Privacy-preserving Cross-domain Location Recommendation
Proceedings of the ACM on Interactive, Mobile, Wearable and Ubiquitous Technologies(2019)
摘要
Cross-domain recommendation is a typical solution for data sparsity and cold start issue in the field of location recommendation. Specifically, data of an auxiliary domain is leveraged to improve the recommendation of the target domain. There is a typical scenario that two interaction domains (location based check-in service, for example) combine data to perform the cross-domain location recommendation task. Existing approaches are based on the assumption that the interaction data from the auxiliary domain can be directly shared across domains. However, such an assumption is not reasonable, since in the real world those domains may be operated by different companies. Therefore, directly sharing raw data may violate business privacy policy and increase the risk of privacy leakage since the user-location interaction records are very sensitive.
In this paper, we propose a framework named privacy-preserving cross-domain location recommendation which works in two stages. First, for the interaction data from the auxiliary domain, we adopt a differential privacy based protection mechanism to hide the real locations of each user to meet the criterion of differential privacy. Then we share the protected user-location interaction to the target domain. Second, we develop a new method of Confidence-aware Collective Matrix Factorization (CCMF) to effectively exploit the transferred interaction data. To verify its efficacy, we collect two real-world datasets suitable for the task. Extensive experiments demonstrate that our proposed framework achieves the best performance compared with the state-of-the-art baseline methods. We further demonstrate that our method can alleviate the data sparsity issue significantly while protecting users' location privacy.
更多查看译文
关键词
Cross-domain Location Recommendation, Differential Privacy, Matrix Factorization
AI 理解论文
溯源树
样例
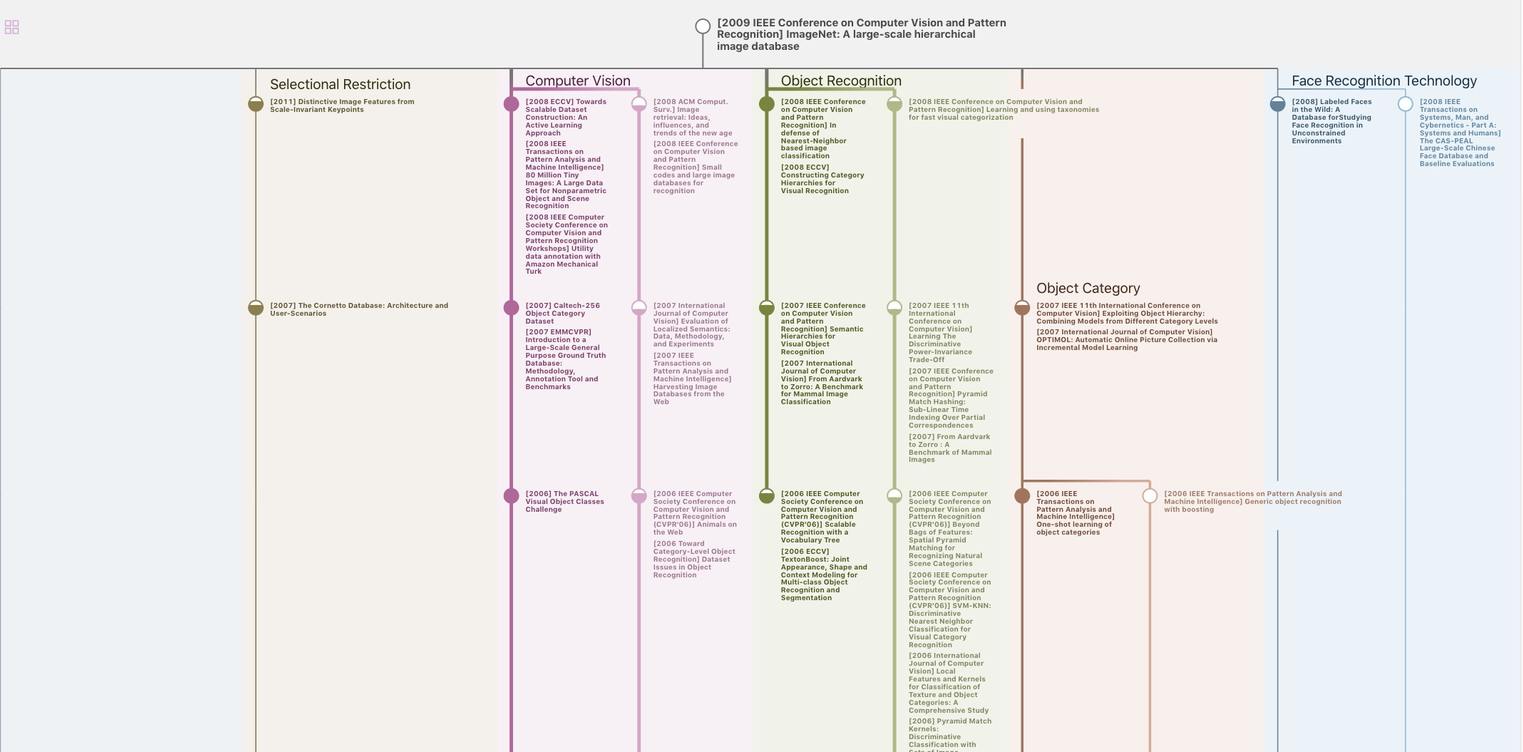
生成溯源树,研究论文发展脉络
Chat Paper
正在生成论文摘要