Modeling Spatio-Temporal App Usage for a Large User Population
Proceedings of the ACM on Interactive, Mobile, Wearable and Ubiquitous Technologies(2019)
摘要
With the wide adoption of mobile devices, it becomes increasingly important to understand how users use mobile apps. Knowing when and where certain apps are used is instrumental for app developers to improve app usability and for Internet service providers (ISPs) to optimize their network services. However, modeling spatio-temporal patterns of app usage has been a challenging problem due to the complicated usage behavior and the very limited personal data. In this paper, we propose a Bayesian mixture model to capture when, where and what apps are used and predict future app usage. To solve the challenge of data sparsity, we apply a hierarchical Dirichlet process to leverage the shared spatio-temporal patterns to accurately model users with insufficient data. We then evaluate our model using a large dataset of app usage traces involving 1.7 million users over 3503 apps. Our analysis shows a clear correlation between the user's location and the apps being used. Extensive evaluations show that our model can accurately predict users' future locations and app usage, outperforming the state-of-the-art algorithms by 11.7% and 11.1%, respectively. In addition, our model can be used to synthesize app usage traces that do not leak user privacy while preserving the key data statistical properties.
更多查看译文
关键词
Bayesian mixture model, app usage, spatio-temporal pattern
AI 理解论文
溯源树
样例
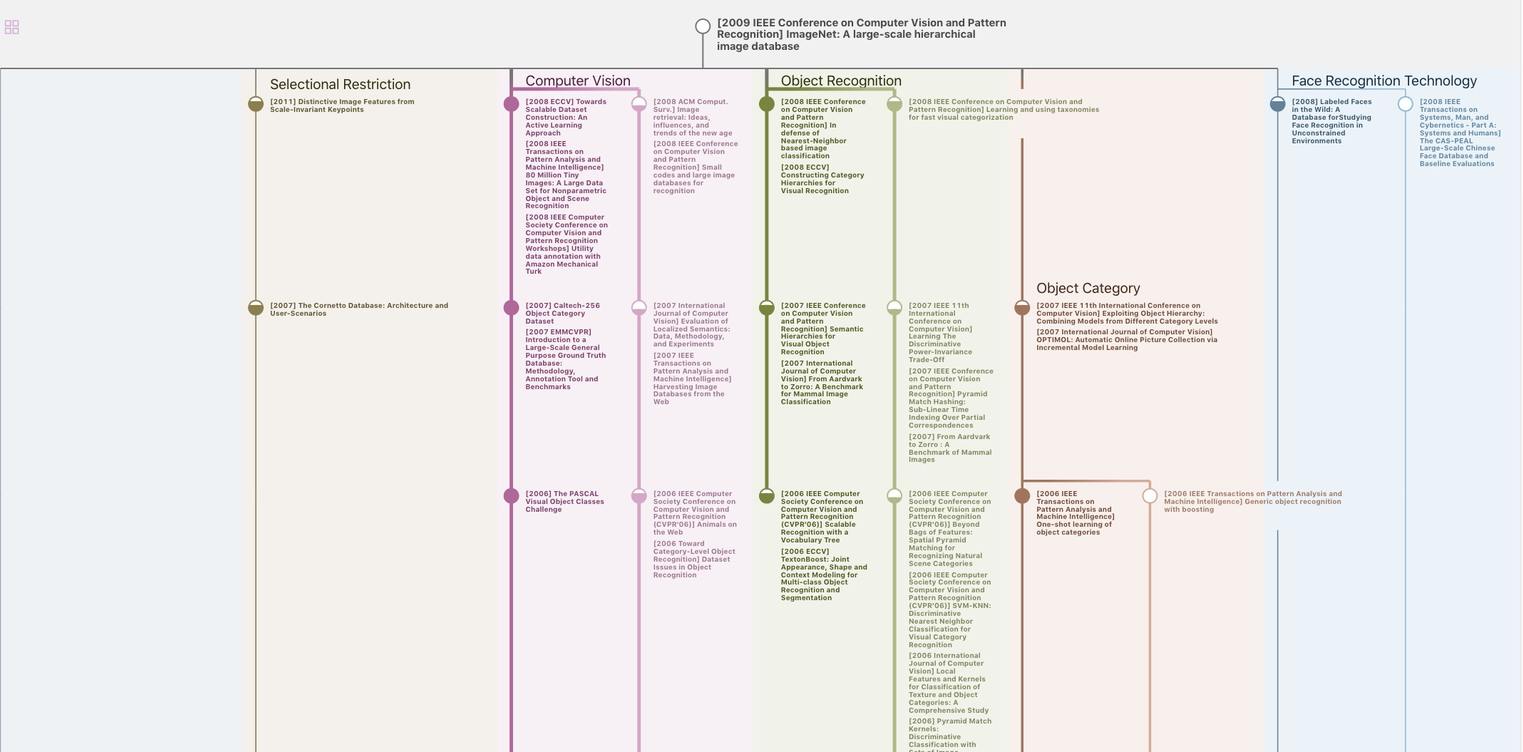
生成溯源树,研究论文发展脉络
Chat Paper
正在生成论文摘要