Neural Networks for Compressing and Classifying Speaker-Independent Paralinguistic Signals.
BigComp(2019)
摘要
Recognizing and classifying paralinguistic signals, with its various applications, is an important problem. In general, this task is considered challenging because the sound information from the signals is difficult to distinguish even by humans. Thus, analyzing signals with machine learning techniques is a reasonable approach to understanding signals. Audio features extracted from paralinguistic signals usually consist of high-dimensional vectors such as prosody, energy, cepstrum, and other speech-related information. Therefore, when the size of a training corpus is not sufficiently large, it is extremely difficult to apply machine learning methods to analyze these signals due to their high feature dimensions. This paper addresses these limitations by using neural networks' feature learning abilities. First, we use a neural network-based autoencoder to compress the signal to eliminate redundancy within the signal feature, and we show that the compressed signal features are competitive in distinguishing the signal compared to the original features. Second, we show by experiment that the neural network-based classification model almost always outperforms nonneural methods such as logistic regression, support vector machines, decision trees, and boosted trees.
更多查看译文
关键词
Task analysis,Training,Feature extraction,Principal component analysis,Support vector machines,Hidden Markov models,Neural networks
AI 理解论文
溯源树
样例
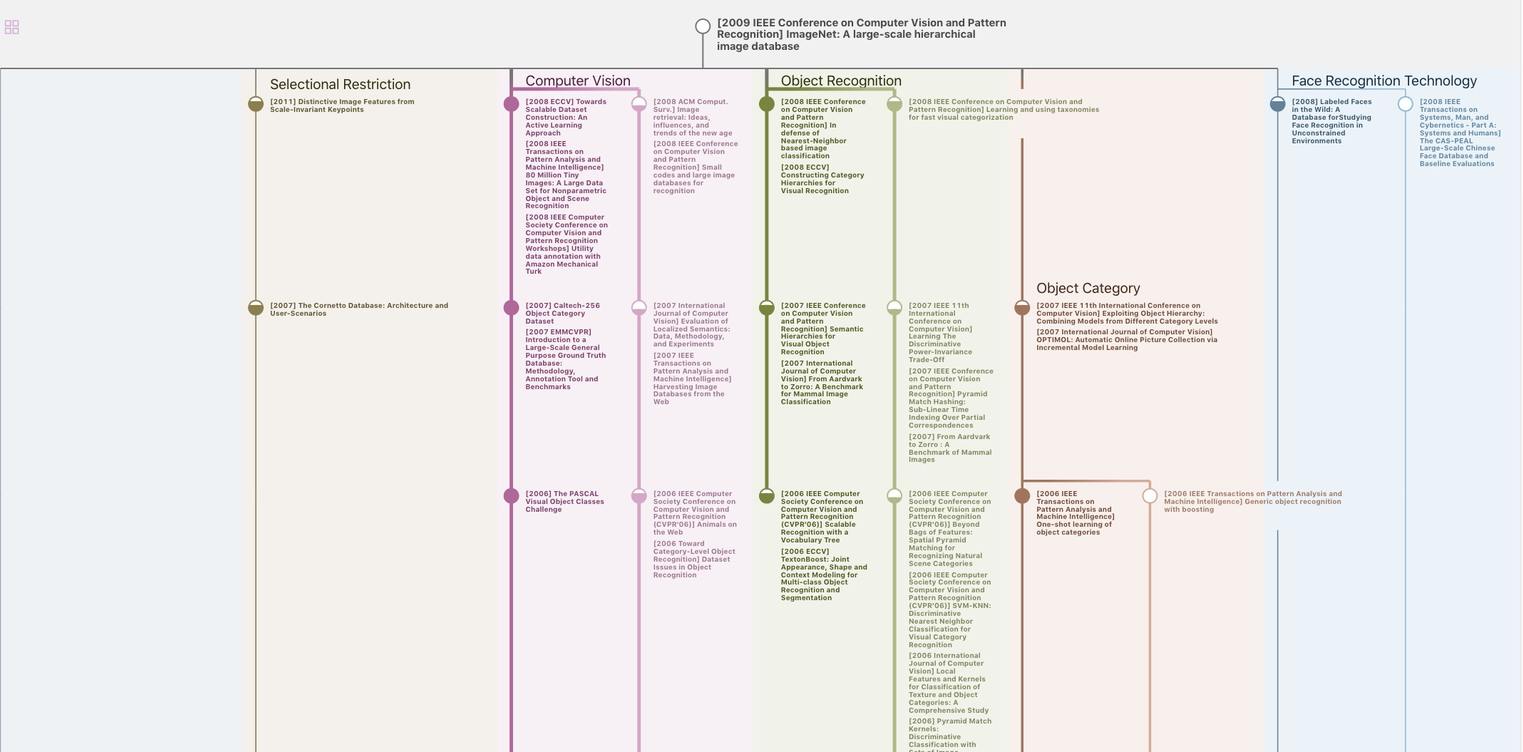
生成溯源树,研究论文发展脉络
Chat Paper
正在生成论文摘要