Enhancing Music Features by Knowledge Transfer from User-item Log Data
ICASSP 2019 - 2019 IEEE International Conference on Acoustics, Speech and Signal Processing (ICASSP)(2019)
摘要
In this paper, we propose a novel method that exploits music listening log data for general-purpose music feature extraction. Despite the wealth of information available in the log data of user-item interactions, it has been mostly used for collaborative filtering to find similar items or users and was not fully investigated for content-based music applications. We resolve this problem by extending intra-domain knowledge distillation to cross-domain: i.e., by transferring knowledge obtained from the user-item domain to the music content domain. The proposed system first trains the model that estimates log information from the audio contents; then it uses the model to improve other task-specific models. The experiments on various music classification and regression tasks show that the proposed method successfully improves the performances of the task-specific models.
更多查看译文
关键词
Task analysis,Training,Data models,Knowledge transfer,Feature extraction,Recommender systems,Matrix converters
AI 理解论文
溯源树
样例
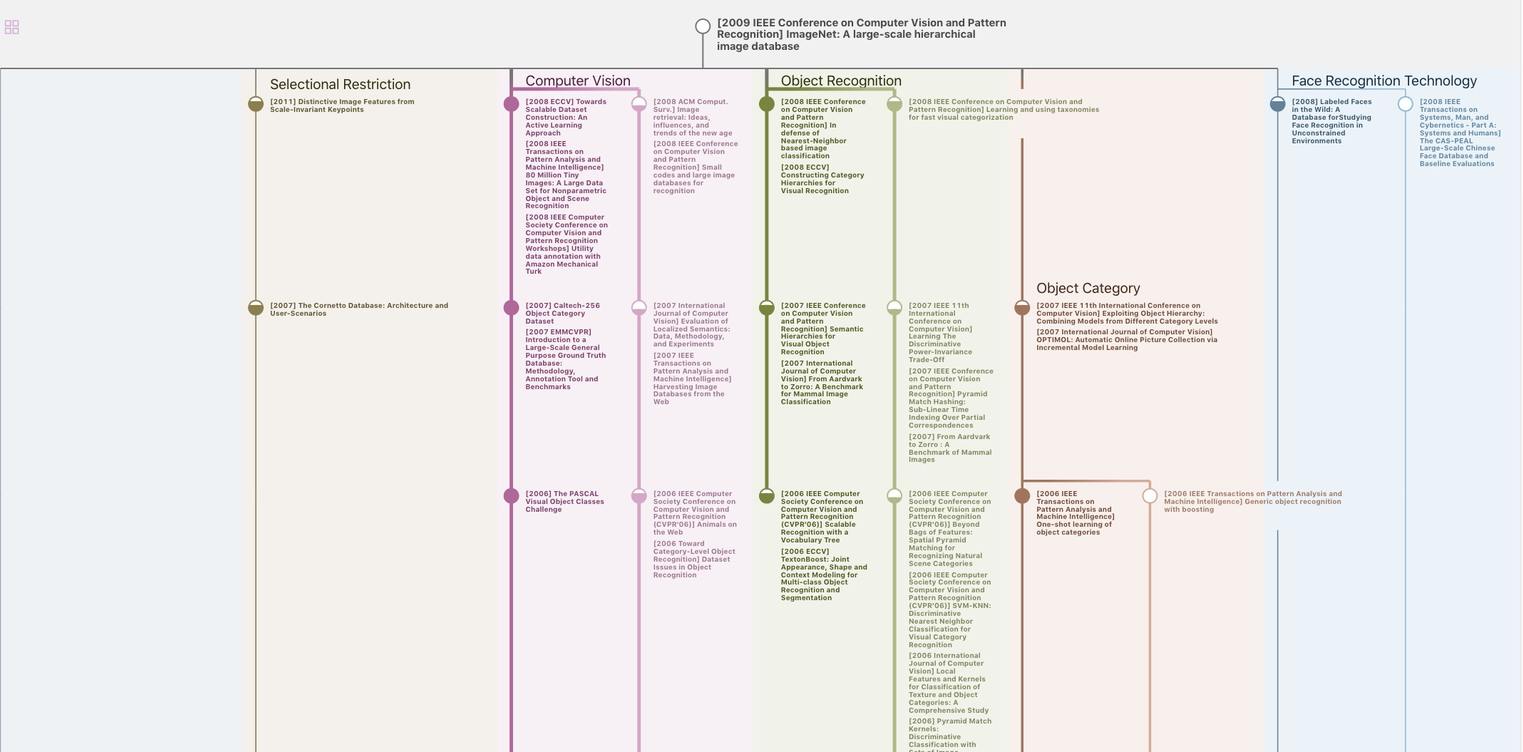
生成溯源树,研究论文发展脉络
Chat Paper
正在生成论文摘要