Illumination Invariant Skin Texture Generation Using CGAN from a Single Image for Haptic Augmented Palpation
2019 Third IEEE International Conference on Robotic Computing (IRC)(2019)
摘要
The problem of illumination normalization in the field of computer vision is a subject that has been studied steadily so far. However, the treatment of strong illumination has not been done to date. In this paper, we present a method of illumination normalization using a deep learning method, the conditional generative adversarial network (CGAN), to reconstruct accurate 3D skin textures from a single image toward efficient haptic palpation. After normalizing the illumination through the conditional generative adversarial network in which the input image is conditioned to obtain the desired output image, bilateral filtering that removes noise while preserving the boundaries enhances fine wrinkles. As the refinement process of enhancing skin textures, intrinsic image decomposition is performed to obtain a shading layer image with histogram equalization that is merged into the normalized image obtained by the illumination normalization process to enhance skin tactile properties (wrinkle and roughness). Through these processes, we can obtain a depth image with normalized illumination and enhanced skin wrinkle texture. Using this, the depth of the skin surface texture is restored in three dimensions precisely. The superiority of the illumination normalization method proposed in this paper over three other illumination normalization methods (CIDRE, LDCT, and TT) is verified through comparison. We also confirm the illumination normalization performance of our method through restoration of the three-dimensional skin surface.
更多查看译文
关键词
Skin,Lighting,Surface reconstruction,Haptic interfaces,Three-dimensional displays,Histograms,Image reconstruction
AI 理解论文
溯源树
样例
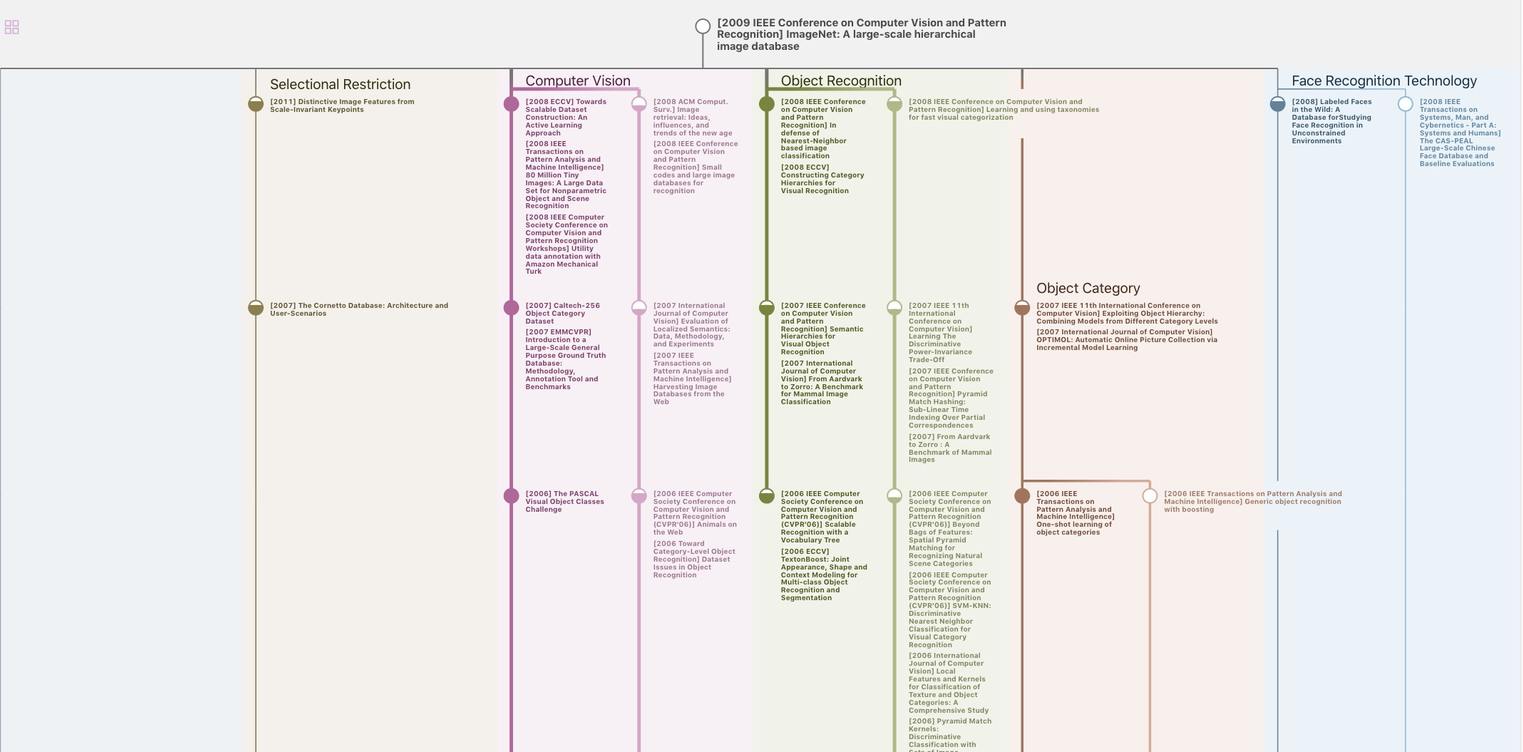
生成溯源树,研究论文发展脉络
Chat Paper
正在生成论文摘要