Batched Multi-armed Bandits Problem.
ADVANCES IN NEURAL INFORMATION PROCESSING SYSTEMS 32 (NIPS 2019)(2019)
摘要
In this paper, we study the multi-armed bandit problem in the batched setting where the employed policy must split data into a small number of batches. While the minimax regret for the two-armed stochastic bandits has been completely characterized in [PRCS16], the effect of the number of arms on the regret for the multi-armed case is still open. Moreover, the question whether adaptively chosen batch sizes will help to reduce the regret also remains underexplored. In this paper, we propose the BaSE (batched successive elimination) policy to achieve the rate-optimal regrets (within logarithmic factors) for batched multi-armed bandits, with matching lower bounds even if the batch sizes are determined in an adaptive manner.
更多查看译文
关键词
minimax regret
AI 理解论文
溯源树
样例
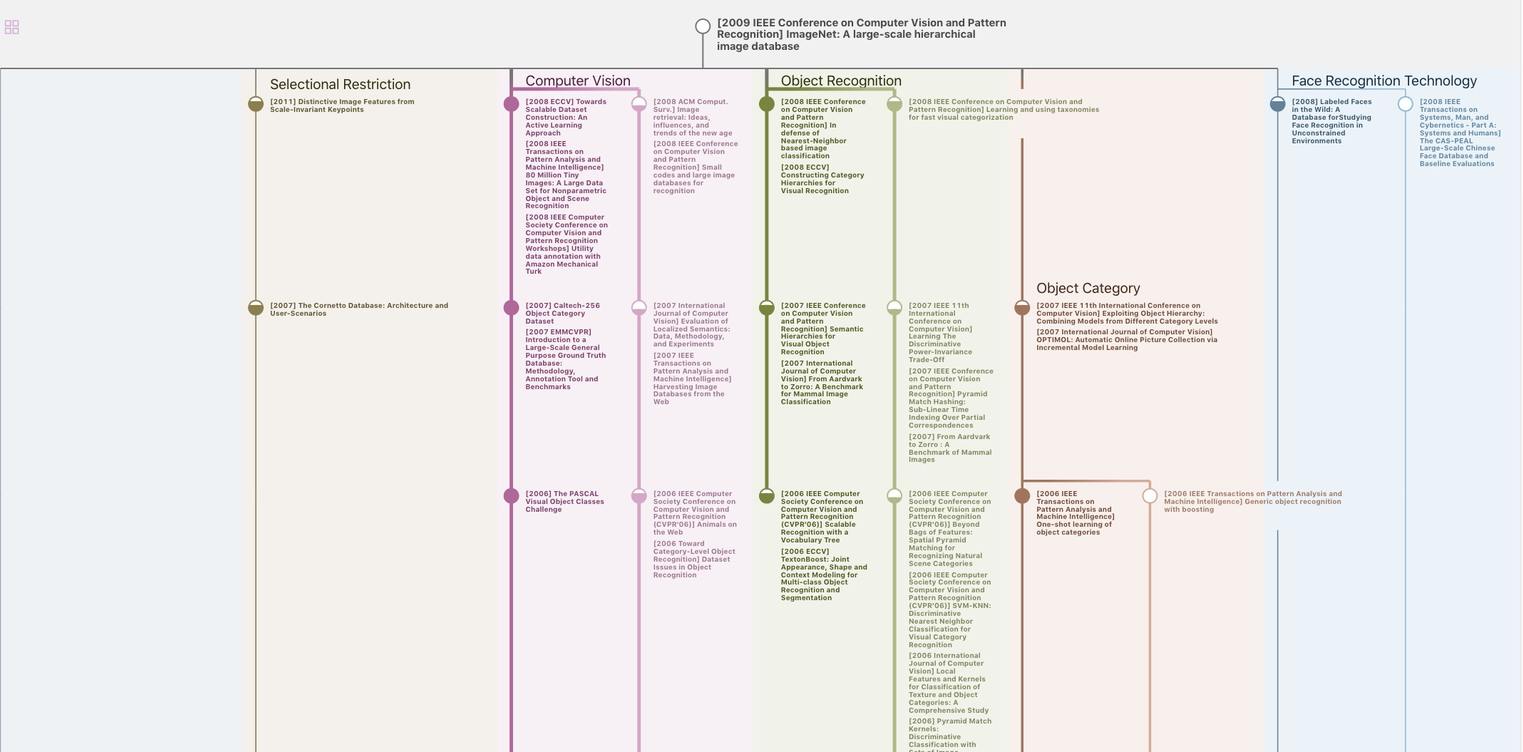
生成溯源树,研究论文发展脉络
Chat Paper
正在生成论文摘要