Resisting Large Data Variations via Introspective Transformation Network
2020 IEEE WINTER CONFERENCE ON APPLICATIONS OF COMPUTER VISION (WACV)(2020)
摘要
Training deep networks that generalize to a wide range of variations in test data is essential to building accurate and robust image classifiers. Data variations in this paper include but not limited to unseen affine transformations and warping in the training data. One standard strategy to overcome this problem is to apply data augmentation to synthetically enlarge the training set. However, data augmentation is essentially a brute-force method which generates uniform samples from some pre-defined set of transformations. In this paper, we propose a principled approach named introspective transformation network (ITN) that significantly improves network resistance to large variations between training and testing data. This is achieved by embedding a learnable transformation module into the introspective network, which is a convolutional neural network (CNN) classifier empowered with generative capabilities. Our approach alternates between synthesizing pseudo-negative samples and transformed positive examples based on the current model, and optimizing model predictions on these synthesized samples. Experimental results verify that our approach significantly improves the ability of deep networks to resist large variations between training and testing data and achieves classification accuracy improvements on several benchmark datasets, including MNIST, affNIST, SVHN, CIFAR-10 and miniImageNet.
更多查看译文
关键词
introspective transformation network,learnable transformation module,convolutional neural network classifier,deep network training,data variations,image classifiers,affine transformations,data augmentation
AI 理解论文
溯源树
样例
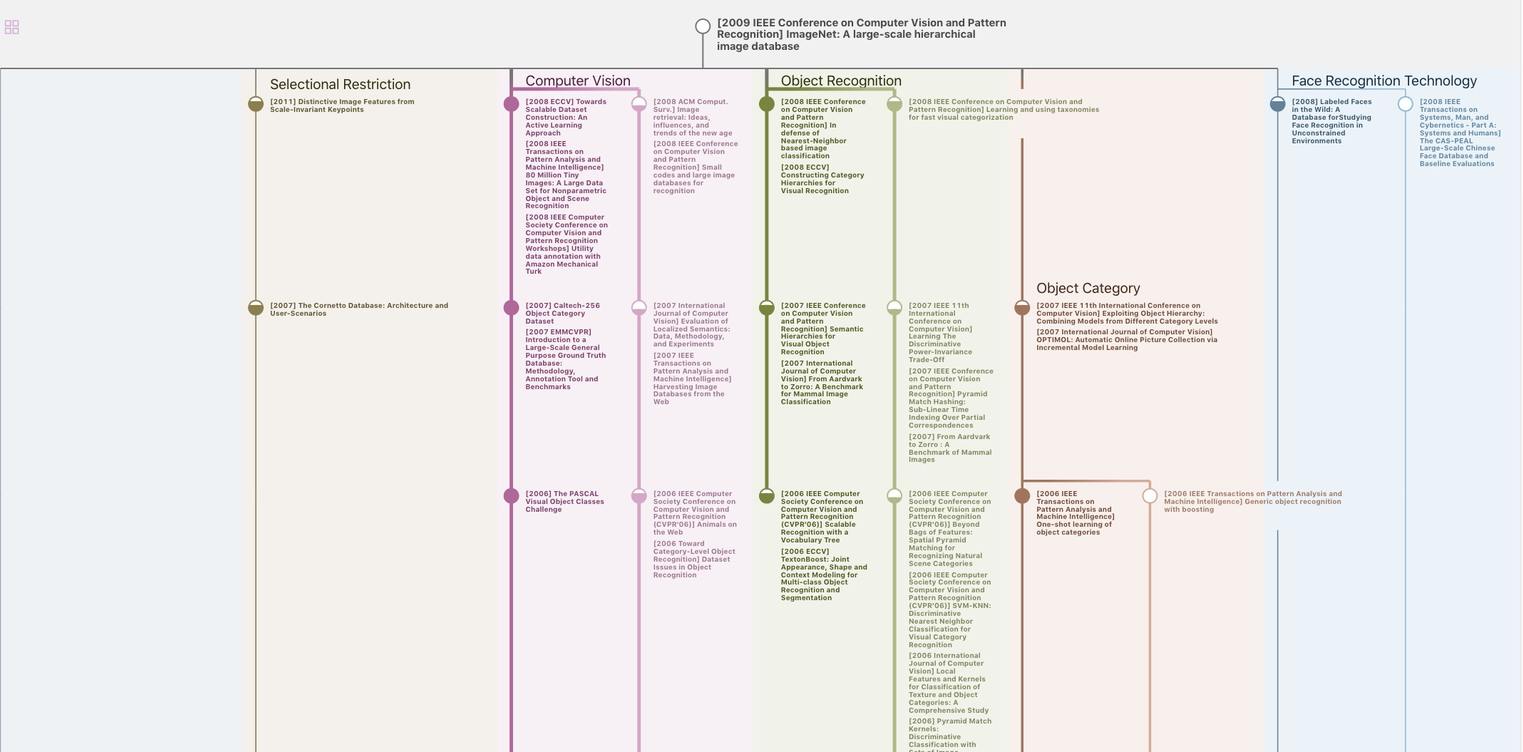
生成溯源树,研究论文发展脉络
Chat Paper
正在生成论文摘要