Application Of Deep Learning In Analog Circuit Sizing
PROCEEDINGS OF 2018 THE 2ND INTERNATIONAL CONFERENCE ON COMPUTER SCIENCE AND ARTIFICIAL INTELLIGENCE (CSAI 2018) / 2018 THE 10TH INTERNATIONAL CONFERENCE ON INFORMATION AND MULTIMEDIA TECHNOLOGY (ICIMT 2018)(2018)
摘要
Operational amplifiers (opamp) are the most common structures in analog circuit. However, opamp sizing problems rely heavily on the designer's personal experience. One problem that hampers opamp's automatic sizing is that the search space for the opamp size is too large, making the search process time consuming. In recent years, with the rapid development of GPU-based deep learning technology, it has become possible to automatically explore the sizing problem of analog circuits through the machine; that is, given the performance index, the machine is required to automatically output the length and width of each transistor and the output circuit performance should be close to the input performance specifications. In this paper, we use two neural network structures, Recurrent Neural Network (RNN) and Deep Neural Network (DNN), to construct two deep learning models that can automatically solve the sizing problem. The final simulation results show that our model can accurately predict the size of the transistor, where the accuracy of the RNN model is 92.6%, and the accuracy of the DNN is 95.6%.
更多查看译文
关键词
Analog integrated circuit (IC), design automation, deep learning, deep neural network (DNN), OpAmp design, recurrent neural network (RNN)
AI 理解论文
溯源树
样例
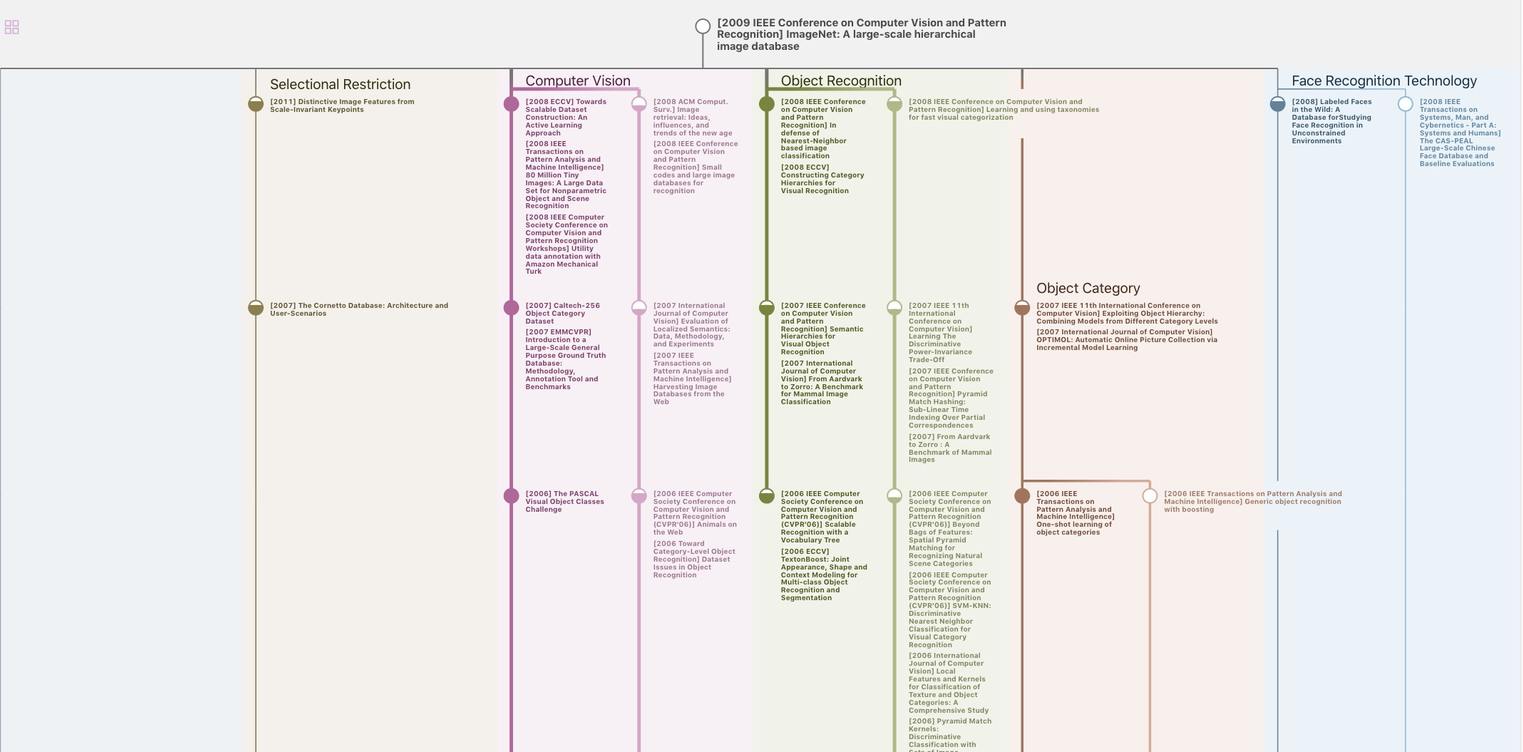
生成溯源树,研究论文发展脉络
Chat Paper
正在生成论文摘要