Pixels To Plans: Learning Non-Prehensile Manipulation By Imitating A Planner
2019 IEEE/RSJ INTERNATIONAL CONFERENCE ON INTELLIGENT ROBOTS AND SYSTEMS (IROS)(2019)
摘要
We present a novel method enabling robots to quickly learn to manipulate objects by leveraging a motion planner to generate "expert" training trajectories from a small amount of human-labeled data. In contrast to the traditional sense-plan-act cycle, we propose a deep learning architecture and training regimen called PtPNet that can estimate effective end-effector trajectories for manipulation directly from a single RGB-D image of an object. Additionally, we present a data collection and augmentation pipeline that enables the automatic generation of large numbers (millions) of training image and trajectory examples with almost no human labeling effort.We demonstrate our approach in a non-prehensile tool-based manipulation task, specifically picking up shoes with a hook. In hardware experiments, PtPNet generates motion plans (open-loop trajectories) that reliably (89% success over 189 trials) pick up four very different shoes from a range of positions and orientations, and reliably picks up a shoe it has never seen before. Compared with a traditional sense-plan-act paradigm, our system has the advantages of operating on sparse information (single RGB-D frame), producing high-quality trajectories much faster than the expert planner (300ms versus several seconds), and generalizing effectively to previously unseen shoes.
更多查看译文
关键词
nonprehensile manipulation learning,motion planner,expert training trajectories,deep learning architecture,PtPNet,end-effector trajectories,RGB-D image,data collection,nonprehensile tool-based manipulation task,open-loop trajectories,expert planner,robot learning,data augmentation
AI 理解论文
溯源树
样例
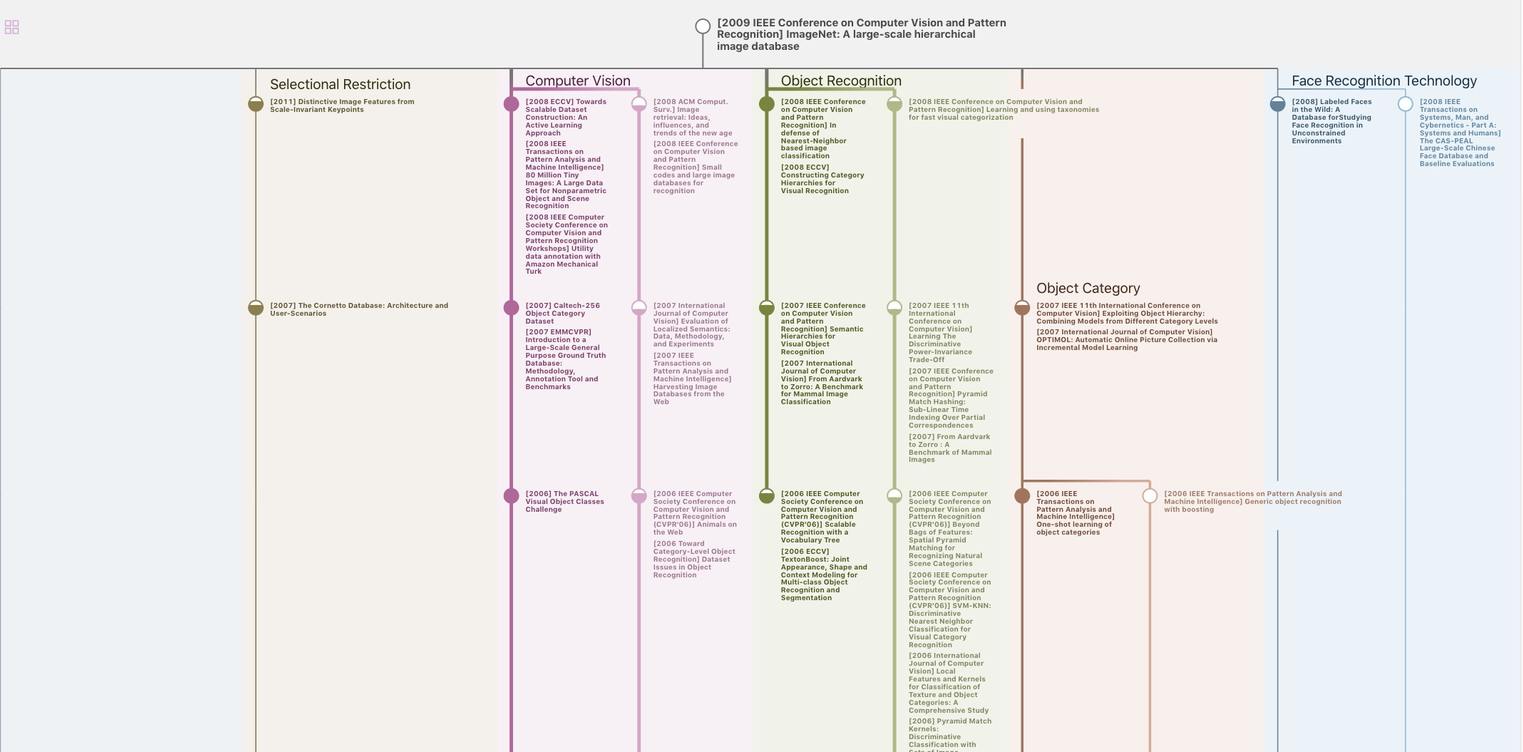
生成溯源树,研究论文发展脉络
Chat Paper
正在生成论文摘要