VINE: Visualizing Statistical Interactions in Black Box Models.
CoRR(2019)
摘要
As machine learning becomes more pervasive, there is an urgent need for interpretable explanations of predictive models. Prior work has developed effective methods for visualizing global model behavior, as well as generating local (instance-specific) explanations. However, relatively little work has addressed regional explanations - how groups of similar instances behave in a complex model, and the related issue of visualizing statistical feature interactions. The lack of utilities available for these analytical needs hinders the development of models that are mission-critical, transparent, and align with social goals. We present VINE (Visual INteraction Effects), a novel algorithm to extract and visualize statistical interaction effects in black box models. We also present a novel evaluation metric for visualizations in the interpretable ML space.
更多查看译文
关键词
Interactive Visualization,Information Visualization,Interpretable Models,Visual Analytics,Visual Explanations
AI 理解论文
溯源树
样例
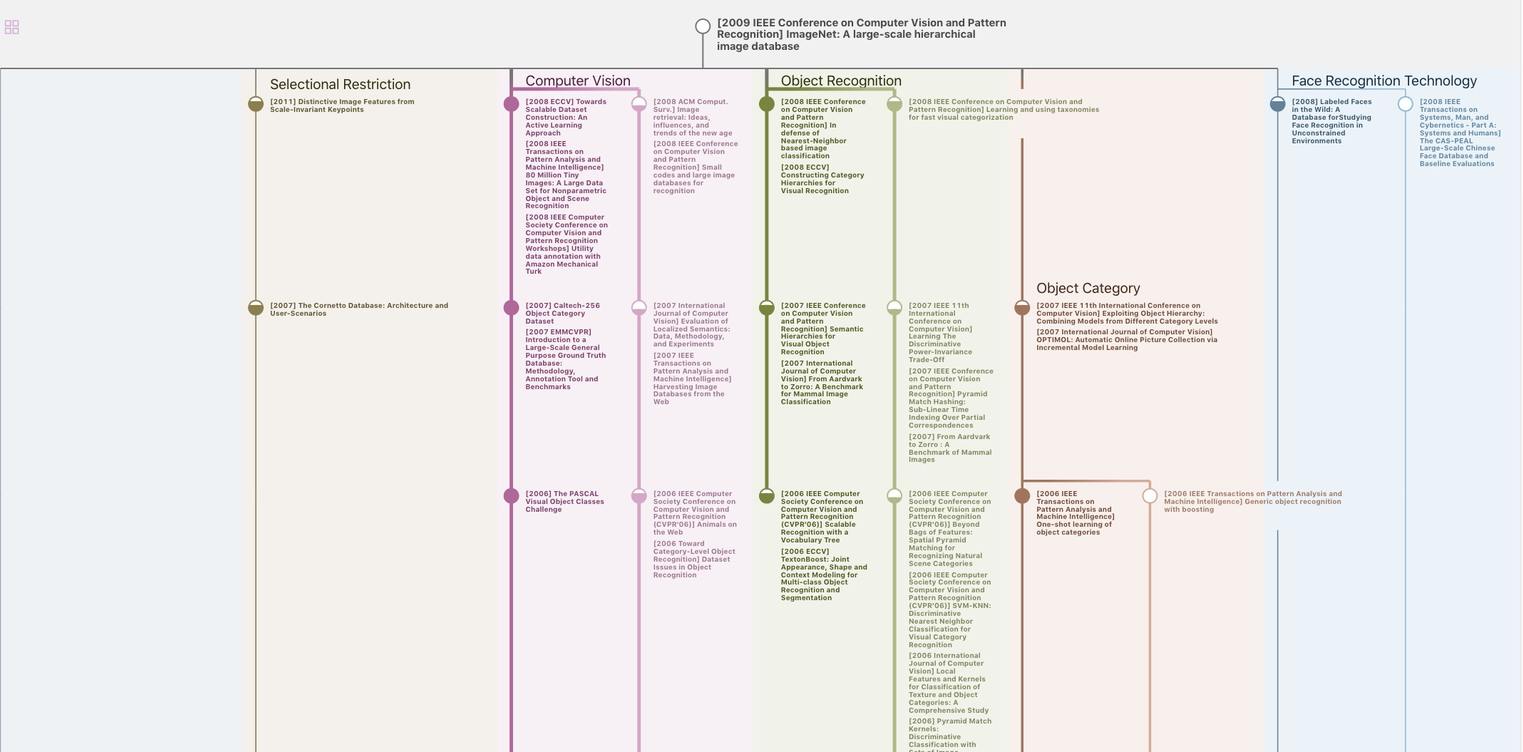
生成溯源树,研究论文发展脉络
Chat Paper
正在生成论文摘要