Differentiation of polyps by clinical colonoscopy via integrated color information, image derivatives and machine learning.
Proceedings of SPIE(2019)
摘要
Clinical colonoscopy is currently the gold standard for polyp detection and resection. Both white light images (WLI) and narrow band images (NBI) could be obtained by the fibro-colonoscope from the same patient and currently used as a diagnosing reference for differentiation of hyperplastic polyps from adenomas. In this paper, we investigate the performance of WLI and NBI in different color spaces for polyp classification. A Haralick model with 30 co-occurrence matrix features is used in our experiments on 74 polyps, including 19 hyperplastic polyps and 55 adenomatous ones. The features are extracted from different color channels in each of three color spaces (RGB, HSV, chromaticity) and different derivative (intensity, gradient and curvature) images. The features from each derivative image in each color space are classified. The classification results from all the color spaces and all the derive images are input to a greedy machine learning program to verify the necessity of the integration of derivative image data and different color spaces. The feature classification and machine learning are implemented by the use of the Random Forest package. The well-known area under the receiver operating characteristics curve is calculated to quantify the performances. The experiments validated the advantage of using the integration of the three derivatives of WLI and NBI and the three different color spaces for polyp classification.
更多查看译文
关键词
Colonoscopy,WLI,NBI,Haralick features,image derivatives,color spaces,polyp classification
AI 理解论文
溯源树
样例
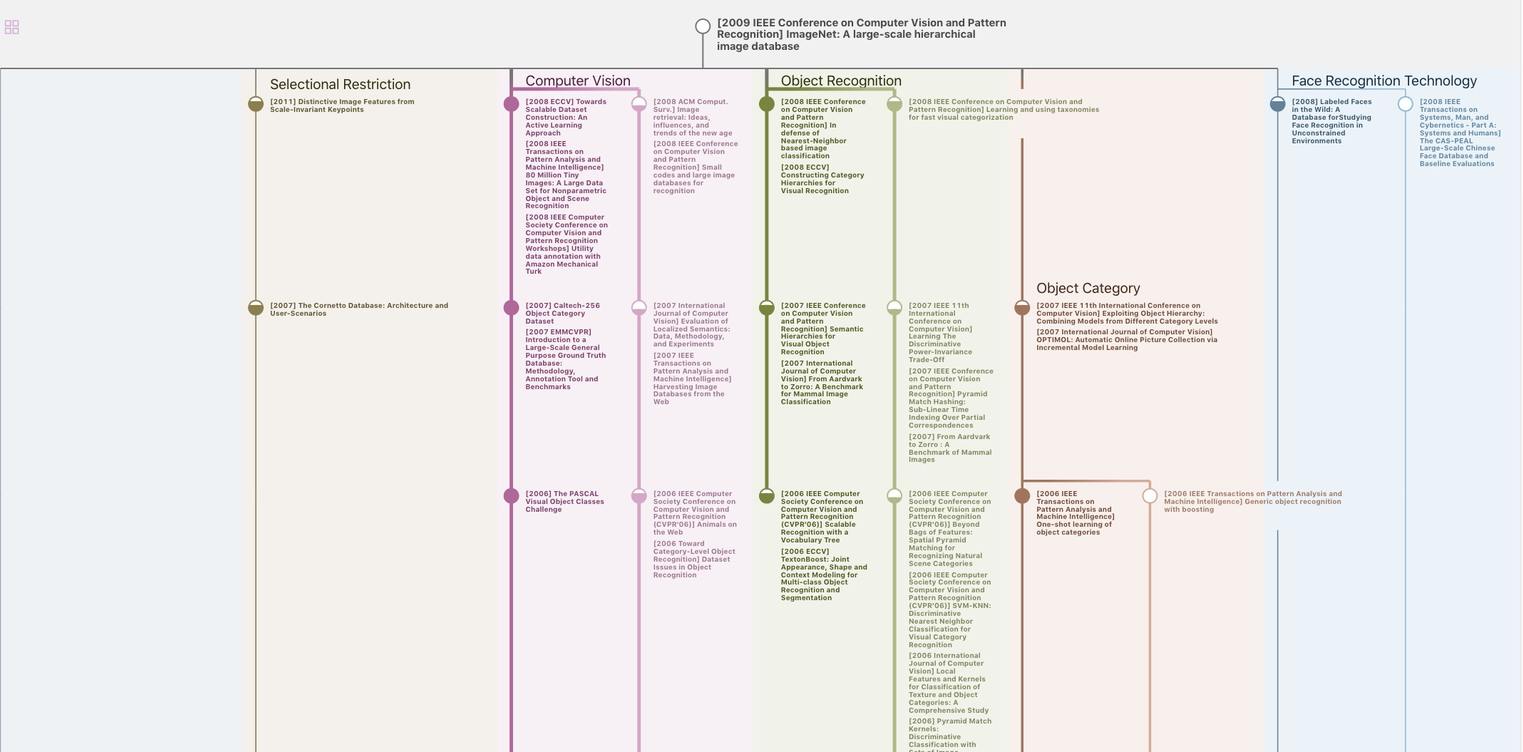
生成溯源树,研究论文发展脉络
Chat Paper
正在生成论文摘要