Electromyography-Based Respiratory Onset Detection in COPD Patients on Non-Invasive Mechanical Ventilation.
ENTROPY(2019)
摘要
To optimize long-term nocturnal non-invasive ventilation in patients with chronic obstructive pulmonary disease, surface diaphragm electromyography (EMGdi) might be helpful to detect patient-ventilator asynchrony. However, visual analysis is labor-intensive and EMGdi is heavily corrupted by electrocardiographic (ECG) activity. Therefore, we developed an automatic method to detect inspiratory onset from EMGdi envelope using fixed sample entropy (fSE) and a dynamic threshold based on kernel density estimation (KDE). Moreover, we combined fSE with adaptive filtering techniques to reduce ECG interference and improve onset detection. The performance of EMGdi envelopes extracted by applying fSE and fSE with adaptive filtering was compared to the root mean square (RMS)-based envelope provided by the EMG acquisition device. Automatic onset detection accuracy, using these three envelopes, was evaluated through the root mean square error (RMSE) between the automatic and mean visual onsets (made by two observers). The fSE-based method provided lower RMSE, which was reduced from 298 ms to 264 ms when combined with adaptive filtering, compared to 301 ms provided by the RMS-based method. The RMSE was negatively correlated with the proposed EMGdi quality indices. Following further validation, fSE with KDE, combined with adaptive filtering when dealing with low quality EMGdi, indicates promise for detecting the neural onset of respiratory drive.
更多查看译文
关键词
fixed sample entropy,adaptive filtering,root mean square,diaphragm electromyography,non-invasive mechanical ventilation,chronic obstructive pulmonary disease
AI 理解论文
溯源树
样例
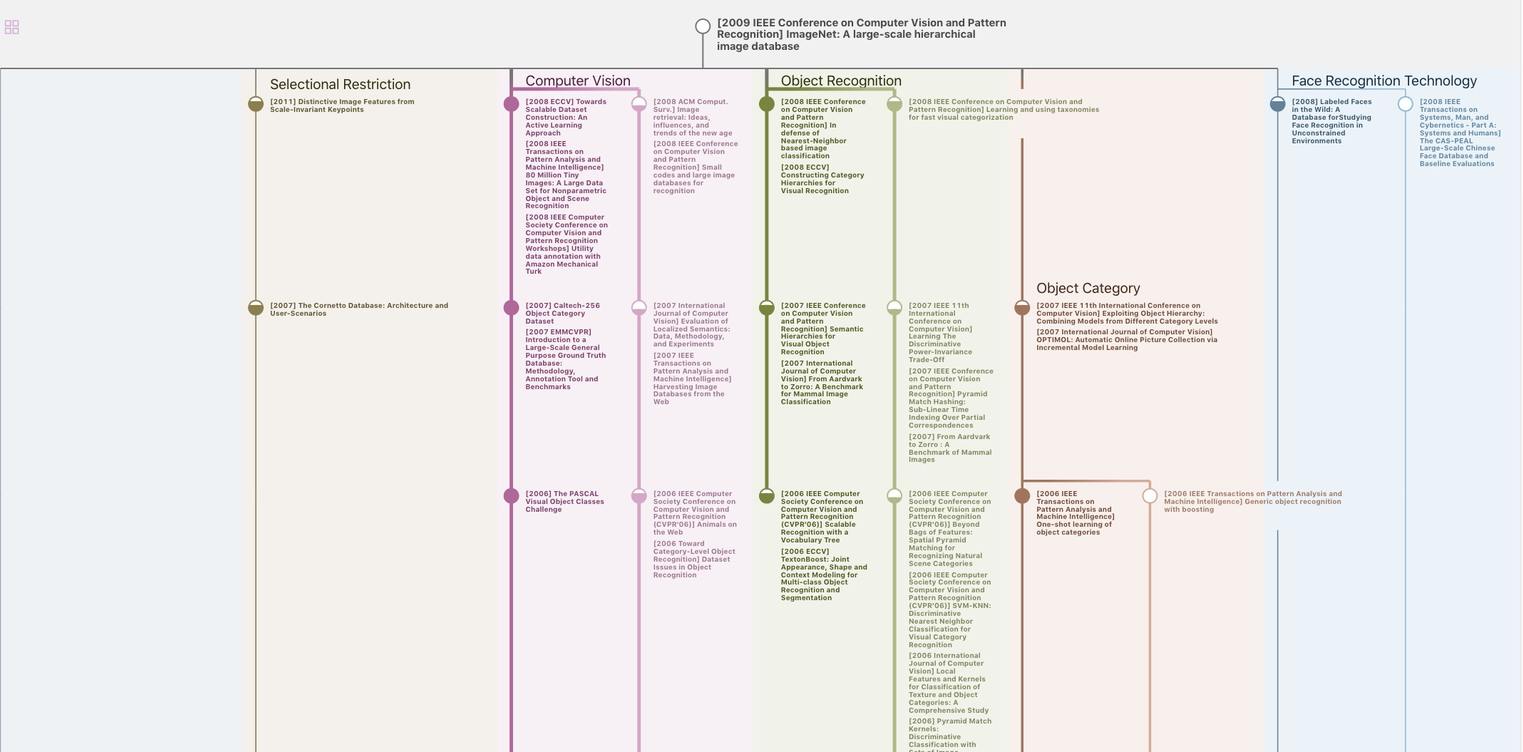
生成溯源树,研究论文发展脉络
Chat Paper
正在生成论文摘要