A Comparison of Policy Search in Joint Space and Cartesian Space for Refinement of Skills
ADVANCES IN SERVICE AND INDUSTRIAL ROBOTICS(2020)
摘要
Imitation learning is a way to teach robots skills that are demonstrated by humans. Transfering skills between these different kinematic structures seems to be straightforward in Cartesian space. Because of the correspondence problem, however, the result will most likely not be identical. This is why refinement is required, for example, by policy search. Policy search in Cartesian space is prone to reachability problems when using conventional inverse kinematic solvers. We propose a configurable approximate inverse kinematic solver and show that it can accelerate the refinement process considerably. We also compare empirically refinement in Cartesian space and refinement in joint space.
更多查看译文
关键词
Learning from demonstration,Imitation learning,Reinforcement learning,Policy search,Inverse kinematics
AI 理解论文
溯源树
样例
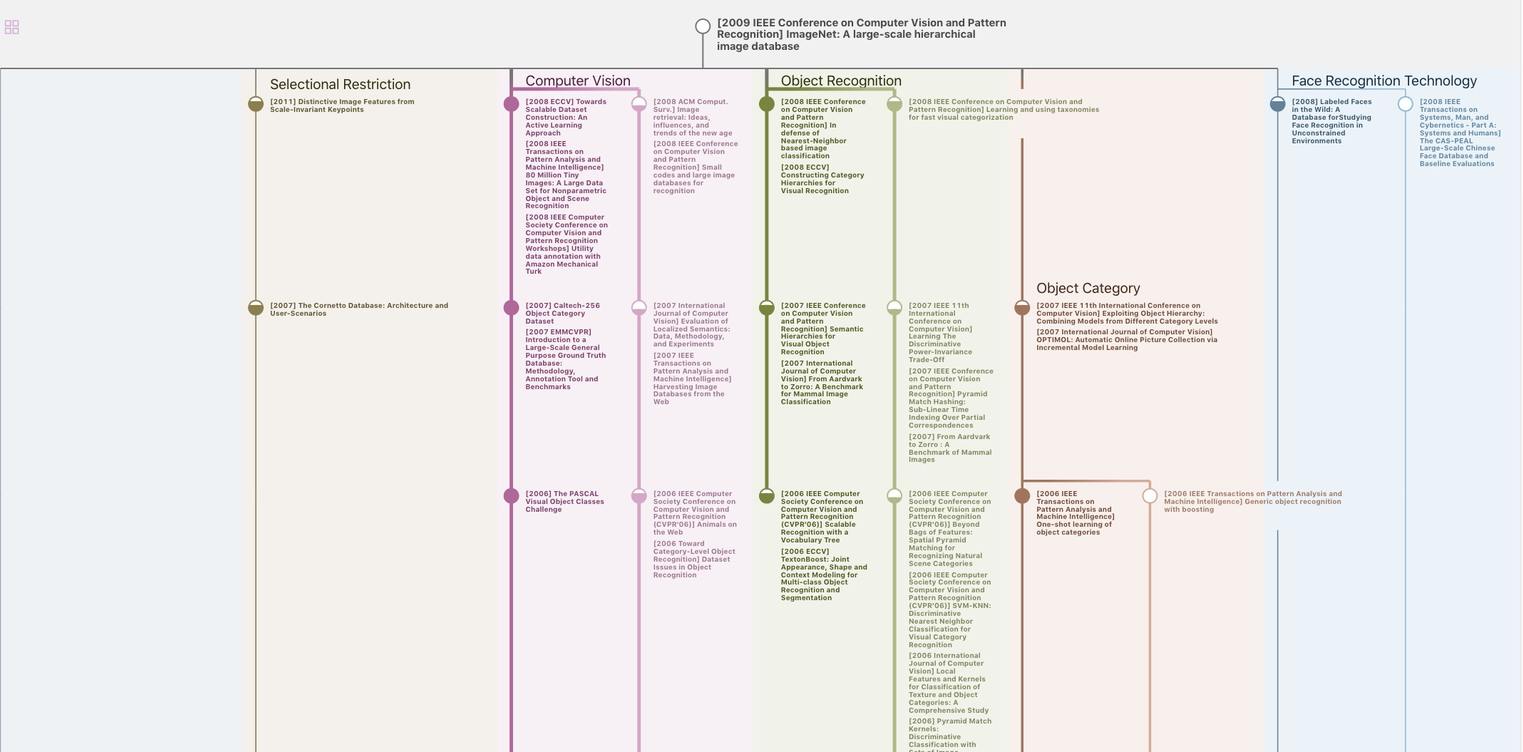
生成溯源树,研究论文发展脉络
Chat Paper
正在生成论文摘要