An Analysis of Erlangen University Hospital's Billing Data on Utility-Based De-Identification.
PubMed(2019)
摘要
Background: To make patient care data more accessible for research, German university hospitals join forces in the course of the Medical Informatics Initiative. In a first step, the administrative data of university hospitals is made available for federated utilization. Project-specific de-identification of this data is necessary to satisfy privacy laws. Objective: We want to make a statement about the population uniqueness of the data. By generalizing the data, we try to reduce uniqueness and improve k-anonymity. Methods: We analyze quasi-identifying attributes of the Erlangen University Hospital's billing data regarding population uniqueness and re-identification risk. We count individuals per equality class (k) to measure uniqueness. Results: Because of the diagnoses and procedures being particularly unique in combination with sex and age of the patients, the data set is not anonymized in matters of k-anonymity with k > 1. We are able to reduce population uniqueness with generalization and suppression of unique domains. Conclusion: To create k-anonymity with k > 1 while still maintaining a particular utility of the data, we need to apply further established strategies of de-identification.
更多查看译文
关键词
Data privacy,k-anonymity,risk,secondary use,population uniqueness
AI 理解论文
溯源树
样例
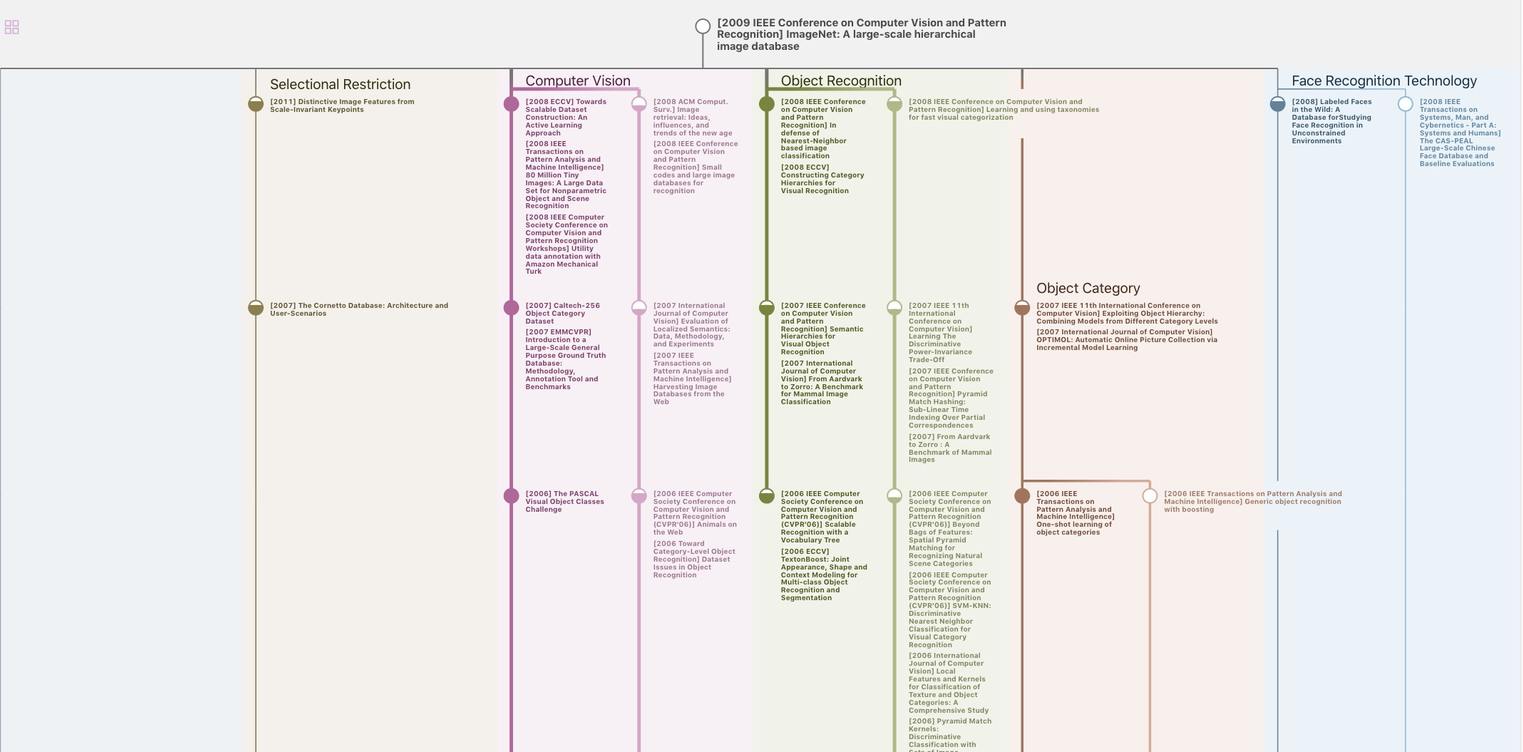
生成溯源树,研究论文发展脉络
Chat Paper
正在生成论文摘要