Machine Learning For Segmenting Cells In Corneal Endothelium Images
MEDICAL IMAGING 2019: COMPUTER-AIDED DIAGNOSIS(2019)
摘要
Images of the endothelial cell layer of the cornea can be used to evaluate corneal health. Quantitative biomarkers extracted from these images such as cell density, coefficient of variation of cell area, and cell hexagonality are commonly used to evaluate the status of the endothelium. Currently, fully-automated endothelial image analysis systems in use often give inaccurate results, while semi-automated methods, requiring trained image analysis readers to identify cells manually, are both challenging and time-consuming. We are investigating two deep learning methods to automatically segment cells in such images. We compare the performance of two deep neural networks, namely U-Net and SegNet. To train and test the classifiers, a dataset of 130 images was collected, with expert reader annotated cell borders in each image. We applied standard training and testing techniques to evaluate pixel-wise segmentation performance, and report corresponding metrics such as the Dice and Jaccard coefficients. Visual evaluation of results showed that most pixel-wise errors in the U-Net were rather non-consequential. Results from the U-Net approach are being applied to create endothelial cell segmentations and quantify important morphological measurements for evaluating cornea health.
更多查看译文
关键词
deep learning,endothelial cell segmentation,cornea
AI 理解论文
溯源树
样例
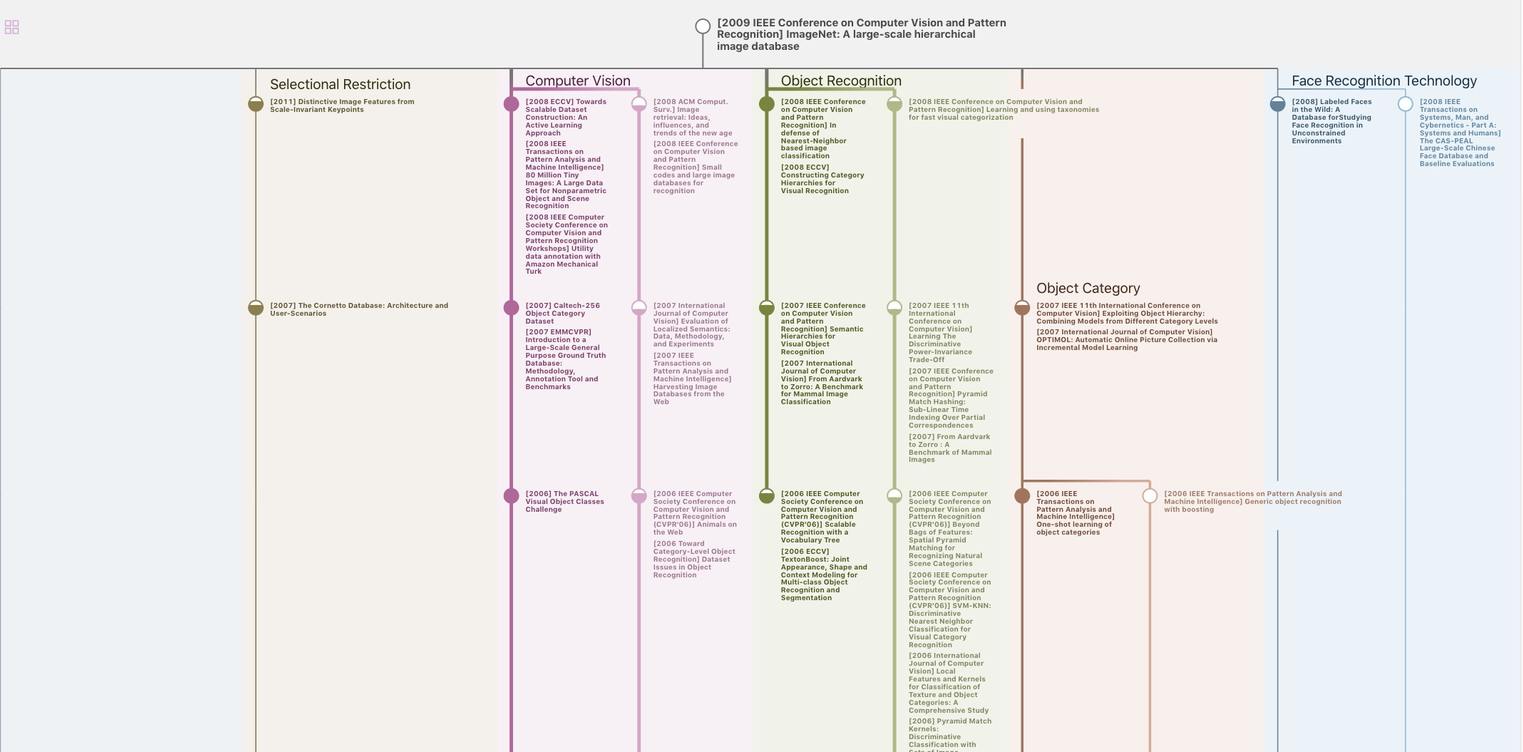
生成溯源树,研究论文发展脉络
Chat Paper
正在生成论文摘要