Exploiting Symmetries in Reinforcement Learning of Bimanual Robotic Tasks
IEEE ROBOTICS AND AUTOMATION LETTERS(2019)
摘要
Movement primitives (MPs) have been widely adopted for representing and learning robotic movements using reinforcement learning policy search. Probabilistic movement primitives (ProMPs) are a kind of MP based on a stochastic representation over sets of trajectories, able to capture the variability allowed while executing a movement. This approach has proved effective in learning a wide range of robotic movements, but it comes with the necessity of dealing with a high-dimensional space of parameters. This may be a critical problem when learning tasks with two robotic manipulators, and this work proposes an approach to reduce the dimension of the parameter space based on the exploitation of symmetry. A symmetrization method for ProMPs is presented and used to represent two movements, employing a single ProMP for the first arm and a symmetry surface that maps that ProMP to the second arm. This symmetric representation is then adopted in reinforcement learning of bimanual tasks (from user-provided demonstrations), using relative entropy policy search algorithm. The symmetry-based approach developed has been tested in an experiment of cloth manipulation, showing a speed increment in learning the task.
更多查看译文
关键词
Learning and Adaptive systems, learning from demonstration, dual arm manipulation
AI 理解论文
溯源树
样例
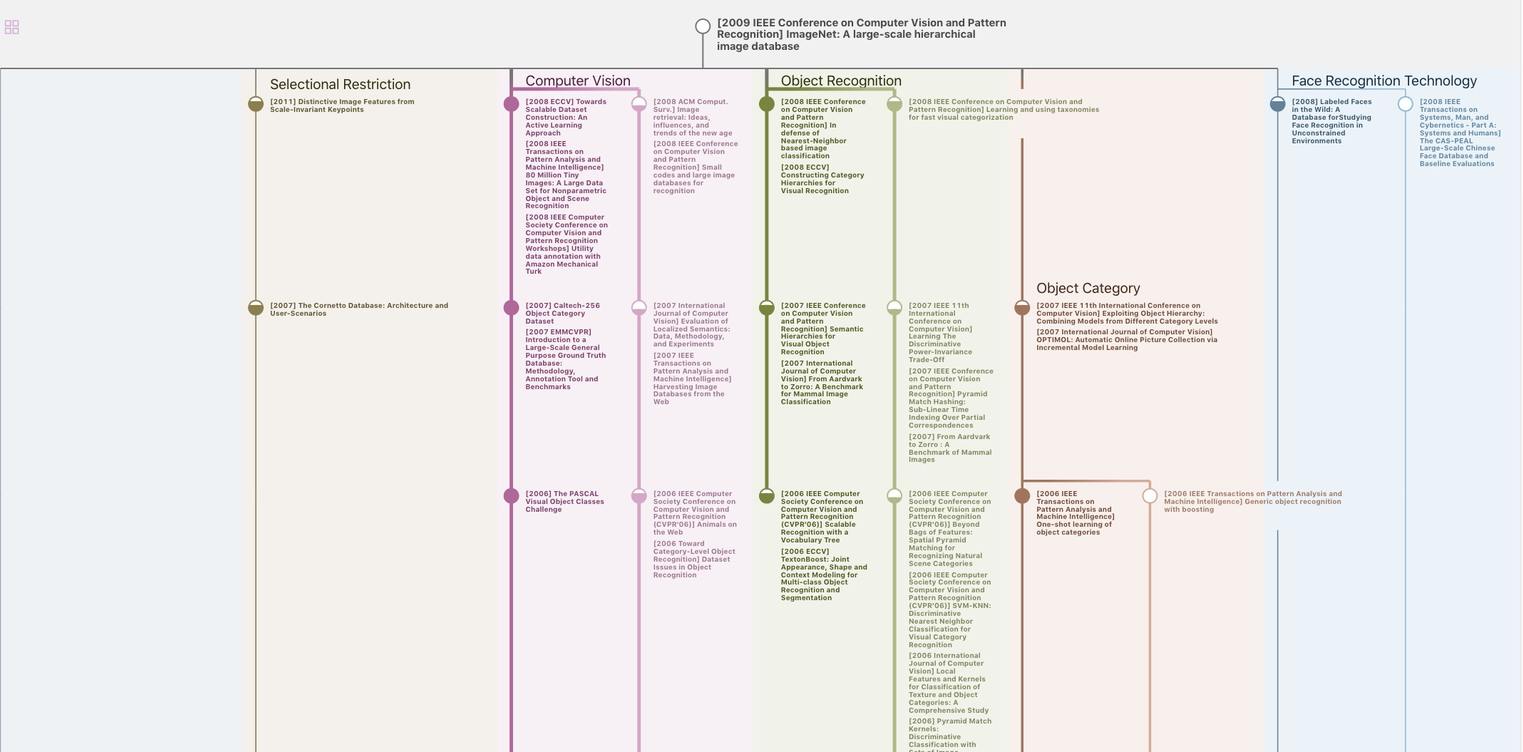
生成溯源树,研究论文发展脉络
Chat Paper
正在生成论文摘要