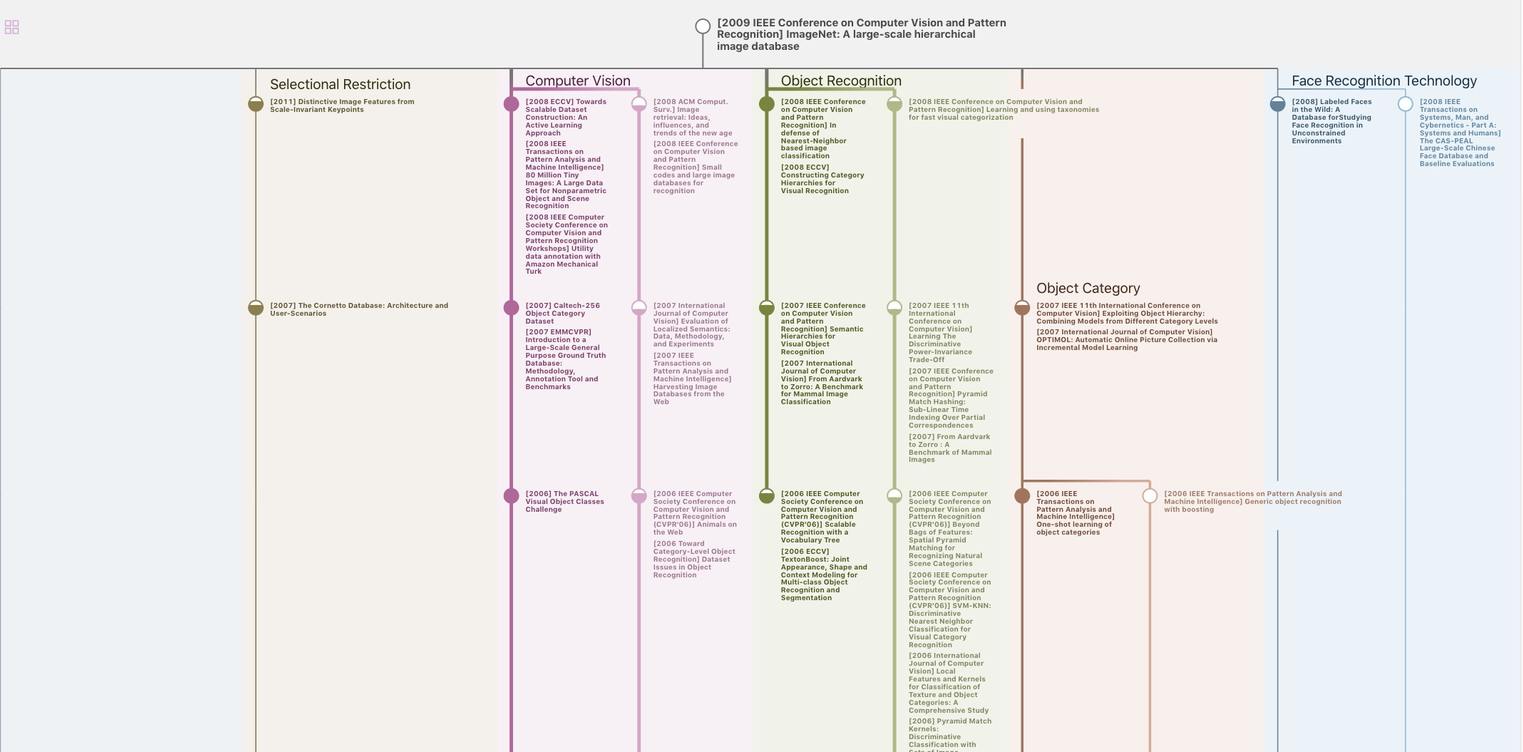
生成溯源树,研究论文发展脉络
Chat Paper
正在生成论文摘要