Asap: Architecture Search, Anneal And Prune
INTERNATIONAL CONFERENCE ON ARTIFICIAL INTELLIGENCE AND STATISTICS, VOL 108(2020)
摘要
Automatic methods for Neural Architecture Search (NAS) have been shown to produce state-of-the-art network models. Yet, their main drawback is the computational complexity of the search process. As some primal methods optimized over a discrete search space, thousands of days of GPU were required for convergence. A recent approach is based on constructing a differentiable search space that enables gradient-based optimization, which reduces the search time to a few days. While successful, it still includes some noncontinuous steps, e.g., the pruning of many weak connections at once. In this paper, we propose a differentiable search space that allows the annealing of architecture weights, while gradually pruning inferior operations. In this way, the search converges to a single output network in a continuous manner. Experiments on several vision datasets demonstrate the effectiveness of our method with respect to the search cost and accuracy of the achieved model. Specifically, with 0.2 GPU search days we achieve an error rate of 1.68% on CIFAR-10.
更多查看译文
AI 理解论文
溯源树
样例
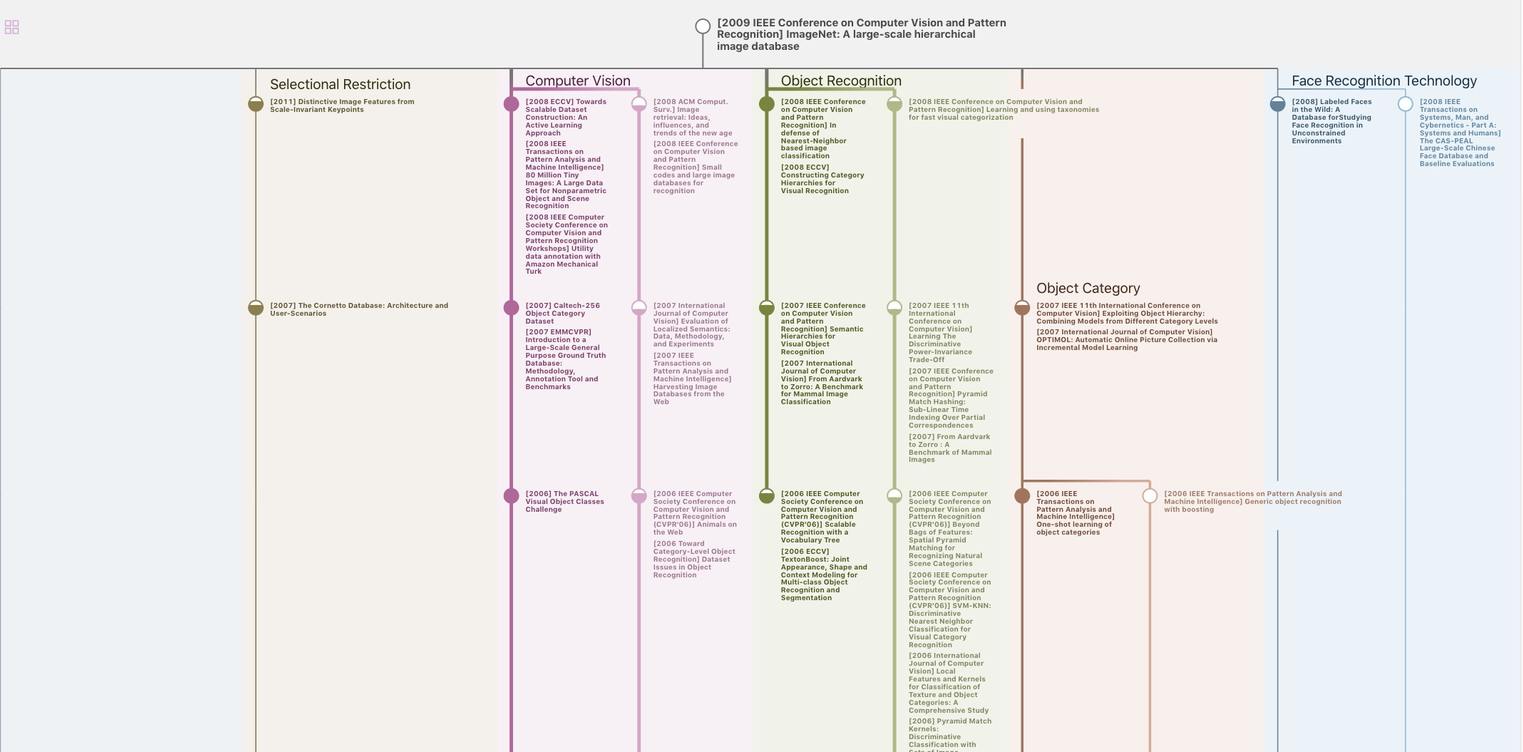
生成溯源树,研究论文发展脉络
Chat Paper
正在生成论文摘要