A Preliminary Study of Machine Learning Workload Prediction Techniques for Cloud Applications
2019 27th Euromicro International Conference on Parallel, Distributed and Network-Based Processing (PDP)(2019)
摘要
Cloud computing has transformed the means of computing in recent years with several benefits over traditional systems, like scalability and high availability. However, there are still some opportunities, especially in the area of resource provisioning and scaling [13]. Since workload may fluctuate a lot in certain environments, over-provisioning is a common practice to avoid abrupt Quality of Service (QoS) drops that may result in Service Level Agreement (SLA) violations, but at the price of an increase in provisioning costs and energy consumption. Workload prediction is one of the strategies by which efficiency and operational cost of a cloud can be improved [13]. Knowing demand in advance allows the previous allocation of sufficient resources to maintain QoS and avoid SLA violations [1]. This paper presents the advantages and disadvantages of three workload prediction techniques when applied in the context of cloud computing. Our preliminary results compare ARIMA, MLP, and GRU under different cloud configurations to help administrators choose the more appropriate and efficient predictive model for their specific problem.
更多查看译文
关键词
Cloud computing,Quality of service,Predictive models,Resource management,Machine learning,Scalability,Computational modeling
AI 理解论文
溯源树
样例
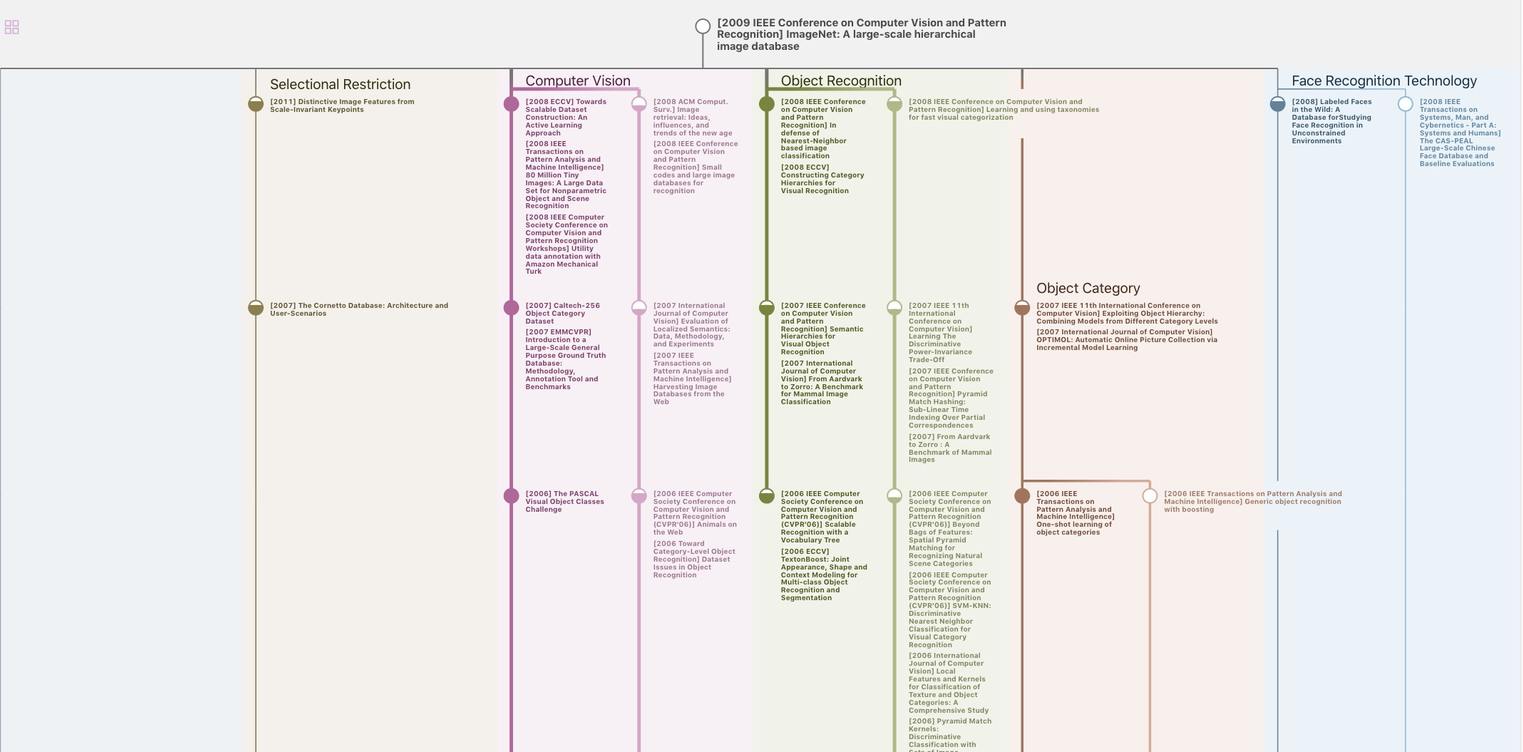
生成溯源树,研究论文发展脉络
Chat Paper
正在生成论文摘要