Image Denoising by Low-Rank Approximation with Estimation of Noise Energy Distribution in SVD Domain
IET image processing(2019)
摘要
Low-rank approximation has shown great potential in various image tasks. It is found that there is a specific functional relationship about singular values between the original image and a series of noisy images, which can be used to construct the singular values of a noise-free image. In this study, the authors propose a novel denoising method based on the above facts and low-rank approximation theory. Firstly, they estimate the noise energy distribution of the group matrix in the singular value decomposition (SVD) domain using the energy characteristics of the image with different noise levels. The energy distribution of the noise is shrunk to obtain the energy distribution of the true signal. Then, based on the optimal energy compaction property of SVD, the low-rank property of matrix is constrained in the SVD domain to obtain the low-rank approximation of the matrix. Moreover, an iterative back projection method is adopted in this study to suppress residual noise. A new noise standard deviation estimation approach, targeted at the back projection process, is proposed to effectively optimise the denoising results during the iteration. Experimental results show that the authors' method efficiently decreases the noise and achieves comparable denoising performance to the state-of-the-art methods regarding both quantitative measurement and visual effect.
更多查看译文
关键词
image denoising,approximation theory,signal denoising,singular value decomposition,wavelet transforms
AI 理解论文
溯源树
样例
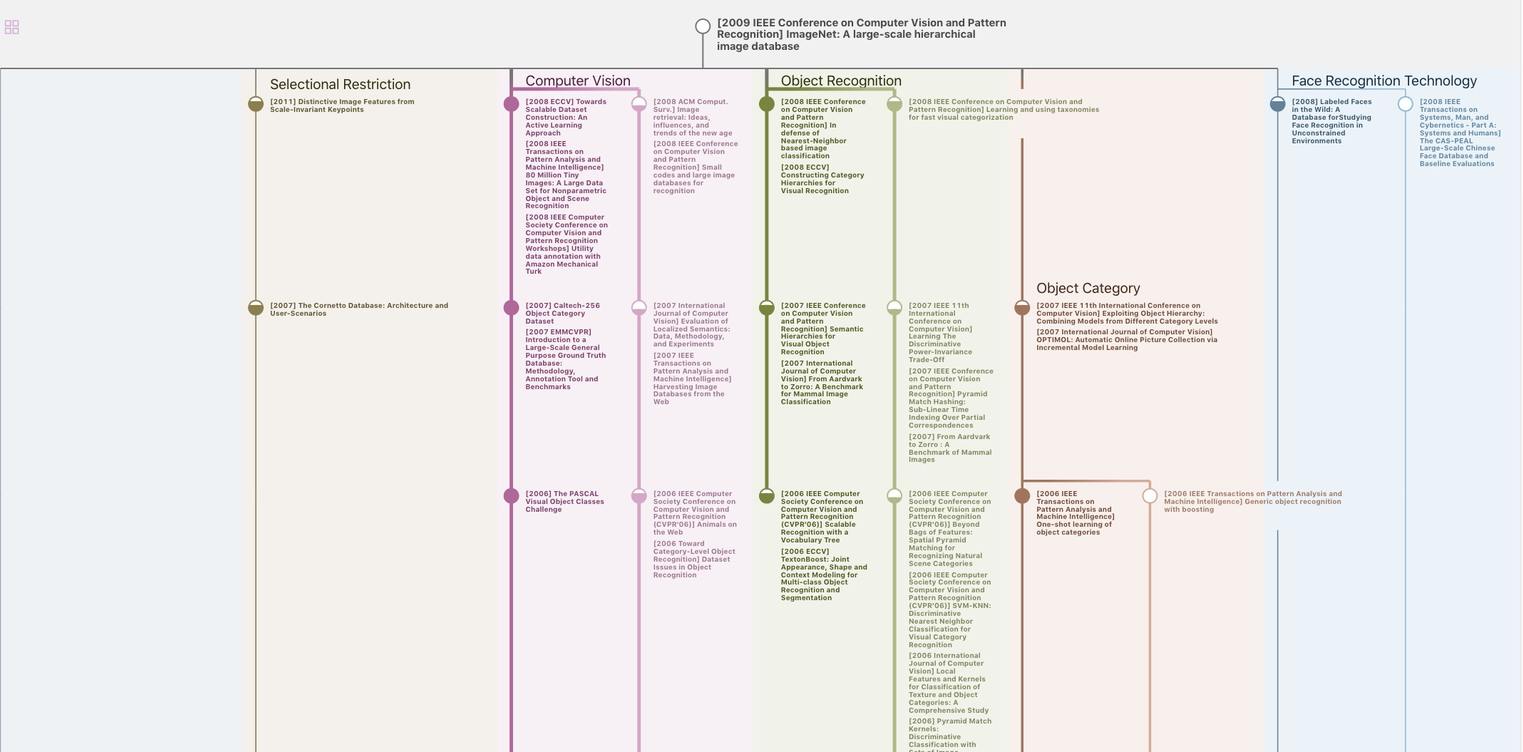
生成溯源树,研究论文发展脉络
Chat Paper
正在生成论文摘要