IoT-based Urban Noise Identification Using Machine Learning - Performance of SVM, KNN, Bagging, and Random Forest.
COINS(2019)
摘要
Noise is any undesired environmental sound. A sound at the same dB level may be perceived as annoying noise or as pleasant music. Therefore, it is necessary to go beyond the state-of-the-art approaches that measure only the dB level and also identify the type of noise. In this paper, we present a machine learning based method for urban noise identification using an inexpensive IoT unit. We use Mel-frequency cepstral coefficients for audio feature extraction and supervised classification algorithms (that is, support vector machine, k-nearest neighbors, bootstrap aggregation, and random forest) for noise classification. We evaluate our approach experimentally with a data-set of about 3000 sound samples grouped in eight sound classes (such as car horn, jackhammer, or street music). We explore the parameter space of the four algorithms to estimate the optimal parameter values for classification of sound samples in the data-set under study. We achieve a noise classification accuracy in the range 88% - 94%.
更多查看译文
关键词
bootstrap aggregation (Bagging), internet of things (IoT), k-nearest neighbors (KNN), mel-frequency cepstral coefficients (MFCC), random forest, smart cities, support vector machine (SVM), urban noise
AI 理解论文
溯源树
样例
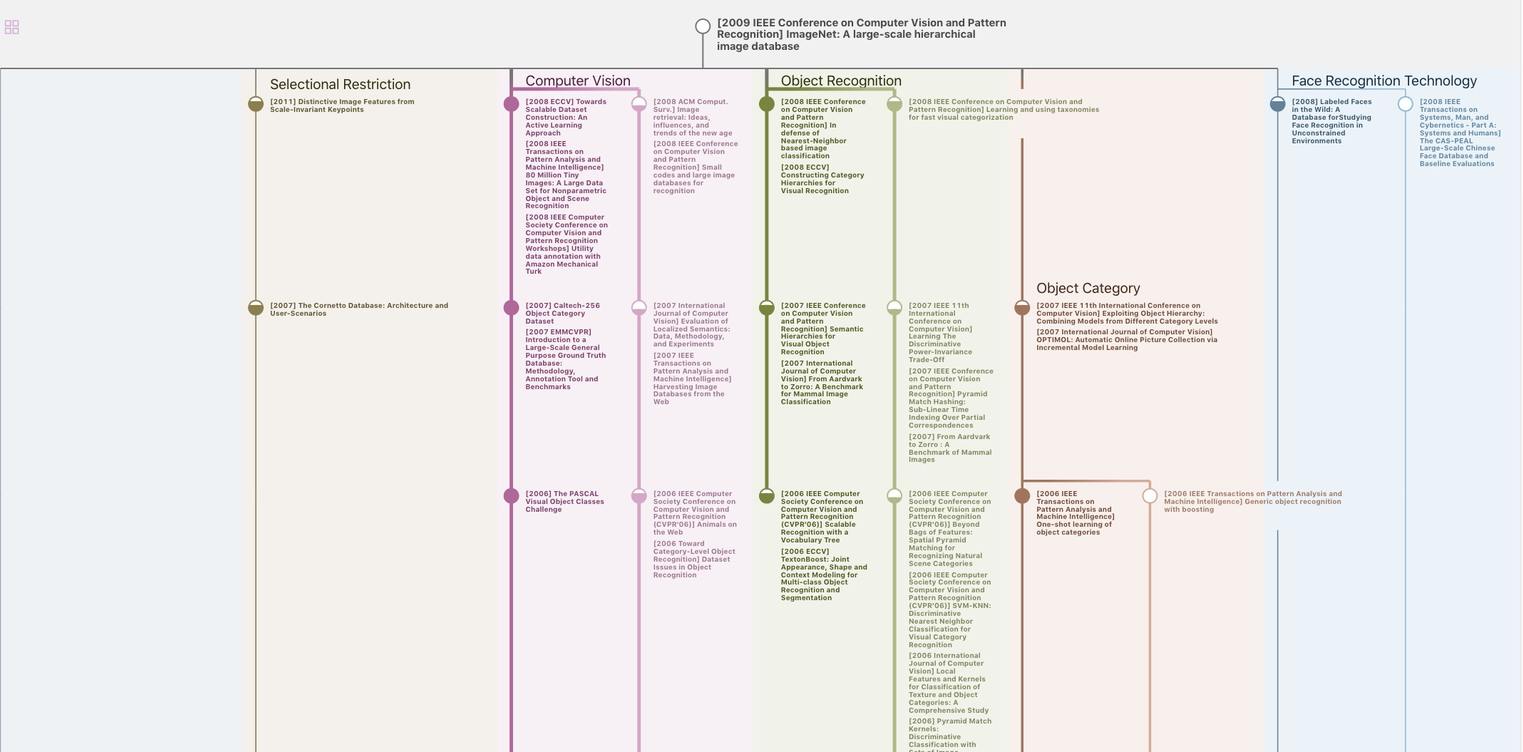
生成溯源树,研究论文发展脉络
Chat Paper
正在生成论文摘要