Actor-Critic Instance Segmentation
2019 IEEE/CVF CONFERENCE ON COMPUTER VISION AND PATTERN RECOGNITION (CVPR 2019)(2019)
摘要
Most approaches to visual scene analysis have emphasised parallel processing of the image elements. However one area in which the sequential nature of vision is apparent, is that of segmenting multiple, potentially similar and partially occluded objects in a scene. In this work, we revisit the recurrent formulation of this challenging problem in the context of reinforcement learning. Motivated by the limitations of the global max-matching assignment of the ground-truth segments to the recurrent states, we develop an actor-critic approach in which the actor recurrently predicts one instance mask at a time and utilises the gradient from a concurrently trained critic network. We formulate the state, action, and the reward such as to let the critic model long-term effects of the current prediction and incorporate this information into the gradient signal. Furthermore, to enable effective exploration in the inherently high-dimensional action space of instance masks, we learn a compact representation using a conditional variational auto-encoder We show that our actor-critic model consistently provides accuracy benefits over the recurrent baseline on standard instance segmentation benchmarks.
更多查看译文
关键词
Scene Analysis and Understanding,Segmentation,Grouping and Shape
AI 理解论文
溯源树
样例
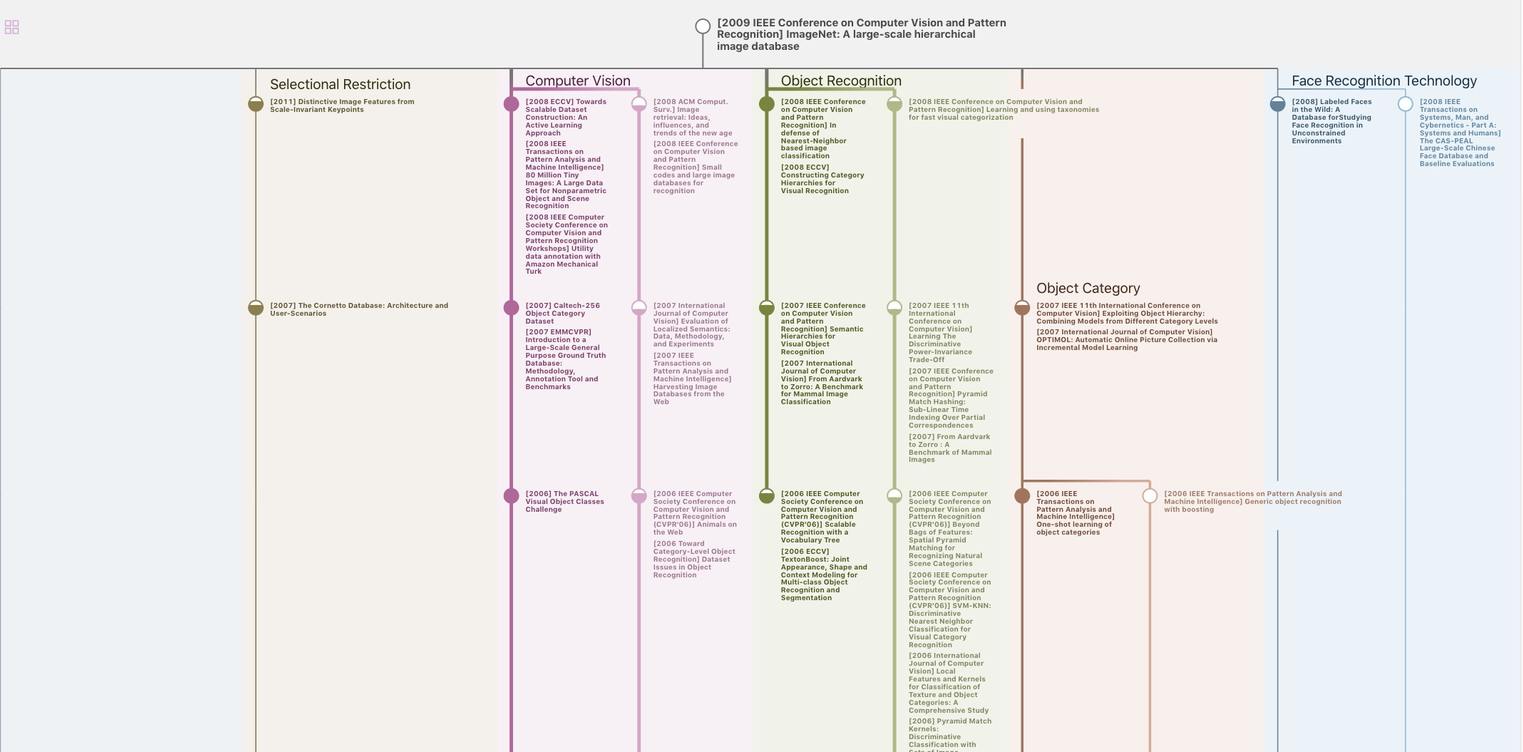
生成溯源树,研究论文发展脉络
Chat Paper
正在生成论文摘要