Extreme Multiclass Classification Criteria
COMPUTATION(2019)
摘要
We analyze the theoretical properties of the recently proposed objective function for efficient online construction and training of multiclass classification trees in the settings where the label space is very large. We show the important properties of this objective and provide a complete proof that maximizing it simultaneously encourages balanced trees and improves the purity of the class distributions at subsequent levels in the tree. We further explore its connection to the three well-known entropy-based decision tree criteria, i.e., Shannon entropy, Gini-entropy and its modified variant, for which efficient optimization strategies are largely unknown in the extreme multiclass setting. We show theoretically that this objective can be viewed as a surrogate function for all of these entropy criteria and that maximizing it indirectly optimizes them as well. We derive boosting guarantees and obtain a closed-form expression for the number of iterations needed to reduce the considered entropy criteria below an arbitrary threshold. The obtained theorem relies on a weak hypothesis assumption that directly depends on the considered objective function. Finally, we prove that optimizing the objective directly reduces the multi-class classification error of the decision tree.
更多查看译文
关键词
multiclass classification, decision trees, boosting
AI 理解论文
溯源树
样例
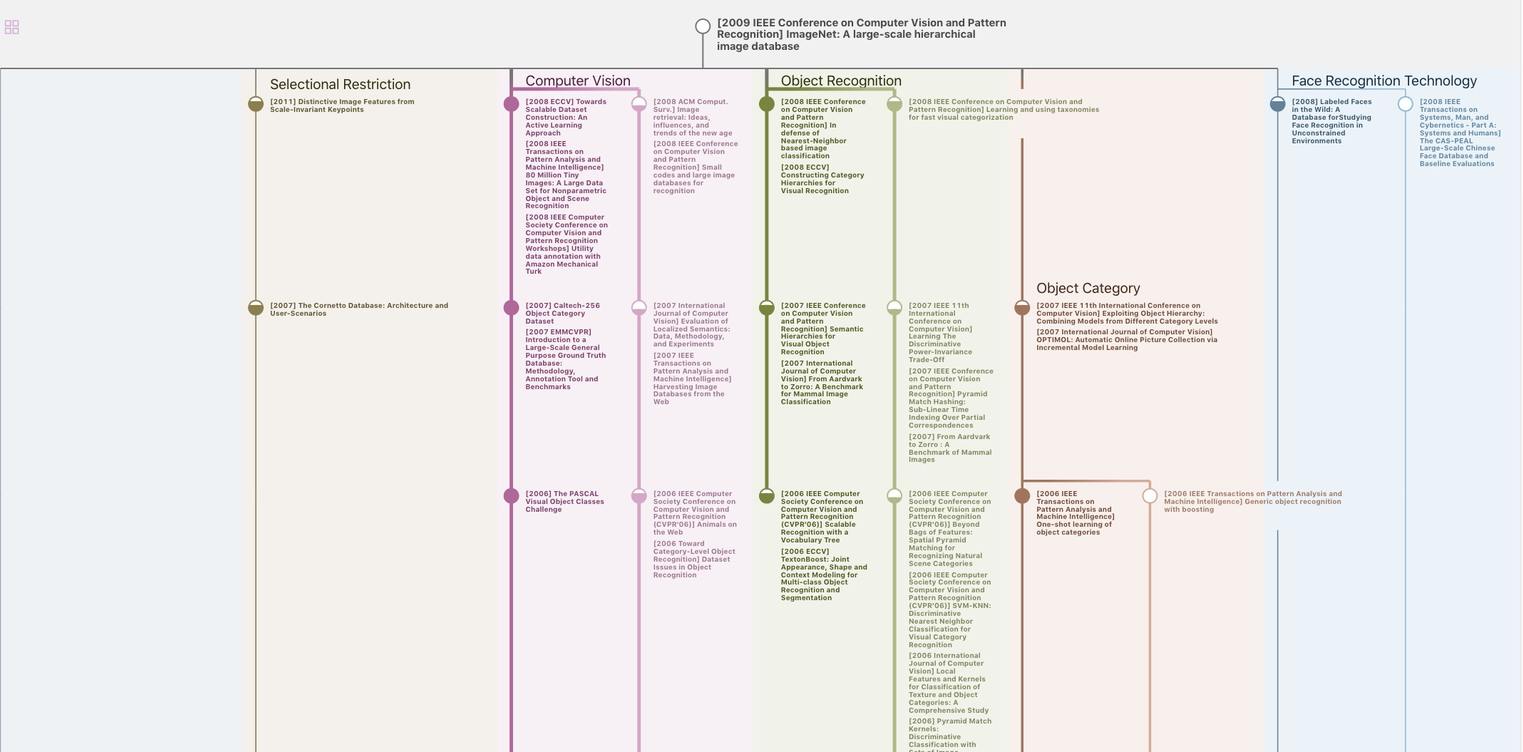
生成溯源树,研究论文发展脉络
Chat Paper
正在生成论文摘要