A Novel Algebraic Multigrid Approach Based on Adaptive Smoothing and Prolongation for Ill-Conditioned Systems.
SIAM JOURNAL ON SCIENTIFIC COMPUTING(2019)
摘要
The numerical simulation of modern engineering problems can easily incorporate millions or even billions of unknowns. In several applications, sparse linear systems with symmetric positive definite matrices need to be solved, and algebraic multigrid (AMG) methods represent common choices for the role of iterative solvers or preconditioners. The reason for their popularity relies on the fast convergence that these methods provide even in the solution of large size problems, which is a consequence of the AMG ability to reduce particular error components across their multilevel hierarchy. Despite carrying the name "algebraic," most of these methods still make assumptions on additional information other than the global assembled matrix, such as the knowledge of the operator's near kernel, which limits their applicability as black-box solvers. In this work, we introduce a novel AMG approach featuring the adaptive factored sparse approximate inverse (aFSAI) method as a flexible smoother, as well as three new approaches to adaptively compute the prolongation operator. We assess the performance of the proposed AMG through the solution of a set of model problems along with real-world engineering test cases. Moreover, we perform comparisons to other methods such as the aFSAI and BoomerAMG preconditioners, showing that our new method proves to be superior to the first strategy and comparable to the second one, if not better, as in the solution of linear elasticity models.
更多查看译文
关键词
adaptive algebraic multigrid,approximate inverses,preconditioning,AMG,parallel computing,adaptive prolongation,iterative methods
AI 理解论文
溯源树
样例
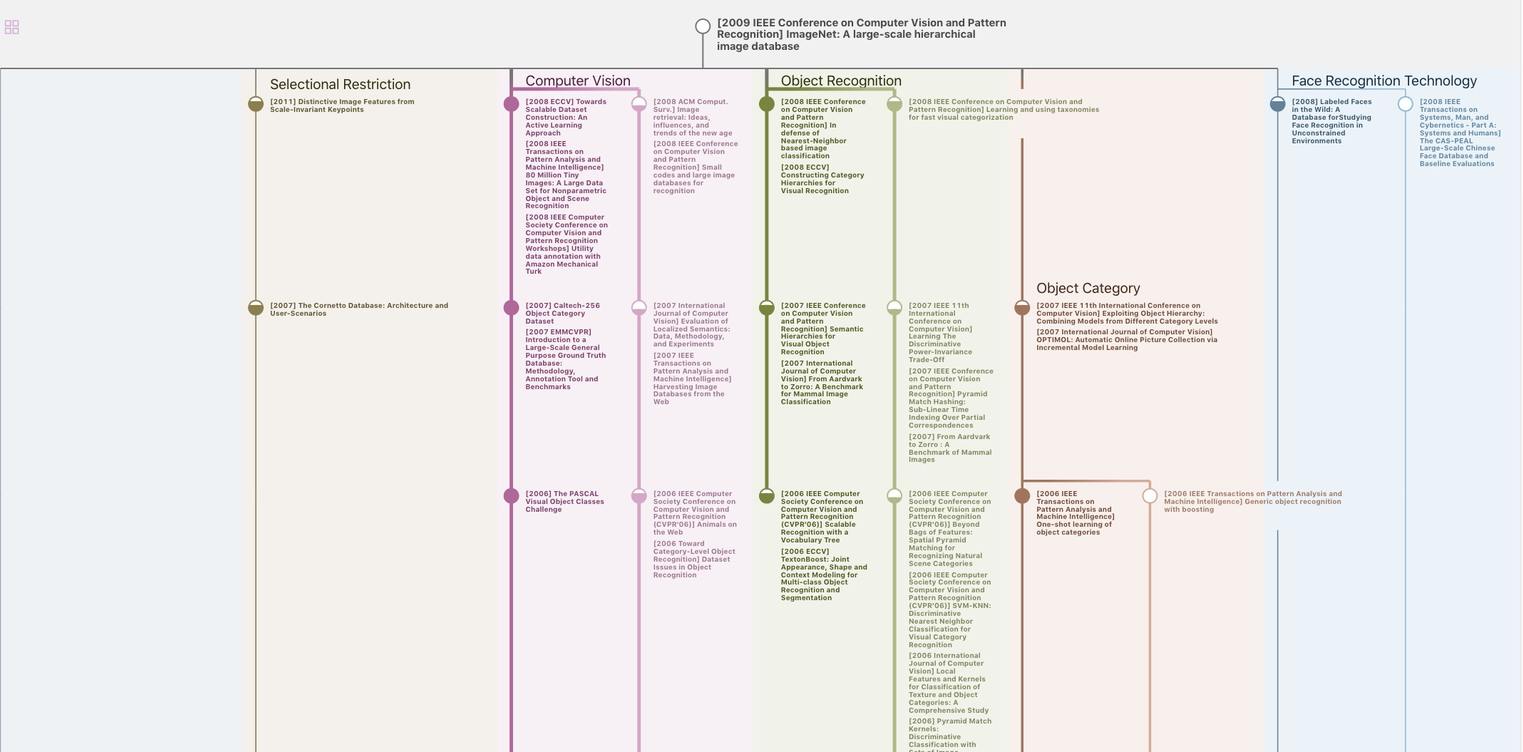
生成溯源树,研究论文发展脉络
Chat Paper
正在生成论文摘要