Traffic Speed Prediction with Convolutional Neural Network Adapted for Non-linear Spatio-temporal Dynamics.
BigSpatial@SIGSPATIAL(2018)
摘要
We develop an effective approach for non-linear and non-periodic traffic speed prediction on urban road networks, featuring convolutional neural networks enhanced by feature selections based on traffic flow dynamics. The prediction models we construct use convolutional neural network for the recognition of the spatio-temporal patterns of potentially congested (thus highly fluctuating) urban traffic. More importantly, a specially designed cone-shaped binary mask in the space-time domain is used to select relevant input features, both for trainings and for predictions. The design of the mask is based on the domain knowledge of the state-of-the-art traffic theories, which characterise the universal features on how perturbations to the road traffic propagate in space-time, based on the non-linear vehicle-to-vehicle interactions resulting from individual human driver responses to the environment. We test our models with empirical sensory data collected from highways in the city state of Singapore, with the aim of predicting traffic speed during peak hours. In addition to an average improvement of prediction accuracy from 0.9% to 3.3% for highly fluctuating road segments given different prediction horizons from 5 minutes to 20 minutes, we can achieve up to 23.8% improvement in accuracy for individual road segment, compared with the best results for applying state-of-art models. Results demonstrate that the proposed convolutional neural networks with masks can capture the non-linear spatio-temporal traffic dynamics and also provide accurate short-term traffic speed prediction at time with sudden changes of traffic conditions.
更多查看译文
关键词
Short-term Traffic Prediction,CNN,Deep Learning,Traffic Dynamics
AI 理解论文
溯源树
样例
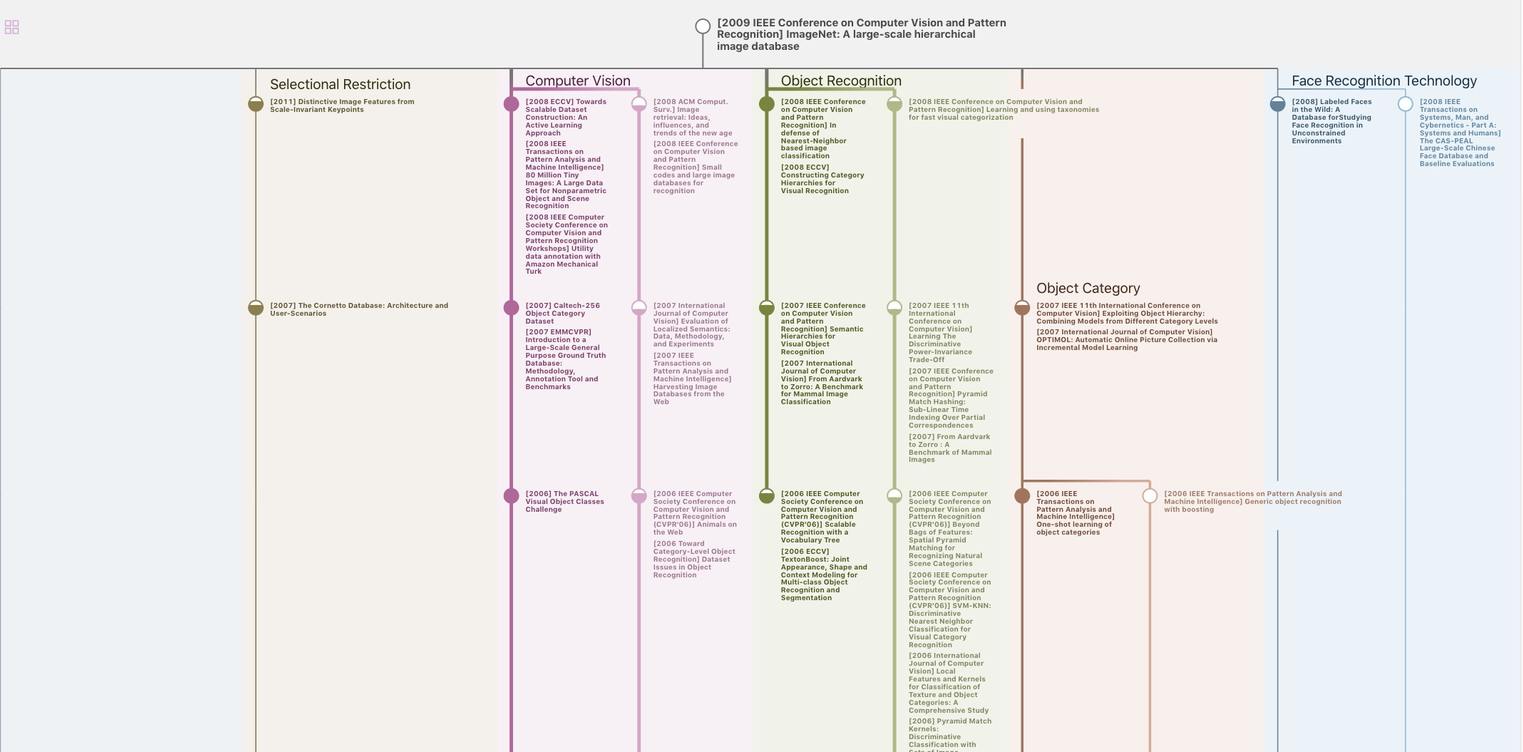
生成溯源树,研究论文发展脉络
Chat Paper
正在生成论文摘要