Data-Driven Deep Learning for Automatic Modulation Recognition in Cognitive Radios
IEEE Transactions on Vehicular Technology(2019)
摘要
Automatic modulation recognition (AMR) is an essential and challenging topic in the development of the cognitive radio (CR), and it is a cornerstone of CR adaptive modulation and demodulation capabilities to sense and learn environments and make corresponding adjustments. AMR is essentially a classification problem, and deep learning achieves outstanding performances in various classification tasks. So, this paper proposes a deep learning-based method, combined with two convolutional neural networks (CNNs) trained on different datasets, to achieve higher accuracy AMR. A CNN is trained on samples composed of in-phase and quadrature component signals, otherwise known as in-phase and quadrature samples, to distinguish modulation modes, that are relatively easy to identify. We adopt dropout instead of pooling operation to achieve higher recognition accuracy. A CNN based on constellation diagrams is also designed to recognize modulation modes that are difficult to distinguish in the former CNN, such as 16 quadratic-amplitude modulation (QAM) and 64 QAM, demonstrating the ability to classify QAM signals even in scenarios with a low signal-to-noise ratio.
更多查看译文
关键词
Constellation diagram,Feature extraction,Task analysis,Training,Deep learning,Signal to noise ratio
AI 理解论文
溯源树
样例
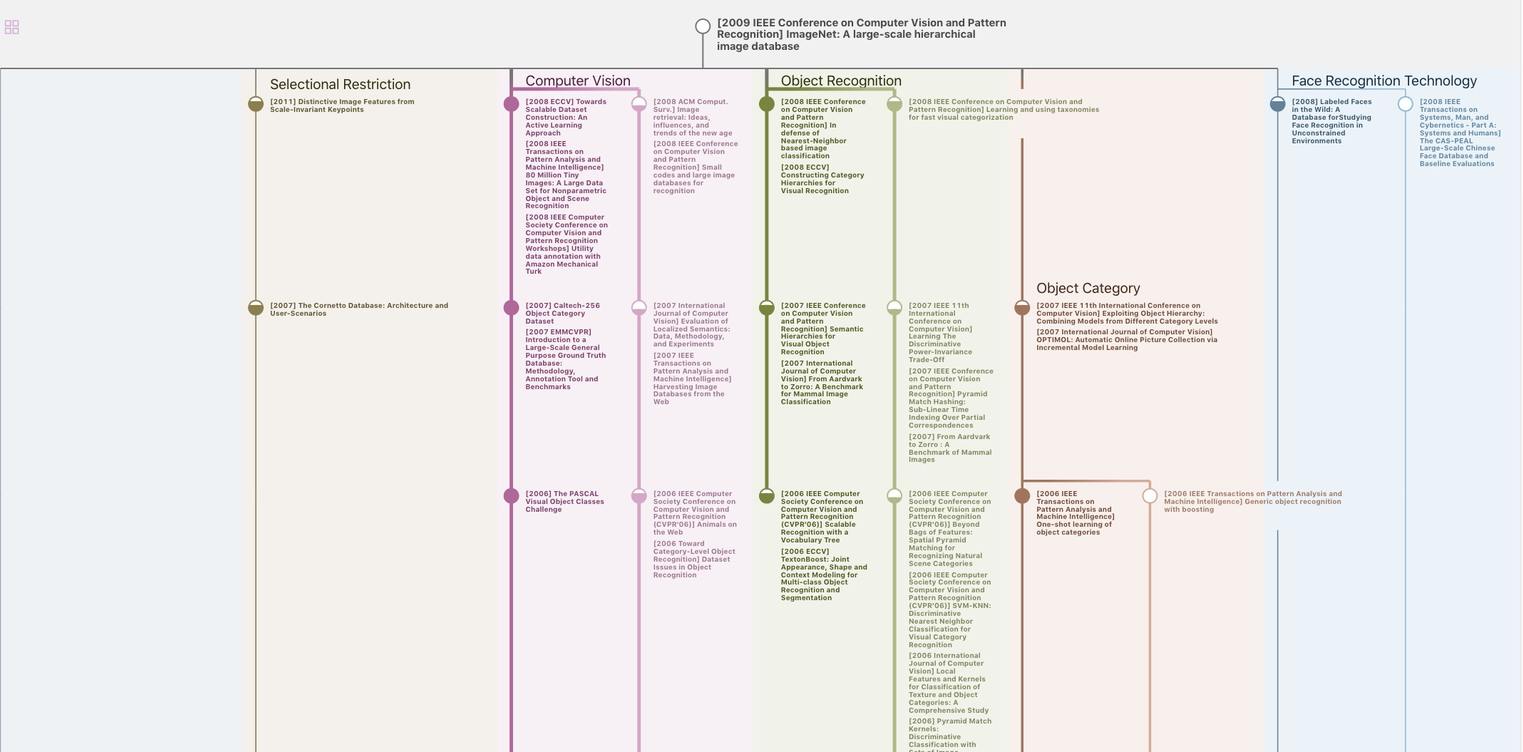
生成溯源树,研究论文发展脉络
Chat Paper
正在生成论文摘要