Predicting Mutations Deleterious To Function In Beta-Lactamase Tem1 Using Mm-Gbsa
PLOS ONE(2019)
摘要
Missense mutations can have disastrous effects on the function of a protein. And as a result, they have been implicated in numerous diseases. However, the majority of missense variants only have a nominal impact on protein function. Thus, the ability to distinguish these two classes of missense mutations would greatly aid drug discovery efforts in target identification and validation as well as medical diagnosis. Monitoring the co-occurrence of a given missense mutation and a disease phenotype provides a pathway for classifying functionally disrupting missense mutations. But, the occurrence of a specific missense variant is often extremely rare making statistical links challenging to infer. In this study, we benchmark a physics-based approach for predicting changes in stability, MMGBSA, and apply it to classifying mutations as functionally disrupting. A large and diverse dataset of 990 residue mutations in beta-lactamase TEM1 is used to assess performance as it is rich in both functionally disrupting mutations and functionally neutral/beneficial mutations. On this dataset, we compare the performance of MM-GBSA to alternative strategies for predicting functionally disrupting mutations. We observe that the MM-GBSA method obtains an area under the curve (AUC) of 0.75 on the entire dataset, outperforming all other predictors tested. More importantly, MM-GBSA's performance is robust to various divisions of the dataset, speaking to the generality of the approach. Though there is one notable exception: Mutations on the surface of the protein are the mutations that are the most difficult to classify as functionally disrupting for all methods tested. This is likely due to the many mechanisms available to surface mutations to disrupt function, and thus provides a direction of focus for future studies.
更多查看译文
AI 理解论文
溯源树
样例
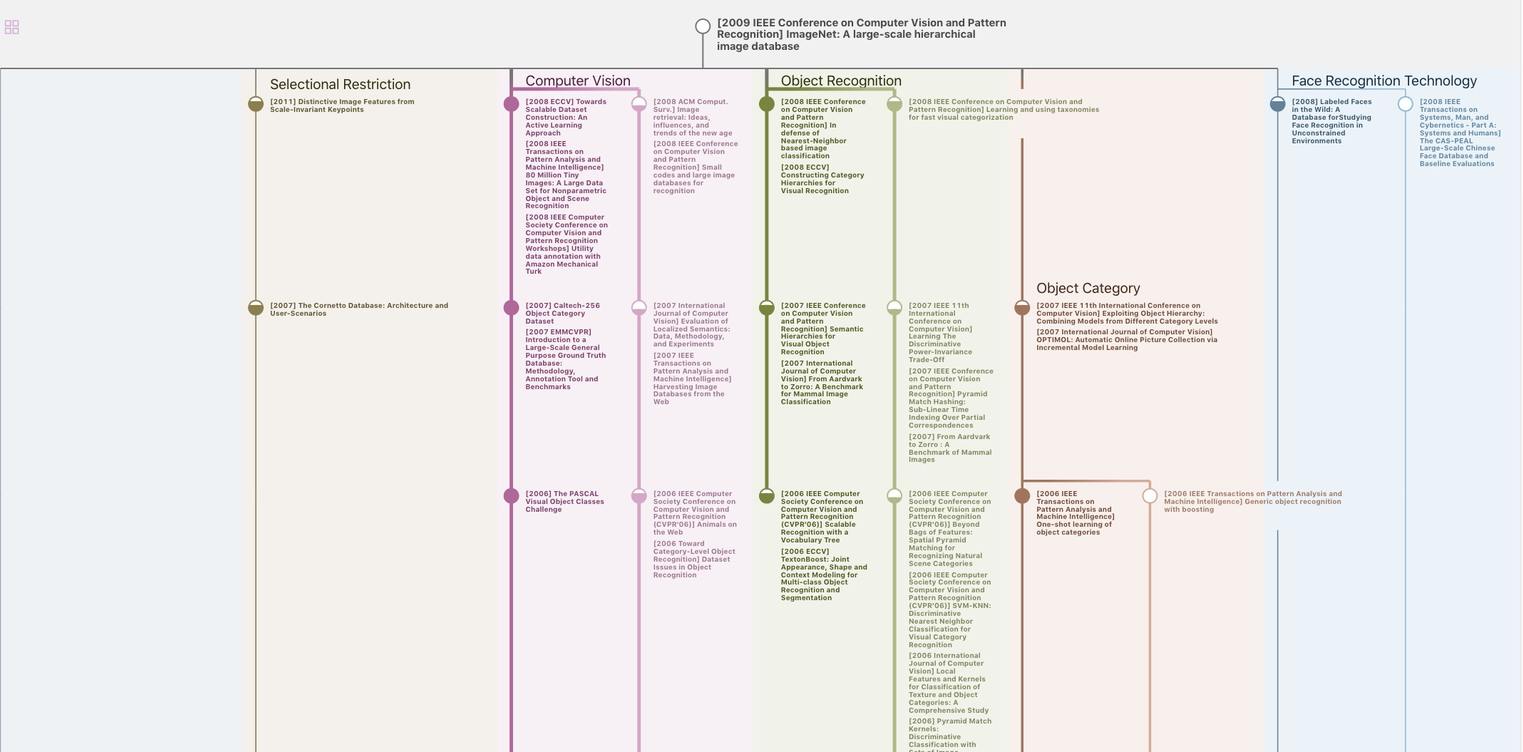
生成溯源树,研究论文发展脉络
Chat Paper
正在生成论文摘要