Automated remote fall detection using impact features from video and audio.
Journal of biomechanics(2019)
摘要
Elderly people and people with epilepsy may need assistance after falling, but may be unable to summon help due to injuries or impairment of consciousness. Several wearable fall detection devices have been developed, but these are not used by all people at risk. We present an automated analysis algorithm for remote detection of high impact falls, based on a physical model of a fall, aiming at universality and robustness. Candidate events are automatically detected and event features are used as classifier input. The algorithm uses vertical velocity and acceleration features from optical flow outputs, corrected for distance from the camera using moving object size estimation. A sound amplitude feature is used to increase detector specificity. We tested the performance and robustness of our trained algorithm using acted data from a public database and real life data with falls resulting from epilepsy and with daily life activities. Applying the trained algorithm to the acted dataset resulted in 90% sensitivity for detection of falls, with 92% specificity. In the real life data, six/nine falls were detected with a specificity of 99.7%; there is a plausible explanation for not detecting each of the falls missed. These results reflect the algorithm's robustness and confirms the feasibility of detecting falls using this algorithm.
更多查看译文
AI 理解论文
溯源树
样例
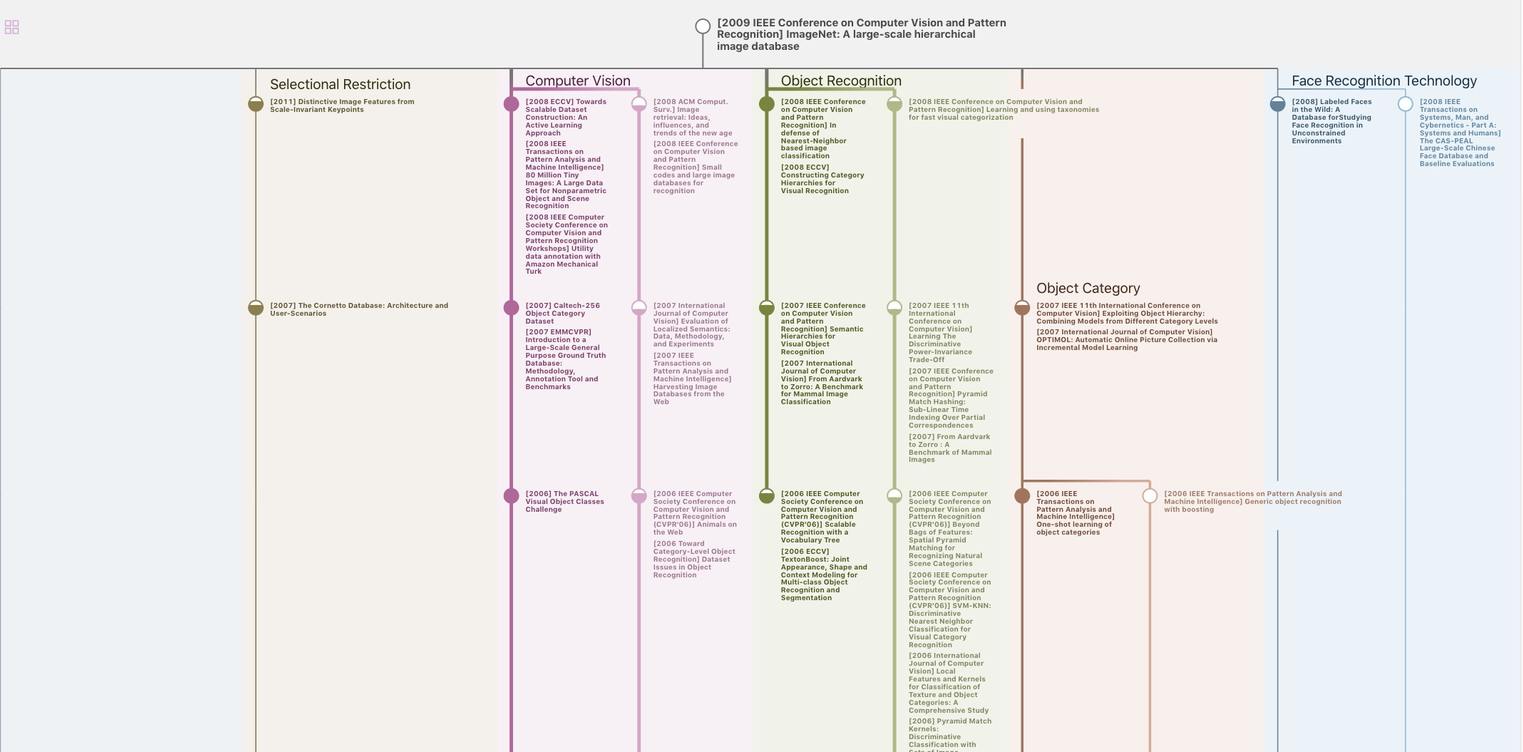
生成溯源树,研究论文发展脉络
Chat Paper
正在生成论文摘要